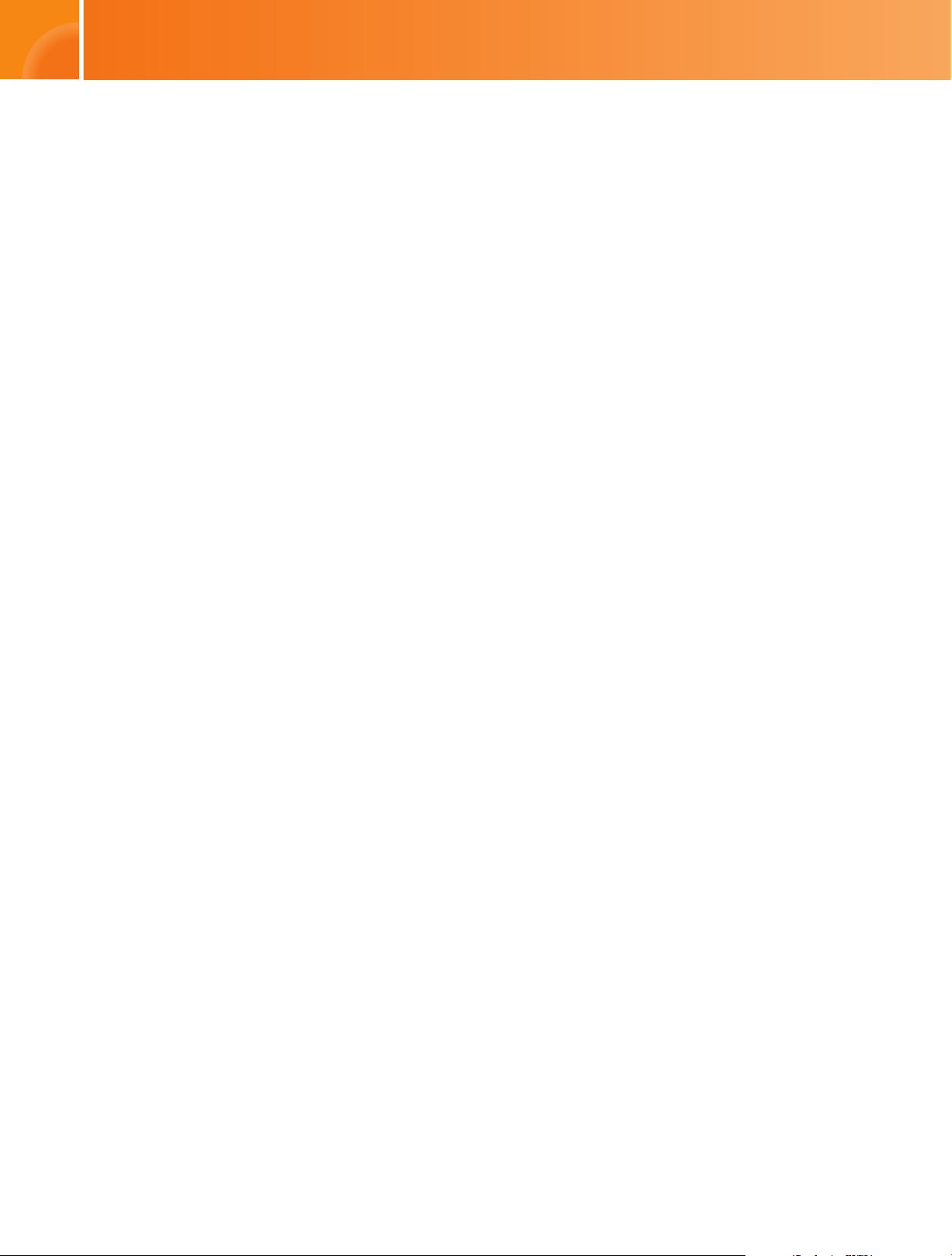
4 Journal of Communications and Information Networks
effective communication service for wireless users.
These data include the distribution of spectrum uti-
lization, spatial statistics of ultra-densely deployed
cells and resource allocation of transmission signals.
Finally, developing wireless big data means the data
set that are generated during the processes of testing
and evaluating the performance of unknown spec-
trum, novel transmission techniques, innovative ac-
cess and revolutionary network structure.
Here, we point out that the wireless big data can
also be categorized according to their specific areas,
which include cellular networks, Wi-Fi hotspots and
smartphones D2D, smart grids, wireless sensor net-
works, IoT, etc.
2.2 Data collection
Data collection is in some sense an engineering
oriented problem, which is mostly concerned with
telecommunication operators, although their pur-
poses are not for wireless big data research. However,
several research works have been recently released on
this topic.
As for the data gathering challenge in gather-
ing real-time big data in a complex indoor indus-
trial environment
[5]
, a RTBDG (Real-Time Big Data
Gathering) algorithm based on an indoor WSN is
proposed, where sensor nodes can screen the data
collected from the environment and equipment ac-
cording to the requirements of risk analysis, which
may be widely applied to risk analysis in different
industrial operations.
Another interesting point in this topic is based
on compressive sensing
[6]
. The authors attempted
to deal with the shortage of energy in wireless sen-
sor nodes, and proposed a compressive-sensing-based
collection framework to minimize the amount of col-
lection while maintaining data quality.
2.3 Data model
The random matrix theory model is applied to repre-
sent varying amounts of data collected from multiple
sources. In Ref. [7], a big data analytic unified data
model based on the random matrix theory and ma-
chine learning in mobile cellular networks is studied.
Several examples of data types have been presented
to clarify the performance of big data analytic based
on random matrix theory, such as big signaling data,
big traffic data, big location data, big radio wave-
forms data, and big heterogeneous data, in which the
high dimensionality of the spatial-temporal datasets
is exploited, and the interrelationship and unique
characteristics between big data and mobile cellu-
lar networks is addressed. Moreover, in Ref. [8], the
large-scale random matrices are introduced as build-
ing blocks to model the massive big data collected
by the massive MIMO (Multiple Input Multiple Out-
put) system, and forwarded to the base station for
processing and storage. This model is applied to
distributed spectrum sensing and network monitor-
ing. The software defined radio platform, equipped
with USRP (Universal Software Radio Peripheral),
is used to emulate the antenna in the base station
and demonstrate the data processing in the CPU.
Large-scale data and heterogeneous data may be
the unique characteristics of wireless big data sim-
ply as variety and veracity, respectively. Based on
these characteristics, various data types are pro-
posed, such as unstructured data, semistructured
data, and structured data. The authors in Ref. [9]
introduced an unified tensor model to represent the
data generated from multiple sources. Based on the
tensor extension operator, different data types are
represented in the forms of subtensors and processed
to unified tensors. Using the aforementioned model,
an incremental high order singular value decompo-
sition method is described for reducing the dimen-
sionality of the big data. Moreover, intelligent trans-
portation is used as a case study to verify the perfor-
mance of the data representation model and incre-
mental dimensionality reduction method and then it
can be seen that this model can be implemented as
the big data system model for the data representa-
tion.
The authors in Ref. [10] introduced a mobility an-
alytical framework for big mobile data, based on real
data traffic collected from 2G/3G/4G networks cov-