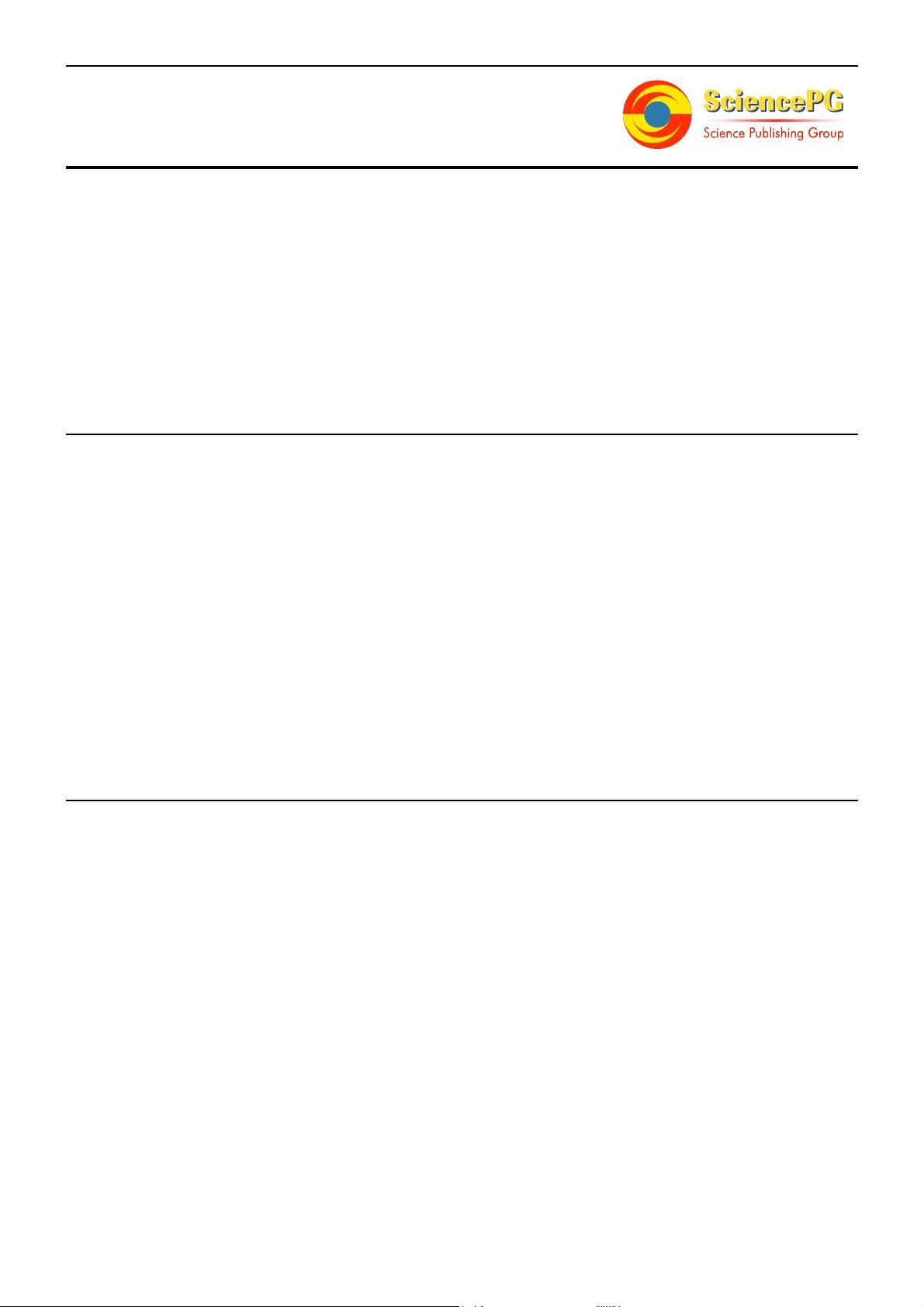
American Journal of Theoretical and Applied Statistics
2015; 4(6): 504-512
Published online October 29, 2015 (http://www.sciencepublishinggroup.com/j/ajtas)
doi: 10.11648/j.ajtas.20150406.21
ISSN: 2326-8999 (Print); ISSN: 2326-9006 (Online)
Modeling Survival Data by Using Cox Regression Model
Medhat Mohamed Ahmed Abdelaal
*
, Sally Hossam Eldin Ahmed Zakria
Statistics and Mathematics Department, Faculty of Commerce, Ain Shams University, Cairo, Egypt
Email address:
medhatal@commerce.asu.edu.eg (M. M. A. Abdelaal), sally.hossam21@yahoo.com(S. H. Eldin Ahmed Zakria)
To cite this article:
Medhat Mohamed Ahmed Abdelaal, Sally Hossam Eldin Ahmed Zakria. Modeling Survival Data by Using Cox Regression Model. American
Journal of Theoretical and Applied Statistics. Vol. 4, No. 6, 2015, pp. 504-512. doi: 10.11648/j.ajtas.20150406.21
Abstract:
Survival analysis refers to the general set of statistical methods developed specifically to model the timing of
events. A popular regression model for the analysis of survival data is the Cox proportional hazards regression model. The Cox
regression model is a semi parametric model, making fewer assumptions than typical parametric methods but more
assumptions than those nonparametric methods. The main objective of this paper is to construct Cox proportional hazards
regression model for examining the covariate effects on the hazard function and to determine the risk factors affecting the
outcome of liver transplantation operation for end-stage liver disease. This article will focus on a review of (a) the Cox model
and interpretation of its results, (b) assessment of the validity of the PH assumption, and (c) accommodating non-proportional
hazards using covariate stratification. Cox PH model showed that the variables: Recipient age,
, Ln_Creatinine,
and GRWR are statistically significant and selected as significant factors for risk of death after liver transplantation operation.
Also the scaled Schoenfeld residual displayed non-proportionality for variable Recipient Age and this variable needed to be
stratified. And the Cox-Snell residual showed the Cox PH model does not fit these data adequately. So the stratified Cox model
could be more appropriate to the current study. The stratified Cox model with interaction and with no interaction were applied
and showed that the no-interaction model is acceptable at 0.05 level of significance and the variables
,
Ln_Creatinine are statistically significant and selected as significant factors for risk of death after liver transplantation
operation at 0.05 level of significance.
Keywords:
Survival Analysis, Censoring, Cox Proportional Hazard Regression Model, Cox- Snell Residual,
Stratified Cox Regression Model
1. Introduction
The most common approach to model covariate effects on
survival is the Cox proportional hazard model, which can
handle censored and/or truncated observations [1].
Regression analysis is generally used for identifying the risk
factors. But due to the presence of censoring in survival data,
ordinary regression models cannot be used. Also simple
logistic regression analysis has the limitation of only
allowing a view of survival probability over the entire study
period as a single time interval and it assume that every
patient is at risk over the entire study period. This is not valid
for studies with long follow up or other situations where
patients have variable time at risk. For this purpose, in
survival analysis, Cox’s regression model is widely
applicable.
The distinguishing feature of Cox PH model is its ability to
estimate the relationship between the hazard rate and
explanatory variables without having to make any
assumptions about the shape of the baseline hazard function.
Hence the Cox model is sometimes referred to as a semi-
parametric model.
The Cox regression model is a statistical theory of
counting processes that unifies and extends nonparametric
censored survival analysis. The approach integrates the
benefits of nonparametric and parametric approaches to
statistical inferences [2].
The Cox proportional hazards regression model relates
covariates to the hazard function as follows:
(1)
Where
is called the baseline hazard function, which
is the hazard function for an individual for whom all the
variables included in the model are zero,
=
is a parameter vector of regression
coefficients,
is the value of the vector of
explanatory variables for a particular individual, and is a
fixed, known scalar function [3].