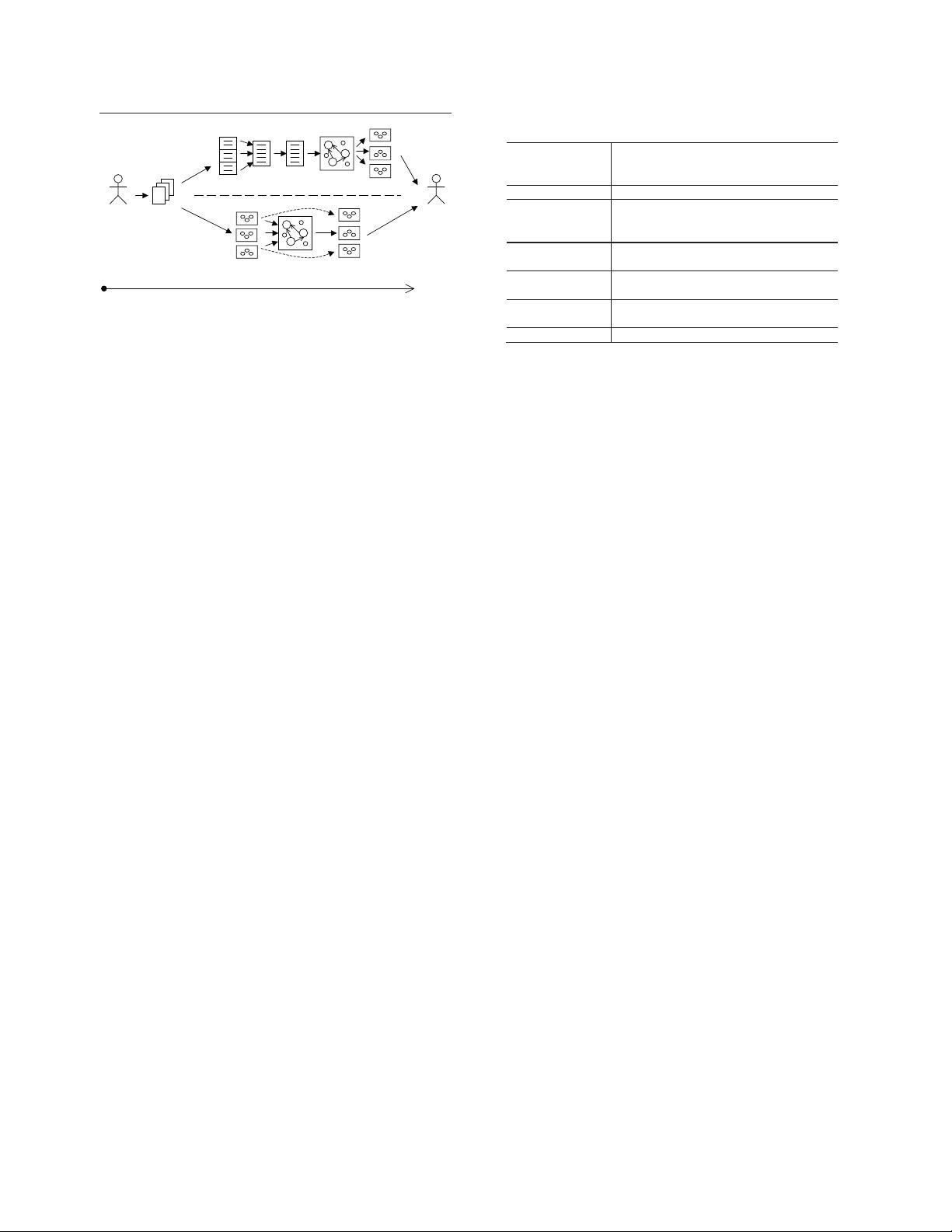
G team
V team
Stakeholders Transcripts
Viewpoints
Models
Merged Model
List of
Concepts
Merged
List
Global
Model
Model Slices
Raw data Interpreted information
Allocated
List
Model Slices
Meeting with
stakeholders
Fig. 1. Original study’s design (adopted from [11]).
a single coherent model” [11]. “Better” was translated to
“a richer domain understanding” and further operationalized
by 3 response variables: “hidden assumptions”, “disagree-
ments between stakeholders”, and “new requirements”. To
test the predicted differences, one team followed a global
(G) approach, whereas the other team adopted viewpoints
(V), to build i
∗
models [27] for the Kids Help Phone (KHP)
organization. The fundamental distinction was model merging
that was explicit for the V team but nonexistent for the G
team. Fig. 1 shows the original study’s design. Table I helps
explain the key components of this design. We summarize the
original study’s main findings as follows.
• R
1
: Viewpoints led to a richer domain understanding.
While the benefits of viewpoints were observed, there
lacked detailed and quantitative analyses (especially those
of the 3 response variables) in [11].
• R
2
: Viewpoints-based modeling was slower. In fact, it
was so time-consuming that the V team was not able to
produce their merged i
∗
model. In Fig. 1, only the slices,
rather than the integrated whole, from both teams were
compared and presented to the KHP stakeholders.
• R
3
: Process was more important than product. This could
be seen as a combination of R
1
and R
2
. On one hand, the
process of merging stakeholder viewpoints did improve
the understanding of the problem domain [11]. On the
other hand, the merged product never existed, due to the
lack of modeling tool support for handling i
∗
syntax [11].
In summary, not only were the viewpoints theory and
hypothesis stated clearly in [11], but the results were thought-
provoking, including such startling claims that promoted a
requirements modeling process even though no end result was
produced. This stands in stark contrast to artifact-based RE
which values the requirements tangibles rather than the way
of creating them [6]. Nevertheless, Easterbrook et al.’s study
design was straightforward and sound. Their work [11] also
appeared to be influential, especially in meeting some emerg-
ing RE challenges [12]–[16]. For these reasons, we believe
Easterbrook et al.’s work [11] is a study worth replicating.
III. R
EPLICATION DESIGN AND EXECUTION
Our theoretical replication investigates the same central
hypothesis as the original study: “Modeling stakeholder view-
points separately and then combining them leads to a richer
TABLE I
O
RIGINAL STUDY’S DESIGN EXPLAINED
Study Context
Kids Help Phone (KHP), a non-profit social
organization that provides counseling to
kids and their parents across Canada
Study Period
around 2004
Organizational
Need Related to
the Study
KHP wanted to analyze the strategic
technology change of developing new
internet-based services
Modeling Input
transcripts from interviewing 14 KHP
stakeholders (approx. 140 pages in total)
V Team
viewpoint modeling team consisting of 3
graduate students
G Team
global modeling team consisting of 2
graduate students
Modeling Output
team-based i* models
understanding of the domain” [11]. Furthermore, we take the-
oretical replication’s advantage to improve the study procedure
in three aspects.
• Mitigate a threat. The original study collected purely
qualitative data, and relied on the subjective opinions of
the modelers to measure “a richer domain understanding”.
In contrast, we examine 3 finer measures — “hidden
assumptions”, “stakeholder disagreements”, and “new
requirements” — which were laid out but not analyzed
in [11]. In our replication, these 3 response variables
are assessed by the domain experts rather than by the
modelers themselves, reducing the experimenter bias.
• Take into account an evolving factor. Among the many
things changed from the original study, we intentionally
incorporate the i
∗
tool support in our replication. In [11],
both the G and V teams used Microsoft Visio for the
modeling. While the V team failed to build the merge,
both teams encountered difficulty with Visio in managing
large, evolving models. In the past decade, i
∗
tooling has
greatly increased. The community wiki, for example, lists
over 20 tools, many of which are open-source [28]. We
choose OpenOME [29] to update the study design and
describe this tool in more detail in Section III-B.
• Devise a new mechanism to evaluate i
∗
models. Unlike
the original study’s focus on the internal qualities of the
models, such as size and readability [11], we resort to
the domain expert by eliciting a set of questions from
the expert and then assessing how well the resulting i
∗
models are capable of answering those questions. We
refer to such an approach as an external way of evaluating
i
∗
models. Horkoff and Yu [30] recently presented an
external framework for interactive i
∗
model analysis,
which we discuss further, along with other goal model
evaluation approaches, in Section III-C.
A. Replication Context
We adopt case study [7] as the basis for our replication
design. The contemporary phenomenon of our investigation
is the Scholar@UC project [31]. Scholar@UC is a digital
repository that enables the University of Cincinnati (UC)
community to share its research and scholarly work with
a worldwide audience. Its mission includes preserving the
188