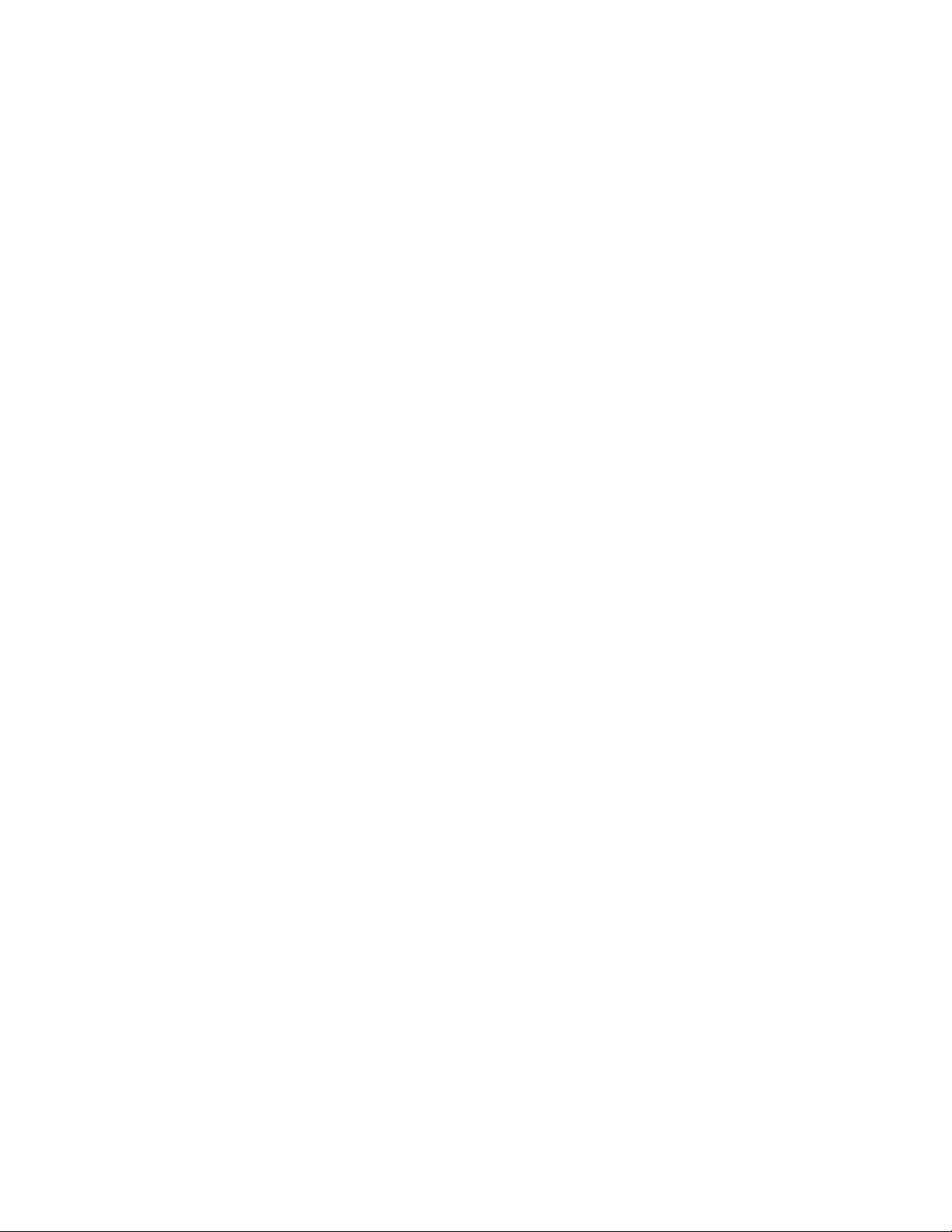
Big Operational Data Oriented Health Diagnosis
Based on Weibull Proportional Hazards Model for
Multi-State Manufacturing System
Yixiao Zhao
School of Reliability and Systems Engineering
Beihang University
Beijing, China
Yihai He, Zhaoxiang Chen, Xiao Han, Zheng He
School of Reliability and Systems Engineering
Beihang University
Beijing, China
Abstract—“Health diagnosis” is the kernel of smart
manufacturing technique, which could monitor system
operational state and predict the impact of performance
degradation on the produced product quality in manufacturing
process systemically. However, previous studies on health
diagnosis have often been depended on the static sensor data of
individual production equipment immoderately while ignoring
the abundant operational performance data of production task
for multi-state manufacturing system. Therefore, a novel mission
reliability modeling of manufacturing system based on the
Weibull proportional hazards model (WPHM) is presented to
diagnose the system health. First, in order to make full use of
abundant operational data, the connotation of manufacturing
system health is proposed. Second, the concept of big operational
data and mission reliability modeling approach based on Quality
state task network (QSTN) are put forward. Third, a health
diagnosis approach is presented with the aid of mission
reliability and WPHM to describe the holistic operational states.
Finally, a case study of a cylinder head manufacturing system
health diagnosis is performed to validate the proposed method.
Keywords—Manufacturing system, health diagnosis, big
operational data, mission reliability modeling, Weibull
proportional hazards model.
I. INTRODUCTION
With the advent of the era of Industry 4.0 and Smart
Manufacturing, manufacturing systems have been becoming
more complicated and predictive [1], which promotes that the
advanced inspection technique and cyber-physical systems
should been adopted to monitor production equipment’s
operational state of various missions during manufacturing
process. In this way, massive operational data could be
collected easily and utilized to provide a solid foundation for
failure analysis and health diagnosis decision-making of
manufacturing systems. Unfortunately, previous studies of
Prognostics and Health Management (PHM) have often been
immoderately depended on the static sensoring data of
individual production equipment while ignored the abundant
operational performance data of production task [2-3]. In
consequence, the holistic health diagnosis approach of
manufacturing systems is not gotten the attention it deserved.
In the field of PHM, there is a kind of data-driven
diagnostic and prognostic method for manufacturing systems
[4], which could provide a firm foundation for potential
operational issues solving and system optimization [5]. Liao
[6] proposed a hidden Markov model(HMM) based on
expectation maximization algorithm for parameters estimation
optimization. Zhang et al. [7] proposed a new
decision-making pattern, and it took advantage of big data and
workshop to achieve intelligent plants. Ni et al. [8]
mentioned
a comprehensive health-state-assessment approach that fused
machine operating records, maintenance data and product
quality information. According to a novel RQR chain, He [9]
presented a method of reliability modeling and optimization
for multi-station manufacturing system. JEFFREY et al. [10]
modeled computer numerical control machine tools
operational reliability through non- and homogeneous Markov
chain.
The Weibull distribution function has a wide range of
applications for researching the components reliability. In
particular, two-parameter Weibull distribution could fit
various types of life data and indicators well instead of using
the normal distribution. Many scholars have gradually turned
their sights to its application in system reliability and
predictive maintenance. Yun [11] described parts failure time
by Weibull distribution for optimal servicing strategy. Chen
[12] calculated the CNC earlier failure period with the aid of
collected failure data and Weibull function. Liao [13]
established the life service model of hydraulic turbine based
on Weibull distribution.
From these previous studies, we can see that owing to the
state changes of materials and machines caused by different
task demands as well as other factors, the reliability of
manufacturing system is actually dynamic in real-time
processes. And the computation of system reliability purely
relied on historical failure data could not meet the
requirements of predictive health diagnosis in operation.
Therefore, a new connotation and algorithm for mission
reliability model based on Quality state task network (QSTN)
is elaborated, and with the aid of the Weibull proportional
hazards model, the health state of manufacturing system
analyzed by reliability index estimation. The final result
shows that it could also guide further predictive production
decision-making.
444
2018 Prognostics and System Health Management Conference
2166-5656/18/$31.00 ©2018 IEEE
DOI 10.1109/PHM-Chongqing.2018.00082