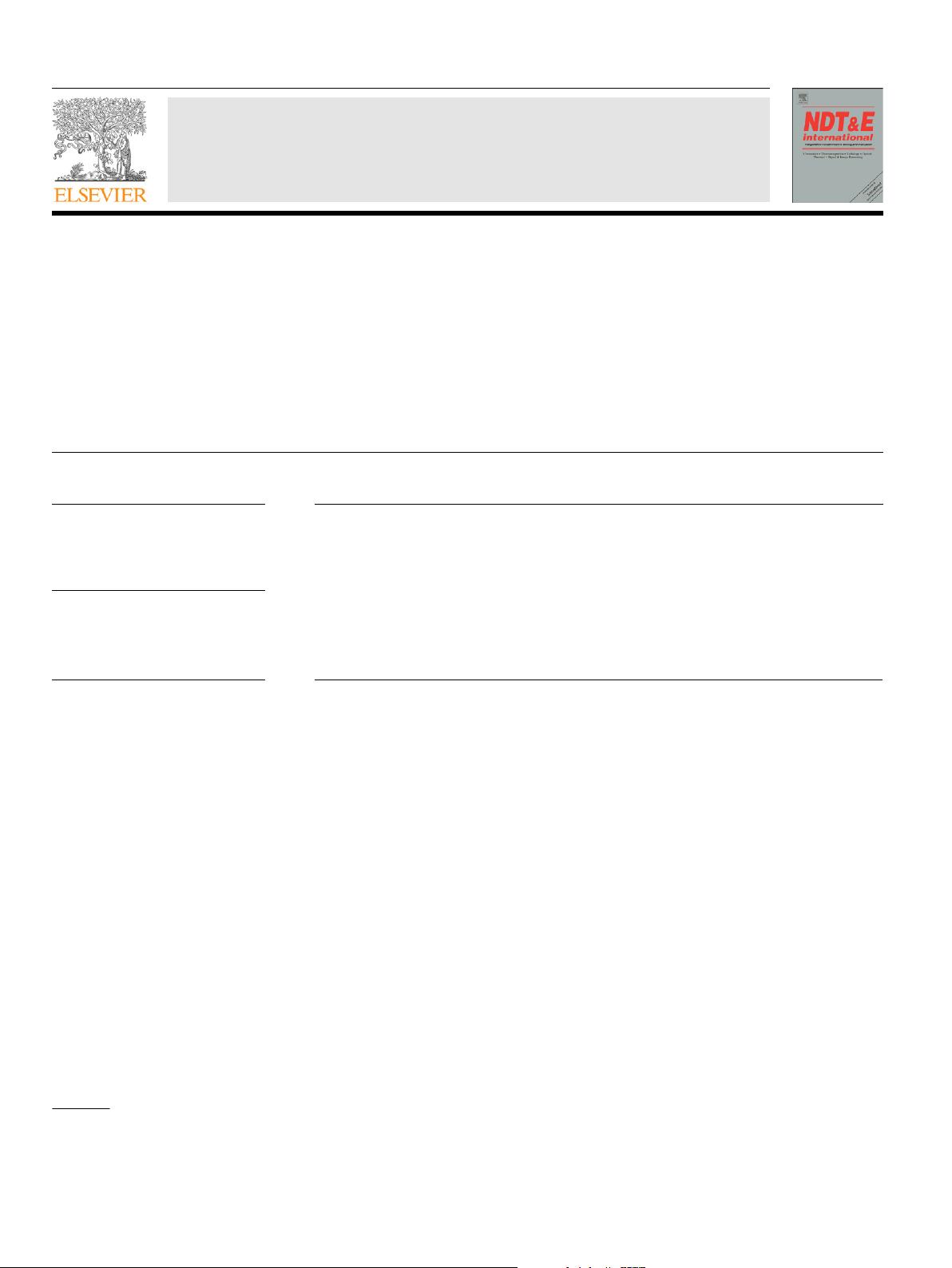
Support vector machines based defect recognition in SonicIR using 2D heat
diffusion features
Zhi Zeng
a,b
, Jing Zhou
c
, Ning Tao
b
, Lichun Feng
b
, Cunlin Zhang
b,
n
, Xiaoyan Han
d,
n
a
College of Physics and electronic engineering, ChongQing Normal University, ChongQing 400047, PR China
b
Beijing Key Laboratory for Terahertz Spectroscopy and Imaging, Key Laboratory of Terahertz Optoelectronics, Ministry of Education, Department of Physics, Capital Normal
University, Beijing 100048, PR China
c
State Key Laboratory of Power Transmission Equipment & System Security and New Technology, College of Electrical Engineering, ChongQing University, ChongQing,
400030, PR China
d
Department of Electrical and Computer Engineering, Wayne State University, Detroit, MI 48202, USA
article info
Article history:
Received 4 December 2010
Received in revised form
26 October 2011
Accepted 1 November 2011
Available online 8 January 2012
Keywords:
Heat diffusion
Defect signal
SonicIR
Support vector machines
abstract
In SonicIR, when a single short pulse of 20 or 40 kHz sound wave passes through materials with
mechanical discontinuities, e.g., cracks with faying surfaces, it will ordinarily cause heating of those
surfaces. This study investigates the effect of support vector machines (SVM), which is a machine-
learning method based on the principle of structural risk minimization, as a classifier tool to identify
defects in SonicIR image sequences. One inconel sample with a known defect has been chosen to
construct the training set, and the 2D heat diffusion patterns of defect and disturbing signals at two
different times during the sonic pulse have been chosen as features to be used in the classification
procedure. A two stages SVM classifier has been employed to recognize defects in 80 inconel and
60 titanium samples, the results indicate that SVM is a promising tool for defect recognition in SonicIR
image sequences.
& 2012 Elsevier Ltd. All rights reserved.
1. Introduction
SonicIR is a nondestructive technology using a sonic pulse to
pass through a material with a mechanical discontinuity, such as
a crack with faying surfaces, and cause heating of those surfaces
[1–7]. This heat appears bright in the experimental image as the
indication of defects. In SonicIR image analysis, usually the
amount of the generated heat is very small and most micro-crack
defects are invisible in the original image. Therefore it is neces-
sary to analyze the bright areas in the image which subtracted the
reference image before sonic excitation. In most of practical
applications, the situation is complicated: the micro fatigue crack
may not appear very bright in the images, while the disturbing
signals may appear much brighter than defect signals, such as
heat reflection, friction heat generated between sample and post,
bad paint and random noise. Those disturbing signals have
different 2D heat diffusive patterns compared with defect signals,
which could be used in defect recognition.
In this paper, we investigate the potential of support vector
machines (SVM) in detecting defect signals in SonicIR image
sequences. SVM is based on the principle of structural risk
minimization, and it attempts to minimize a bound on the
generalization error [8]. The basic idea is to find a hyperplane
that separates the d-dimensional data into two classes: defect or
non-defect. SVM has found a wide range of real-world applica-
tions, including handwritten digit recognition, speaker identifica-
tion, face detection, etc [9]. While currently there is no automated
system for inspection and analysis of SonicIR images, the objec-
tive of this study is to develop a method based on SVM using 2D
heat diffusion features to automatically recognize defect signals
in SonicIR image sequences that may contain lots of disturbing
signals. Since some of the disturbing signals may have similar
patterns as defect signal, a two stages SVM has been proposed to
process 80 inconel and 60 titanium samples, and the results have
indicated that SVM is a promising tool for defect recognition in
SonicIR image sequences.
2. An overview of SonicIR imaging
2.1. Experimental setup
The experimental setup of SonicIR is shown in Fig. 1: the metal
sample is held by posts at two ends, then a sonic pulse is coupled
into sample at the left side through a piece of duct tape, and an
Contents lists available at SciVerse ScienceDirect
journal ho mepage: www.elsevier.com/locate/ndteint
NDT&E International
0963-8695/$ - see front matter & 2012 Elsevier Ltd. All rights reserved.
doi:10.1016/j.ndteint.2011.11.001
n
Corresponding authors.
E-mail addresses: cunlin_zhang@cnu.edu.cn (C. Zhang),
xhan@eng.wayne.edu (X. Han).
NDT&E International 47 (2012) 116–123