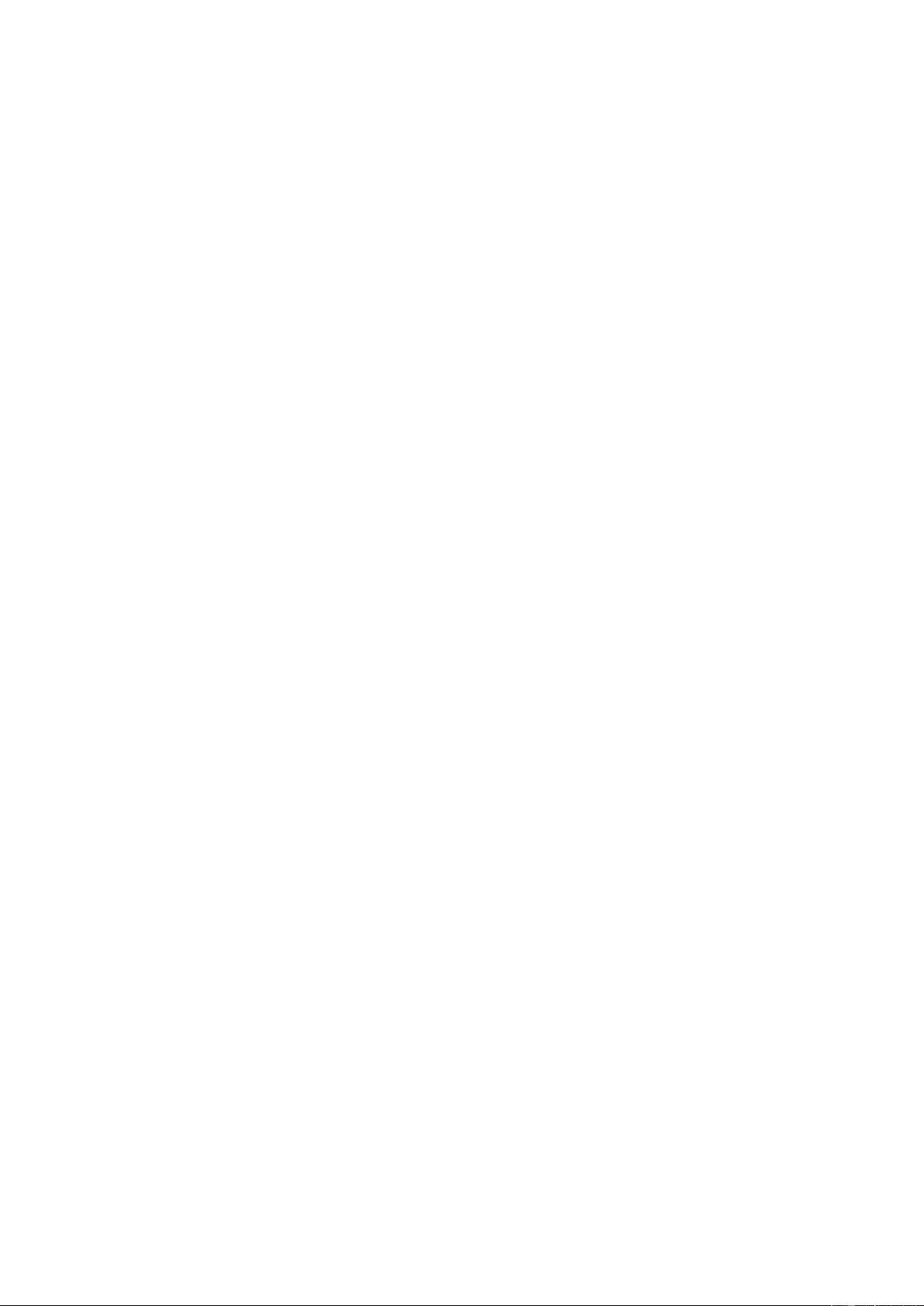
Comparison of optical and concentration feature used for fNIRS-based
BCI system using HMM
XU Baolei
12,a
, Fu Yunfa
3,b
, Shi Gang
1,c
, Yin Xuxian
12,d
, Miao Lei
1,e
Wang Zhidong
1,f
, Li Hongyi
15,g
1
State Key Laboratory of Robotics, Shenyang Institute of Automation (SIA), Chinese Academy of
Sciences (CAS), Shenyang 110016, P. R. China
2
University of Chinese Academy of Sciences, Beijing 100049, P. R. China
3
School of Automation and Information Engineering, Kunming University of Science and Technology,
Kunming 650500, P. R. China
4
Dept. of Advanced Robotics, Chiba Institute of Technology, Chiba 2750016, Japan
5
School of Mechanical Engineering & Automation, Northeastern University, Shenyang, China
a
blxu@sia.cn,
b
fyf@ynu.edu.cn,
c
sg0105@sia.cn,
d
yinxuxian@sia.cn,
e
miaolei@sia.cn,
f
zhidong.wang@it-chiba.ac.jp,
g
hli@sia.cn
Keywords: Brain-Computer Interface (BCI), functional Near-Infrared Spectroscopy (fNIRS), Hidden
Morkov Model (HMM), feature selection.
Abstract. Brain-Computer Interface (BCI) is very useful for people who lose limb control such as
amyotrophic lateral sclerosis (ALS) patients, stroke patients and patients with prosthetic limbs.
Among all the brain signal acquisition devices, functional near-infrared spectroscopy (fNIRS) is an
efficient approach to detect hemodynamic responses correlated with brain activities using optical
method, and its spatial resolution is much higher than EEG. In this paper, we investigate the
classification performance of both optical signal and hemodynic signal that both used in fNIRS-based
BCI system using Hidden Markov Model (HMM). Our results show that hemodynamic signal has a
much lower error rate than optical signal, especially the Oxy-hemoglobin (HbO) has the lowest error
rate. This result is important for researchers who want to design an fNIRS-based BCI system and get
better performance.
Introduction
Brain-Computer Interface (BCI) is a technology to control an outside device using brain signals
directly, which is especially important for people who lose limb control such as amyotrophic lateral
sclerosis (ALS) patients, stroke patients and patients with prosthetic limbs. The concept of BCI was
first funded by America Defense Advanced Research Projects Agency (DARPA) in 1970s [1], and get
great development since 1990s [2]. Currently, there are more than 300 institutions doing BCI related
researches. Its applications include rehabilitation [3], games control [4], virtual environment [5],
robot control [6], car control [7], prosthetics control [8], wheel chair control [9] and military weapon
control [10].
The brain signal used for BCI control can be acquired noninvasively from many equipments, such as
electroencephalograph (EEG) [11], magnetoencephalography (MEG) [12], functional magnetic
resonance imaging (fMRI) [13], and functional near-infrared spectroscopy (fNIRS) [14]. The EEG is
the most used method because its high sampling frequency, small size and low cost compared with
MEG and fMRI. However, the spatial resolution of EEG is very low due to the capacitance resistance
effect of cerebro-spinal fluid and the skull. Fortunately, fNIRS provides another way to investigate
brain activities through hemodynamics approach with higher spatial resolution than EEG [15].
Furthermore, the signals acquired by fNIRS is highly related to fMRI signals [16], but the price of
fNIRS equipment is an order lesser than fMRI.