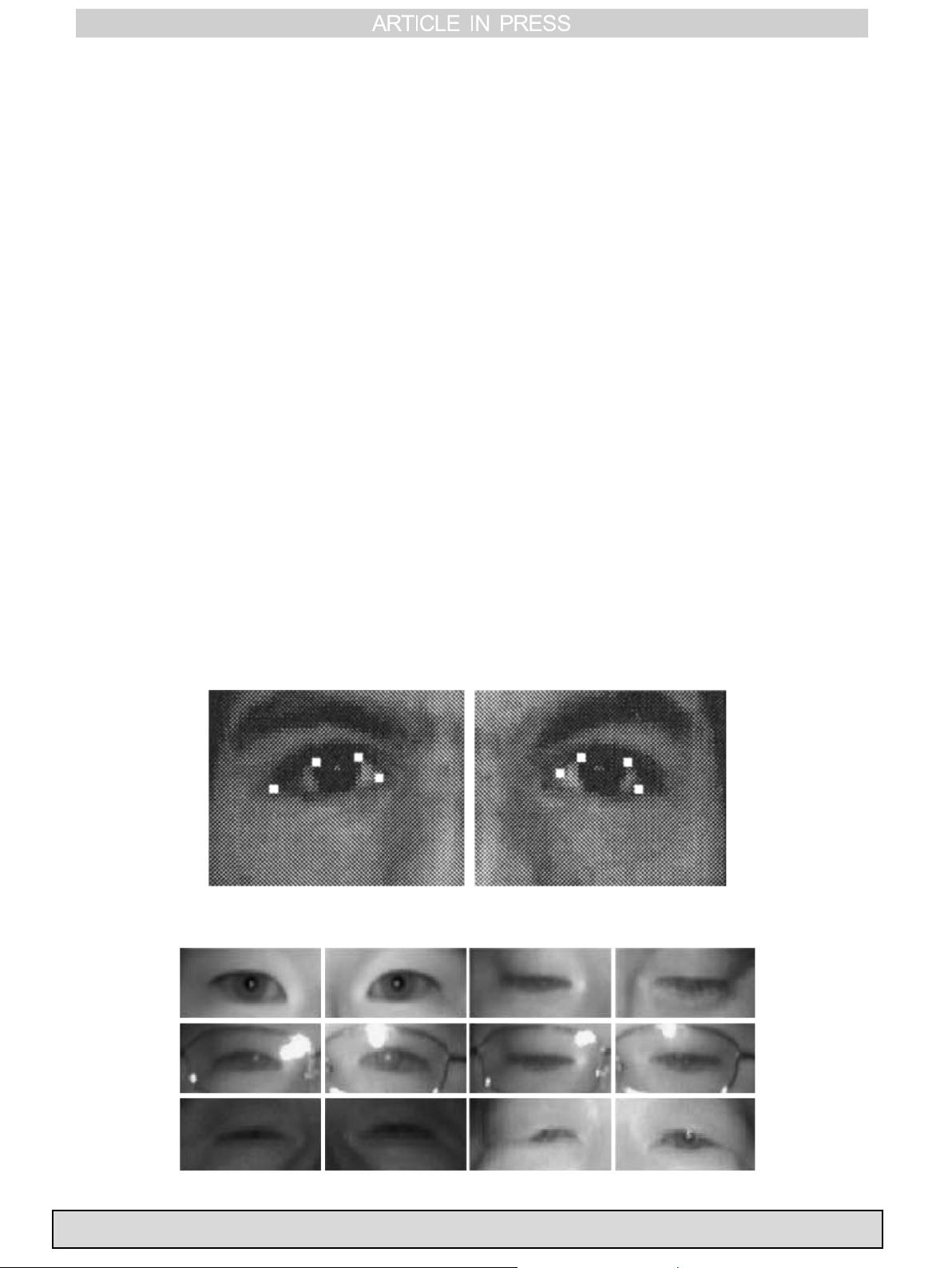
Typical methods to measure such patterns include Variance
Projection Function (VPF) and Integral Projection Function (IPF)
[27], both of which are adopted in General Projection Function
(GPF) [114] under a unified architecture for accurate eye localiza-
tion. These projection functions actually estimate the global inten-
sity distribution around the coarse eye region. However, in some
real-world applications, the global intensity distribution might be
deteriorated by noisy light spots of iris. To address this, [65]
proposed to accumulate locally smoothed version of pixel intensity,
which tends to be more stable compared to the global one.
Alternatively, Wang et al. [99] developed a facial landscape
navigation technique for eye localization, in which the interested
intensity pattern (a pit at the eye center surrounded by hillside) is
searched in a 3D terrain surface manifold of the face. In a similar
method, gradient patterns [53] instead of intensity patterns are
calculated around the eye region and served as the template for
eye matching.
2.1.3. Context characteristics
When the shape or intensity characteristics of the eyes cannot
be reliably measured, the context characteristics are very useful for
eye localization. This is because eyes in the face context usually
have stable relationship with other facial features in terms of both
appearance and structure distribution. Therefore, one may exploit
this prior knowledge to locate the positions of the eyes in the
Bayesian framework. For example, Kawato and Ohya [50] proposed
an eye tracking system through quickly localizing the ‘between-
eyes’ region.
2.1.4. Active infrared lighting characteristics
One of the most effective ways to deal with the lighting
changes is the active near-infrared (NIR) imaging techniques, due
to the fact that under the active IR lighting, the pupil and iris will
show different illumination properties. In particular, the pupil
usually has a larger reflection rate than the iris, resulting in a
bright spot at pupil position. This bright spot is a good indicator of
the pupil and can be used for eye localization [117,116] (cf. Fig. 5).
In practice, a near-infrared light source with a wavelength from
780 to 880 nm will meet the requirements of most in-door
application scenarios. Due to its robustness against visible lighting
changes, this method has been widely used in driver fatigue
detection and face recognition [64]. It should be mentioned,
however, that there exist several conditions (restrictions) that
must be satisfied to ensure good performance, such as opened eye
states and the on-axis light, together with NIR imaging hardware.
Li et al. [64] observed that although active NIR makes the
appearance of the eyes robust to different lighting conditions in
general, the glasses and eye states may cause trouble for precise
eye localization (cf. Fig. 5), and they proposed a tree-structured
detector to carefully address this issue [64].
2.1.5. Discussion
In this section, we summarized several major eye localization
methods which measure different characteristics of eyes, including
the shape, strong intensity contrast, context information, and
active NIR lighting characteristics. It is worth noting that most of
these, except the active NIR technique, are developed at the early
stage of eye localization research and their own limitations
become more pronounced under the complicated uncontrolled
conditions where the characteristics may not be reliably measured
any more. To deal with this problem, most recent eye localization
methods resort to more advanced statistic methods. This is the
main topic of the next section.
2.2. Learning statistical appearance model
In contrast to aforementioned methods where eye-characteristics
with intuitive visual meanings are measured, methods reviewed in
Fig. 4. Corners located for eyes [58].
Fig. 5. Eyes examples under active near-infrared lights [64].
F. Song et al. / Pattern Recognition ∎ (∎∎∎∎) ∎∎∎–∎∎∎4
Please cite this article as: F. Song, et al., A literature survey on robust and efficient eye localization in real-life scenarios, Pattern
Recognition (2013), http://dx.doi.org/10.1016/j.patcog.2013.05.0 09i