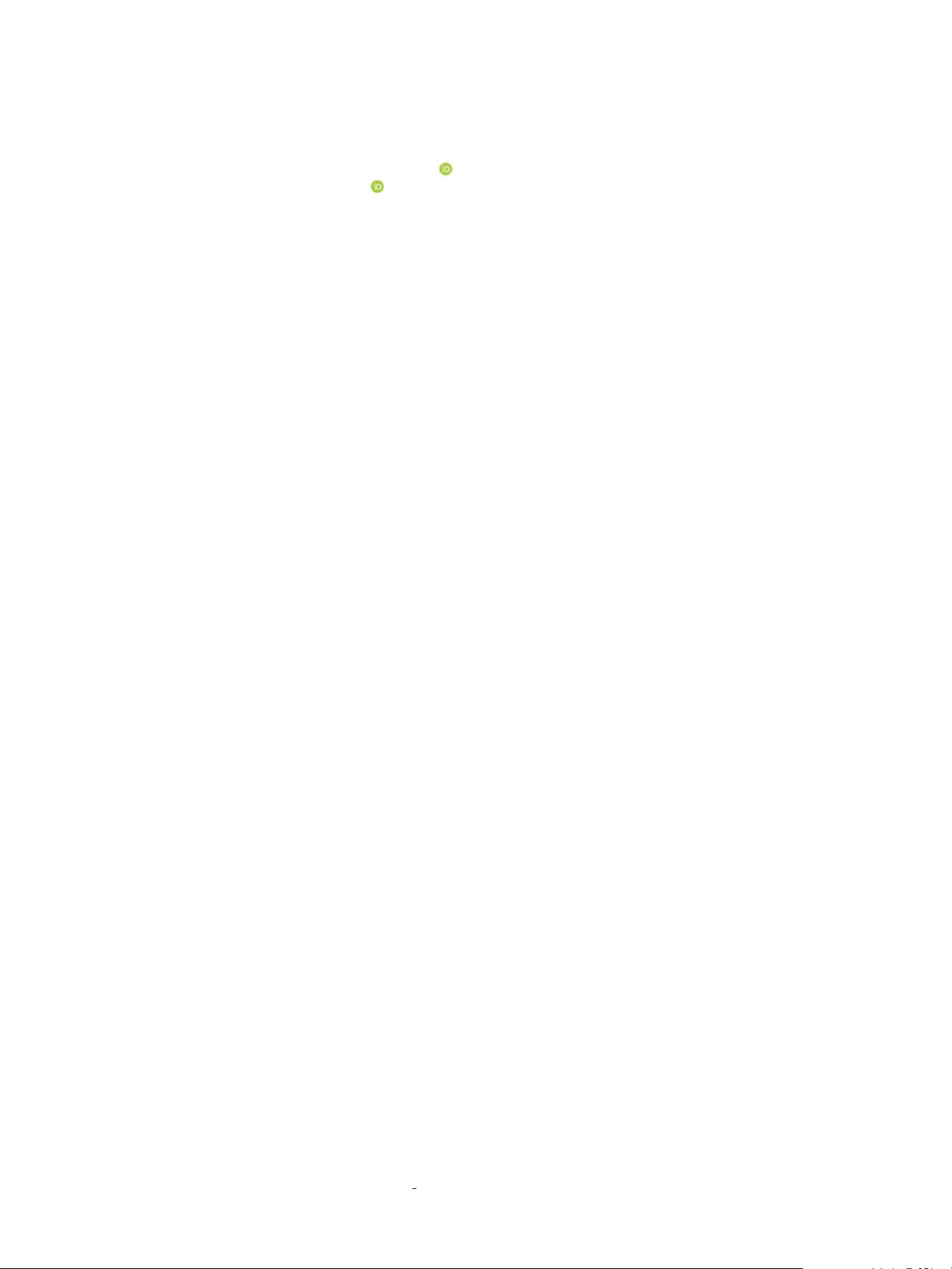
9592 IEEE TRANSACTIONS ON VEHICULAR TECHNOLOGY, VOL. 66, NO. 10, OCTOBER 2017
Carrier Frequency Offset Estimation in Uplink OFDMA
Systems: An Approach Relying on Sparse Recovery
Min Huang, Lei Huang, Senior Member, IEEE, Chongtao Guo ,
Peichang Zhang, Jihong Zhang, and Lie-Liang Yang
, Fellow, IEEE
Abstract—This paper proposes a novel blind carrier frequency offset
(CFO) estimator, namely the sparse recovery assisted CFO (SR-CFO)
estimator, for the uplink orthogonal frequency-division multiple access
(OFDMA) systems. By exploiting the sparsity embedded in the OFDMA
data, the CFO estimation is formulated as an optimization problem of
sparse recovery with high-resolution. Meanwhile, in order to enhance the
estimation accuracy of CFOs, background noise and sampling errors are
mitigated by exploiting the structure of the noise covariances matrix in
the transformed observation data, and the asymptotic distribution of the
sampling errors. Furthermore, we propose an approach for deriving the
regularization parameter used by the SR-CFO estimator, so as to control
the tradeoff between the data fitting error and the sparsity of solution.
The performance of the proposed SR-CFO estimator along with other four
existing estimators is investigated and compared. Numerical results show
that the proposed SR-CFO estimator is superior to the state-of-the-art
estimators in terms of the estimation reliability.
Index Terms—OFDMA, carrier frequency offset, estimator, sparse
recovery.
I. INTRODUCTION
In wireless communications, orthogonal frequency-division multi-
plexing (OFDM) has been widely employed to support the downlink
and uplink transmissions, and to support multiuser communications
via assigning different subcarriers to different users, forming the or-
thogonal frequency division multiple access (OFDMA). In OFDMA
systems, the achievable performance is very sensitive to the carrier fre-
quency offsets (CFOs) induced by the mismatch between transceiver
oscillators. CFOs lead to strong multiple-access interference ( MAI),
which may significantly degrade the system performance if it is not
Manuscript received January 18, 2017; revised April 3, 2017 and May
16, 2017; accepted May 21, 2017. Date of publication May 24, 2017; date
of current version October 13, 2017. This work was supported in part by
the Natural Science Foundation of China under Grants U1501253, 61601300,
6160010632, 61501300, 61601304, and 61601307, in part by the Natural Sci-
ence Foundation of Guangdong Province under Grant 2015A030311030, in
part by the Foundation of Shenzhen under Grants JCYJ20150324140036835,
JCYJ20160520165659418, and JCYJ20160422102022017, in part by the
National Science Foundation under Grant CCF-1218388, in part by the
China Postdoctoral Science Foundation under Grant 2016M592535, in part
by the Shenzhen Key Laboratory Establishment Foundation under Grants
ZDSYS201507081625213 and KC2015ZDYF0023A, and in part by the China
Scholarship Council. The work of L.-L. Yang was supported by the EPSRC,
U.K. The review of this paper was coordinated by Prof. R. Dinis. (Corresponding
author: Lei Huang.)
M. Huang, L. Huang, C. Guo, P. Zhang, and J. Zhang are with the Col-
lege of Information Engineering, Shenzhen University, Shenzhen 518060,
China (e-mail: mhuang@szu.edu.cn; lhuang@szu.edu.cn; ctguo@szu.edu.cn;
zpc1029384756@hotmail.com; zhangjh@szu.edu.cn).
L.-L. Yang is with the School of Electronics and Computer Science, Uni-
versity of Southampton, Southampton SO17 1BJ ,U.K. (e-mail: lly@ecs.
soton.ac.uk).
Color versions of one or more of the figures in this paper are available online
at http://ieeexplore.ieee.org.
Digital Object Identifier 10.1109/TVT.2017.2707671
effectively mitigated. Hence, in practical systems, the CFOs need to be
estimated with sufficient accuracy which are then compensated or used
for MAI mitigation.
In literature, maximum likelihood algorithm [1] and its variant ver-
sions [2], [3] have been proposed for estimation of the CFOs in OFDMA
systems. The common drawbacks of these methods are the high imple-
mentation complexity and the requirement of pilots, which reduces the
attainable spectral efficiency. In order to combat these problems, a blind
CFO estimation scheme has been proposed in [4]. Specially, by ex-
ploiting the periodic structure of the received signals in the interleaved
OFDMA uplink systems, the multiple signal classification approach
referred to as MUSIC has been adopted in [4] to estimate the CFOs.
It is shown in [4] that the MUSIC algorithm has a low computational
complexity, and is capable of achieving a good estimation performance.
However, when the CFOs of the signals from different users are close
to each other in the frequency domain, and when the signal-to-noise
ratio (SNR) is relatively low, it is usually hard to separate the CFOs
by the MUSIC approach. Furthermore, in order to identify the CFOs,
the MUSIC approach has to resort to a highly involved spectral search-
ing procedure, which is rather computationally intensive. Due to these
issues, the MUSIC estimator has been replaced by the estimators de-
signed based on the rotational invariance technique, such as the ESPRIT
[5]. Moreover, in order to further reduce the computational complex-
ity, an improved ESPRIT, namely the Unitary-ESPRIT, has been pro-
posed in [6] for estimation of CFOs. More recently, a unitary subspace
improved minimum output variance (U-SIMOV) estimator has been
introduced in [7] for CFO estimation. By combining the unitary trans-
formation with the subspace projection technique, the U-SIMOV is able
to improve the CFO estimation performance of the Unitary-ESPRIT,
provided that the CFOs with respect to different users are sufficiently
separated from each other.
In order to attain high-resolution estimations for the closely located
CFOs, especially, in low SNR region, we propose a novel CFO estima-
tor in this paper, with the main contributions as follows.
1) A novel CFO estimation algorithm designed based on the sparse
recovery is proposed in this paper, which is referred to as the
sparse recovery assisted CFO (SR-CFO) estimator. Our high-
resolution CFO estimator is formulated as a sparse optimization
problem without relying on requiring pilots. Furthermore, with
the aid of the Lagrangian duality theory [8], [9], an approach is
proposed for deriving the regularization parameter required by
our SR-CFO estimator.
2) A selection matrix is designed for mitigating the effect of additive
Gaussian noise by exploiting the structure of the noise variances
in the transformed observation data.
3) A whitening filter is designed for coping with the sampling er-
rors by exploiting the asymptotic distribution of the sampling
errors.
4) The performance of our SR-CFO estimator is investigated by
simulations, which is also compared with four existing CFO
estimators. Our studies and simulation results show that the pro-
posed SR-CFO estimator outperforms all the benchmark CFO
estimators by providing a higher resolution and also a higher
0018-9545 © 2017 IEEE. Personal use is permitted, but republication/redistribution requires IEEE permission.
See http://www.ieee.org/publications
standards/publications/rights/index.html for more information.