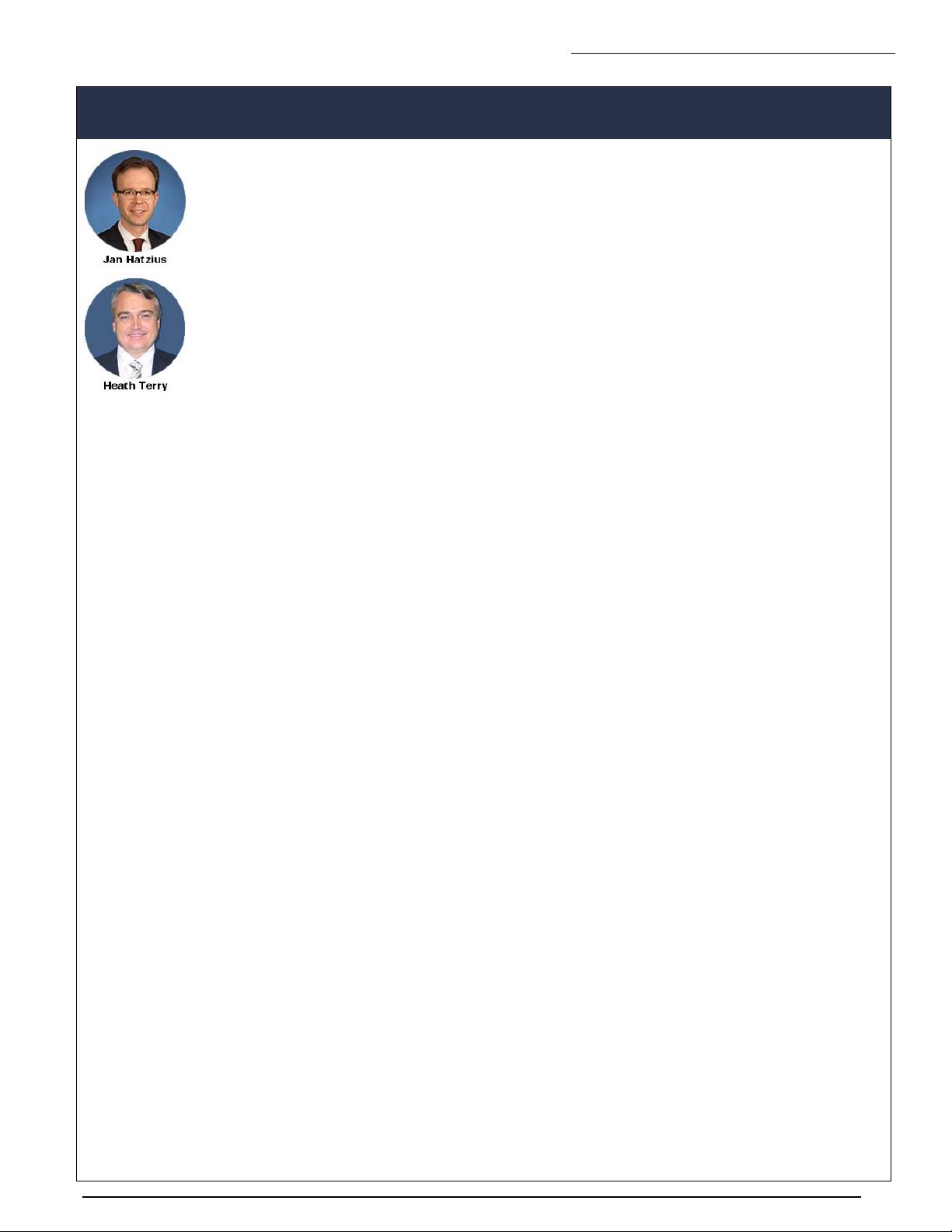
November 14, 2016 Profiles in Innovation
Goldman Sachs Global Investment Research 18
GS Research Internet analyst Heath Terry sat
down with Chief Economist Jan Hatzius to
discuss the role AI & machine learning could
play in boosting lagging labor productivity.
Heath Terry: W
hat has led to the lack of
measurable productivity growth over the
last decade?
Jan Hatzius:
A good starting point is the
1990s, where we did have a sizeable
measured productivity acceleration which
was mainly technology driven. The
technology sector had gotten bigger and
measured output growth in the technology
sector was very rapid, and that was enough to produce an overall
acceleration in the economy. Recently, over the last 10 years or so,
we’ve seen a renewed deceleration to productivity growth rates
that are as low as the 1970s and 1980s, and potentially even lower.
I think there is more than one driver, but I would say there are three
things that have lowered productivity growth in my view. One is a
bit of a cyclical effect. We still have some hangover from the great
recession with a relatively slow pace of capital accumulation,
relatively low levels of investment, and relatively rapid employment
growth. Since productivity is measured as output per hour worked,
that somewhat perversely means that you can have lower
productivity numbers when the labor market is improving rapidly.
Another factor may be some slowdown in the overall pace of
technological progress. It’s reasonable to believe that perhaps the
1990s, with the introduction of the internet, was a relatively rapid
period for technological progress, and I would say there is some
support for the idea that it is a little slower now.
The third point is that technological progress that has occurred
over the last decade, like mobile and consumer focused
technology, is something that statisticians are not very well set up
to capture in the numbers. The reason is that quality improvement
in a lot of the new technologies that have dominated in the last
decade or so is very difficult to capture in a quantitative sense.
Statisticians are not building in significant quality improvement
into a lot of the areas that have been at the cutting edge.
So I would point to three things. There are cyclical effects,
probably some slowdown in technological progress, and very
likely an increase in the measurement error in the productivity
statistics.
Terry: Back to the productivity boom in the 1990s, what role did
technology play?
Hatzius:
What drove it was mainly general purpose technologies
like semiconductors and computers, which had become much
larger as a share of the economy than they were in the 1970s or
1980s and where technological progress was very rapid, in ways
that statisticians were well set up to measure. The statisticians in
the 1990s had made a lot of effort to capture quality improvement
faster; processer speeds, more memory, better attributes in
computer hardware, which led to large increases in the measured
contribution of the technology sector. The technology sector was
very central to pick up in the productivity numbers from the 1990s
lasting to the early and mid-2000s.
Terry:
We’ve seen a lot of technology development over the last
10-15 years. Why hasn’t there been a similar impact to
productivity from technologies such as the iPhone, Facebook, and
the development of cloud computing?
Hatzius:
We don’t have a full answer to it, but I do think an
important part of the answer is the statistical ability to measure
improvement in quality, and the impact of new products in the
economic statistics is limited. It’s relatively easy to measure
nominal GDP, that’s basically a matter of adding up receipts. There
is room for measurement error as there is in almost everything,
but I don’t have real first order concern that measurement is
getting worse in terms of measuring nominal GDP. Converting
nominal GDP numbers into real GDP numbers by deflating it with a
quality adjusted overall price index is where I think things get very
difficult. If you look, for example, at the way software sectors enter
the official numbers, if you believe the official numbers, $1000 of
outlay on software now buys you just as much real software as
$1000 of outlay bought you in the 1990s. There has been no
improvement in what you get for your money in the software
sector. That’s just very difficult to believe. That just does not pass
the smell test. Because of the difficulty of capturing these quality
improvements, the fact that the technology sector has increasingly
moved from general purpose hardware to specialized hardware,
software and digital products has led to increased understatement
and mismeasurement.
Terry:
What kind of impact could the development of technologies
like artificial intelligence and machine learning have on
productivity?
Hatzius: In principle, it does seem like something that could be
potentially captured better in the statistics than the last wave of
innovation to the extent that artificial intelligence reduces costs
and reduces the need for labor input into high value added types of
production. Those cost saving innovations in the business sector
are things statisticians are probably better set up to capture than
increases in variety and availability of apps for the iPhone, for
example. To the extent Artificial Intelligence has a broad based
impact on cost structures in the business sector, I’m reasonably
confident that it would be picked up by statisticians and would
show up in the overall productivity numbers.
I would just add one general caveat, which is that the U.S.
economy is very large. Even things that are important in one sector
of the economy and seem like an overwhelming force often look
less important when you divide by $18tn, which is the level of U.S.
nominal GDP, so the contribution in percentage terms may not
look as large as one might think from a bottom up perspective. But
in principle, this is something that could have a measurable
impact.
Terry: You touched on the impact to cost, how do you see
something like that impacting pricing? Does that become a
contributor to the broader deflationary force that we’ve seen in
certain parts of the economy?
Hatzius: I certainly think that in terms of productivity gains, the first
order impact is often to lower costs and lower prices. Keeping
everything else constant would mean lower overall inflation in the
economy. It’s often not the right assumption that you want to keep
AI & The Productivity Paradox: An interview with Jan Hatzius
起点学院www.qidianla.com,互联网黄埔军校,打造最专业最系统的产品、运营、交互课程。