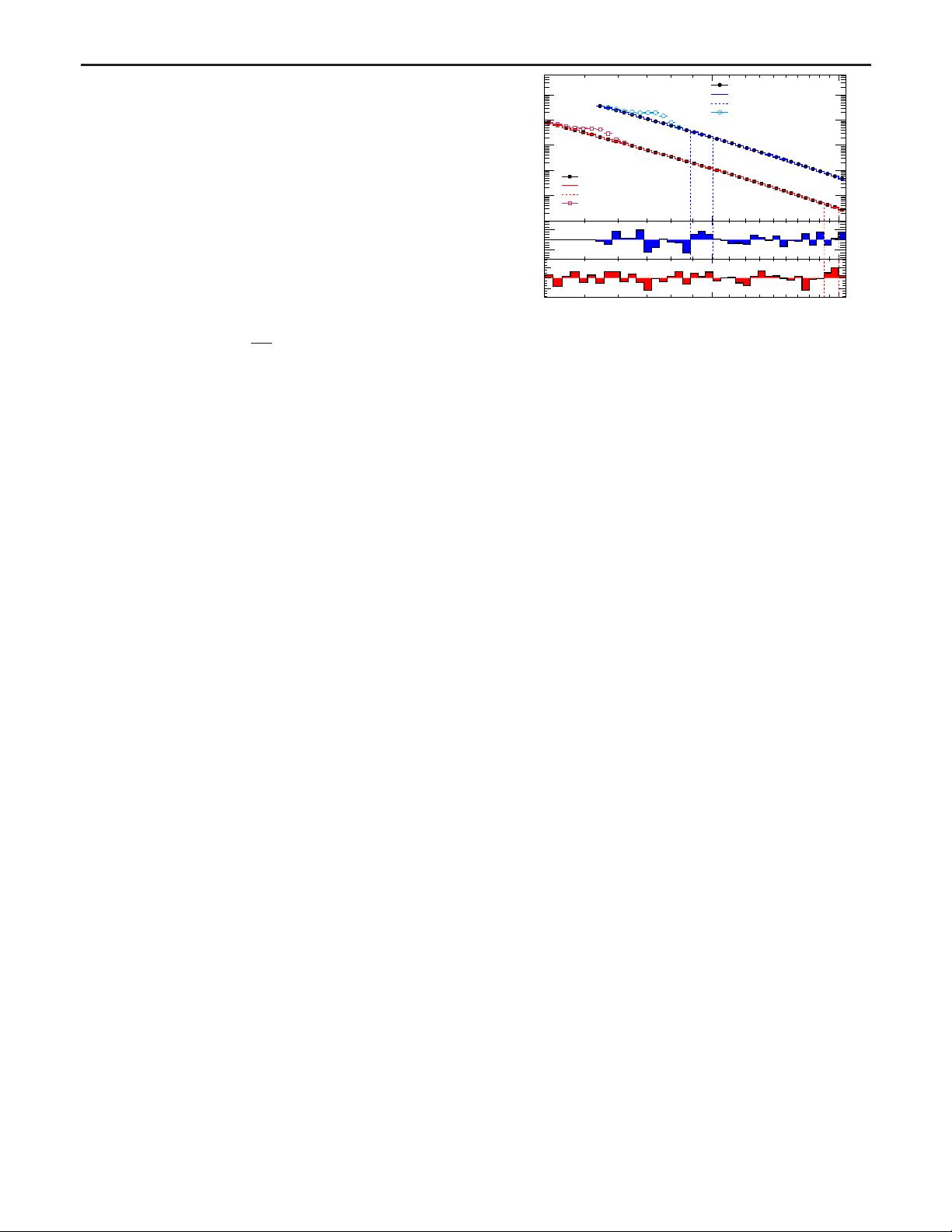
beyond the range, to 2970 (3490) GeV for the jy
j < 0.6
(0.3) signal selection, and the fit is evaluated at the center of
the window.
In each sliding window, three functional forms are fit to
the data: a five-parameter function of the form
fðxÞ¼p
1
ð1 − xÞ
p
2
x
p
3
þp
4
ln xþp
5
ln x
2
; ð1Þ
where p
i
are free parameters and x ≡ m
jj
=
ffiffiffi
s
p
; a four-
parameter function, which is the same as Eq. (1) but with
p
5
¼ 0; and a four-parameter function used by the UA2
Collaboration [28], defined as
fðxÞ¼
p
1
x
p
2
e
−p
3
x−p
4
x
2
: ð2Þ
The function used for each signal selection is the one that
yields the best χ
2
over the full fitted m
jj
range. An
alternative function is chosen to evaluate a systematic
uncertainty. For the signal selection with jy
j < 0.6,
Eq. (1) is used, yielding a χ
2
p value of 0.13, while the
alternative function is the four-parameter function with a χ
2
p value of 0.11. For the signal selection with jy
j < 0.3, the
four-parameter version of Eq. (1) yields the best χ
2
p value
of 0.42 and the alternative function is Eq. (2), with a χ
2
p value of 0.35.
The size of the sliding window is optimized to yield the
best χ
2
value for the full m
jj
range while still being larger
than the width of the expected signals and therefore
insensitive to potential signal contributions. This latter
requirement is checked by including signal models in
pseudo-data samples and studying the dependence of the
signal sensitivity on different window sizes.
Systematic uncertainties in the estimate of the back-
ground used in setting limits include the uncertainty due to
the choice of functional form and uncertainties in the fit
parameter values. The effect of the choice of functional
form is evaluated by comparing the nominal function to the
alternative. The uncertainties in the fit parameter values are
evaluated using pseudoexperiments, where the pseudodata
are drawn from Poisson fluctuations around the nominal
background model.
Results and limits.—Figure 4 shows the invariant mass
distributions for dijet events in each signal region including
the results from the sliding-window background estimates.
The χ
2
p value of the overall background is 0.13 for the
jy
j < 0.6 signal selection and 0.42 for the jy
j < 0.3 signal
selection, indicating the data agrees well with the back-
ground estimate. The most discrepant interval identified by
the BumpHunter algorithm [33,34] is 889–1007 GeV for
events with jy
j < 0.6. Accounting for statistical uncer-
tainties only, the probability of observing a deviation at
least as significant as that observed in data, anywhere in this
distribution, is 0.44 and corresponds to significance of
0.16σ. Thus, there is no evidence of any localized excess.
Limits are set on both a leptophobic Z
0
simplified
dark-matter model [36] and a generic Gaussian model.
The Z
0
simplified model assumes axial-vector couplings to
SM quarks and to a Dirac fermion dark-matter candidate.
No interference with the SM is simulated. Signal samples
were generated so that the decay rate of the Z
0
into dark-
matter particles is negligible and the dijet production rate
and resonance width depend only on the coupling of the Z
0
to quarks, g
q
, and the mass of the resonance, m
Z
0
[9]. The
model’s matrix elements were calculated in M
AD
G
RAPH
5
[37] and parton showering was performed in P
YTHIA
8 [38]
with the A14 set of tuned parameters for underlying event
[39] and NNPDF2.3 parton distribution functions [40].
The width of a Z
0
resonance with g
q
¼ 0.10, including
parton shower and detector resolution effects, is approx-
imately 7%. Limits are set on the cross section, σ, times
acceptance, A, times branching ratio, B, of the model, and
then displayed in the ðg
q
;m
Z
0
Þ plane [41]. The acceptance
for a mass of 550 GeV is 20% for a Z
0
simplified model
with g
q
¼ 0.10 for the jy
j < 0.3 signal selection, and 41%
for a signal of mass equal to 750 GeV for the jy
j < 0.6
signal selection.
Limits are also set on a generic model where the signal is
modeled as a Gaussian contribution to the observed m
jj
distribution. For a given mean mass, m
G
, four different
Gaussian widths are considered: a width equal to the
simulated mass resolution (which ranges between 4%
and 6%), and the fixed fractions 5%, 7%, and 10% of
m
G
. As the width increases, the expected signal contribu-
tion is distributed across more bins. Wider signals are
Events / Bin
4
10
5
10
6
10
7
10
8
10
[GeV]m
500 600 700 800 900
1000
2−
0
2
[GeV]
jj
m
500 600 700 800 900
1000
2000
2−
0
2
Significance
ATLAS
, |y*| < 0.3
-1
Data, 3.6 fb
Background fit
BumpHunter interval
x 500σZ’,
= 0.1
q
= 550 GeV, g
Z’
m
, |y*| < 0.6
-1
Data, 29.3 fb
Background fit
BumpHunter interval
x 500σZ’,
= 0.1
q
= 750 GeV, g
Z’
m
value = 0.6pBH
value = 0.42p
2
χ
value = 0.44pBH
value = 0.13p
2
χ
FIG. 4. The reconstructed dijet mass distribution (filled points)
for events in the jy
j < 0 . 3 and jy
j < 0 . 6 signal regions. Solid
lines depict the background estim ate obtained by a sliding-
window fit. Overall agreement between the background estimate
and the data is quantified by the χ
2
p value. The most discrepant
localized excess in either signal region identified by the Bump-
Hunter algorithm is indicated by the vertical lines. The open
points show two possible signal models. The lower panels show
the bin-by-bin significances of differences between the data and
the background estimate, considering only statistical uncertain-
ties (see Ref. [35]).
PHYSICAL REVIEW LETTERS 121, 081801 (2018)
081801-4