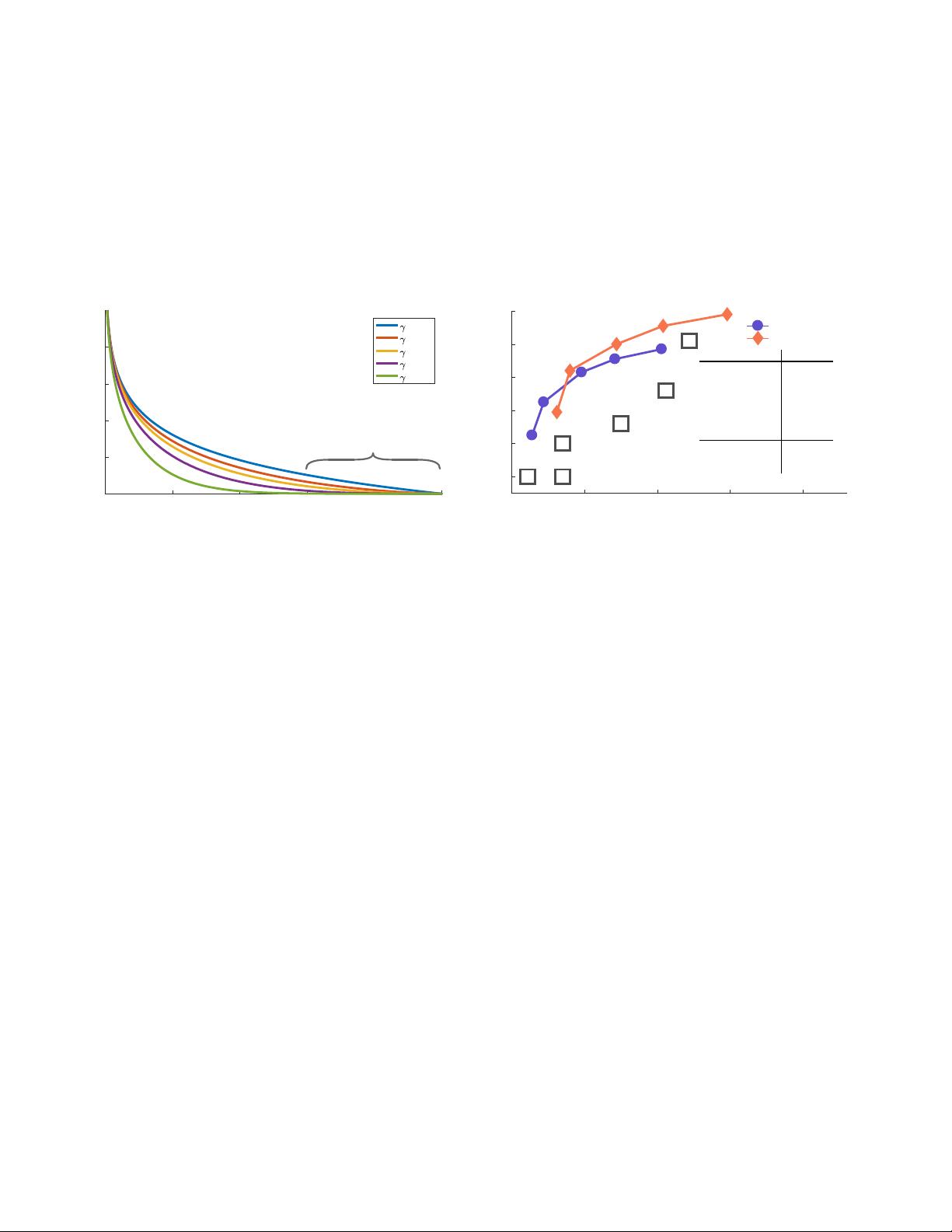
Focal Loss for Dense Object Detection
Tsung-Yi Lin Priya Goyal Ross Girshick Kaiming He Piotr Doll
´
ar
Facebook AI Research (FAIR)
0 0.2 0.4 0.6 0.8 1
probability of ground truth class
0
1
2
3
4
5
loss
= 0
= 0.5
= 1
= 2
= 5
well-classied
examples
well-classied
examples
CE(p
t
) = − log(p
t
)
FL(p
t
) = −(1 − p
t
)
γ
log(p
t
)
Figure 1. We propose a novel loss we term the Focal Loss that
adds a factor (1 − p
t
)
γ
to the standard cross entropy criterion.
Setting γ > 0 reduces the relative loss for well-classified examples
(p
t
> .5), putting more focus on hard, misclassified examples. As
our experiments will demonstrate, the proposed focal loss enables
training highly accurate dense object detectors in the presence of
vast numbers of easy background examples.
Abstract
The highest accuracy object detectors to date are based
on a two-stage approach popularized by R-CNN, where a
classifier is applied to a sparse set of candidate object lo-
cations. In contrast, one-stage detectors that are applied
over a regular, dense sampling of possible object locations
have the potential to be faster and simpler, but have trailed
the accuracy of two-stage detectors thus far. In this paper,
we investigate why this is the case. We discover that the ex-
treme foreground-background class imbalance encountered
during training of dense detectors is the central cause. We
propose to address this class imbalance by reshaping the
standard cross entropy loss such that it down-weights the
loss assigned to well-classified examples. Our novel Focal
Loss focuses training on a sparse set of hard examples and
prevents the vast number of easy negatives from overwhelm-
ing the detector during training. To evaluate the effective-
ness of our loss, we design and train a simple dense detector
we call RetinaNet. Our results show that when trained with
the focal loss, RetinaNet is able to match the speed of pre-
vious one-stage detectors while surpassing the accuracy of
all existing state-of-the-art two-stage detectors.
50 100 150 200 250
inference time (ms)
28
30
32
34
36
38
COCO AP
B C
D
E
F
G
RetinaNet-50
RetinaNet-101
AP time
[A] YOLOv2
†
[26] 21.6 25
[B] SSD321 [21]
28.0 61
[C] DSSD321 [9] 28.0 85
[D] R-FCN
‡
[3] 29.9 85
[E] SSD513 [21] 31.2 125
[F] DSSD513 [9] 33.2 156
[G] FPN FRCN [19] 36.2 172
RetinaNet-50-500 32.5 73
RetinaNet-101-500 34.4 90
RetinaNet-101-800 37.8 198
†
Not plotted
‡
Extrapolated time
Figure 2. Speed (ms) versus accuracy (AP) on COCO test-dev.
Enabled by the focal loss, our simple one-stage RetinaNet detec-
tor outperforms all previous one-stage and two-stage detectors, in-
cluding the best reported Faster R-CNN [27] system from [19]. We
show variants of RetinaNet with ResNet-50-FPN (blue circles) and
ResNet-101-FPN (orange diamonds) at five scales (400-800 pix-
els). Ignoring the low-accuracy regime (AP<25), RetinaNet forms
an upper envelope of all current detectors, and a variant trained for
longer (not shown) achieves 39.1 AP. Details are given in §5.
1. Introduction
Current state-of-the-art object detectors are based on
a two-stage, proposal-driven mechanism. As popularized
in the R-CNN framework [11], the first stage generates a
sparse set of candidate object locations and the second stage
classifies each candidate location as one of the foreground
classes or as background using a convolutional neural net-
work. Through a sequence of advances [10, 27, 19, 13], this
two-stage framework consistently achieves top accuracy on
the challenging COCO benchmark [20].
Despite the success of two-stage detectors, a natural
question to ask is: could a simple one-stage detector achieve
similar accuracy? One stage detectors are applied over a
regular, dense sampling of object locations, scales, and as-
pect ratios. Recent work on one-stage detectors, such as
YOLO [25, 26] and SSD [21, 9], demonstrates promising
results, yielding faster detectors with accuracy within 10-
40% relative to state-of-the-art two-stage methods.
This paper pushes the envelop further: we present a one-
stage object detector that, for the first time, matches the
1
arXiv:1708.02002v1 [cs.CV] 7 Aug 2017