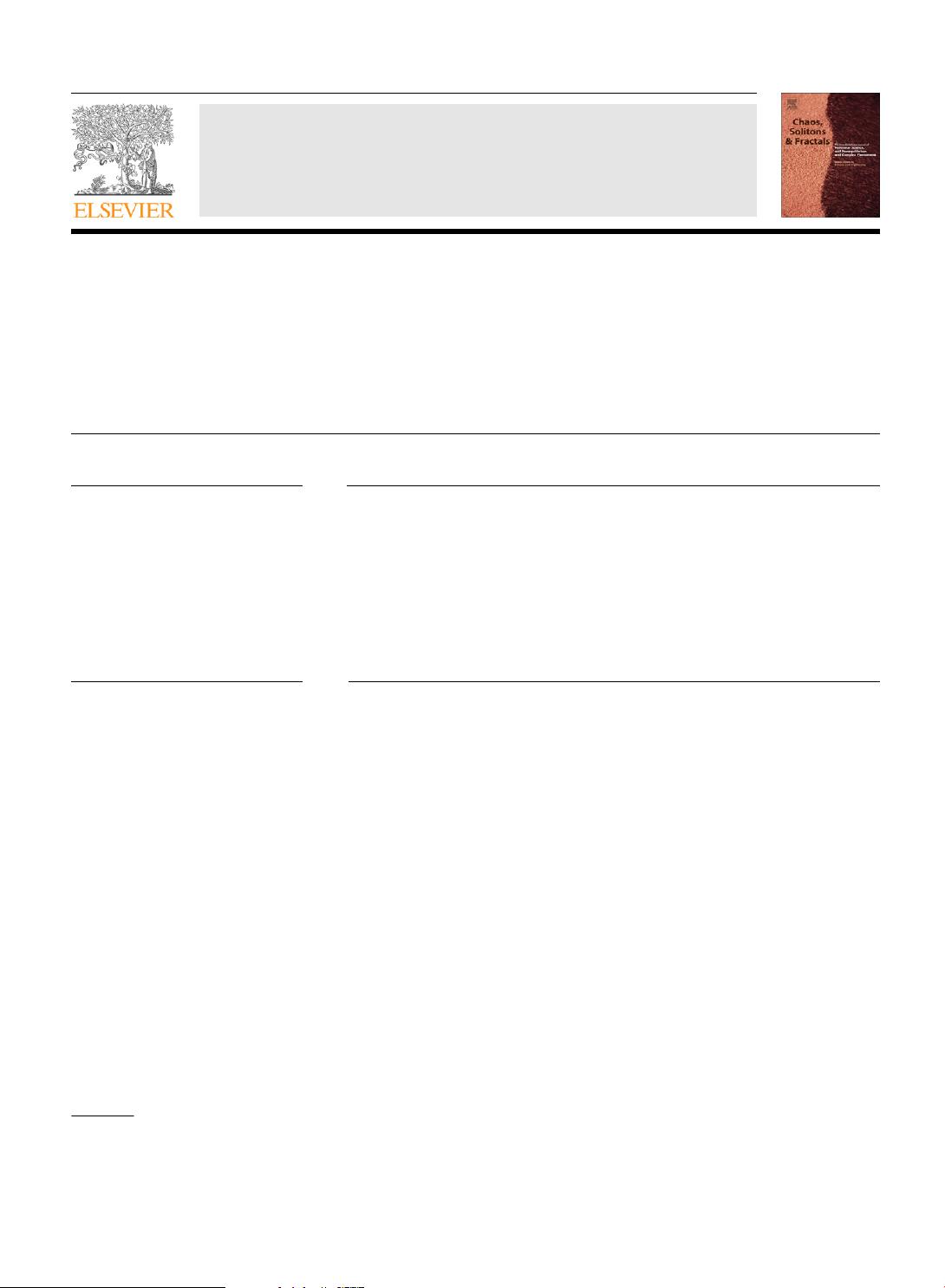
Robust anti-synchronization of uncertain chaotic systems based
on multiple-kernel least squares support vector machine modeling
Qiang Chen
a,
⇑
, Xuemei Ren
a
, Jing Na
b
a
School of Automation, Beijing Institute of Technology, Beijing 100081, PR China
b
Faculty of Mechanical and Electrical Engineering, Kunming University of Science and Technology, Kunming 650093, PR China
article info
Article history:
Received 14 October 2010
Accepted 1 September 2011
Available online 5 October 2011
abstract
In this paper, we propose a robust anti-synchronization scheme based on multiple-kernel
least squares support vector machine (MK-LSSVM) modeling for two uncertain chaotic sys-
tems. The multiple-kernel regression, which is a linear combination of basic kernels, is
designed to approximate system uncertainties by constructing a multiple-kernel Lagrangian
function and computing the corresponding regression parameters. Then, a robust feedback
control based on MK-LSSVM modeling is presented and an improved update law is employed
to estimate the unknown bound of the approximation error. The proposed control scheme
can guarantee the asymptotic convergence of the anti-synchronization errors in the presence
of system uncertainties and external disturbances. Numerical examples are provided to
show the effectiveness of the proposed method.
Crown Copyright Ó 2011 Published by Elsevier Ltd. All rights reserved.
1. Introduction
Over the past decades, chaos synchronization and anti-
synchronization have been intensively studied in various
fields [1–8]. Anti-synchronization (AS) is an interesting
phenomenon and the main feature is that the states of
response system own the same amplitude but opposite
signs as those of drive system. Recently, AS phenomena
have been observed experimentally in salt-water oscilla-
tors [9], semiconductor lasers [10–12] and so on. Various
methods have been proposed to anti-synchronize chaotic
systems, such as active control [13], adaptive control
[14,15], backstepping technique [16], and H
1
control
[17]. All of those schemes aforementioned are able to
achieve stable anti-synchronization for chaotic systems
with fully known or unknown parameters. However, when
model uncertainties and external disturbances are
included in the control system, most of them may not work
efficiently. Thus, the robust anti-synchronization problem
for chaotic systems in the presence of model uncertainties
and external disturbances still remains challenging.
In order to deal with model uncertainties in nonlinear
systems, many intelligent modeling approaches, such as
fuzzy systems, neural networks, support vector machines
(SVMs), etc., were investigated [18–23]. Compared with fuz-
zy systems and neural networks, support vector machines
own better generalization ability and avoid the over-fitting
problem according to the structural risk minimization
(SRM) principle. Recently, the least squares support vector
machine (LSSVM) was proposed and widely used for the
classification and regression problems of dynamic systems
[21–23]. An essential ingredient of the LSSVM is the kernel
design. In principle, the kernel can be chosen by standard
model selection methods such as cross validation [24,25],
and single kernel has been widely used. Multiple kernel
learning (MKL) is capable of optimizing kernel weights,
and generalizing feature selection to kernel selection by
enforcing sparse coefficients. Recently, MKL has been pro-
ven to be useful to improve the classifying or predicting per-
formance of support vector machines (SVMs) [26–28].
However, to the best of authors’ knowledge, there have been
few results published on the controller design based on mul-
tiple kernel modeling.
In this paper, a multiple-kernel least squares support
vector machine (MK-LSSVM) modeling based robust
anti-synchronization scheme is proposed for two chaotic
0960-0779/$ - see front matter Crown Copyright Ó 2011 Published by Elsevier Ltd. All rights reserved.
doi:10.1016/j.chaos.2011.09.001
⇑
Corresponding author. Tel.: +86 10 68914506.
E-mail address: chenqiang8404@yahoo.com.cn (Q. Chen).
Chaos, Solitons & Fractals 44 (2011) 1080–1088
Contents lists available at SciVerse ScienceDirect
Chaos, Solitons & Fractals
Nonlinear Science, and Nonequilibrium and Complex Phenomena
journal homepage: www.elsevier.com/locate/chaos