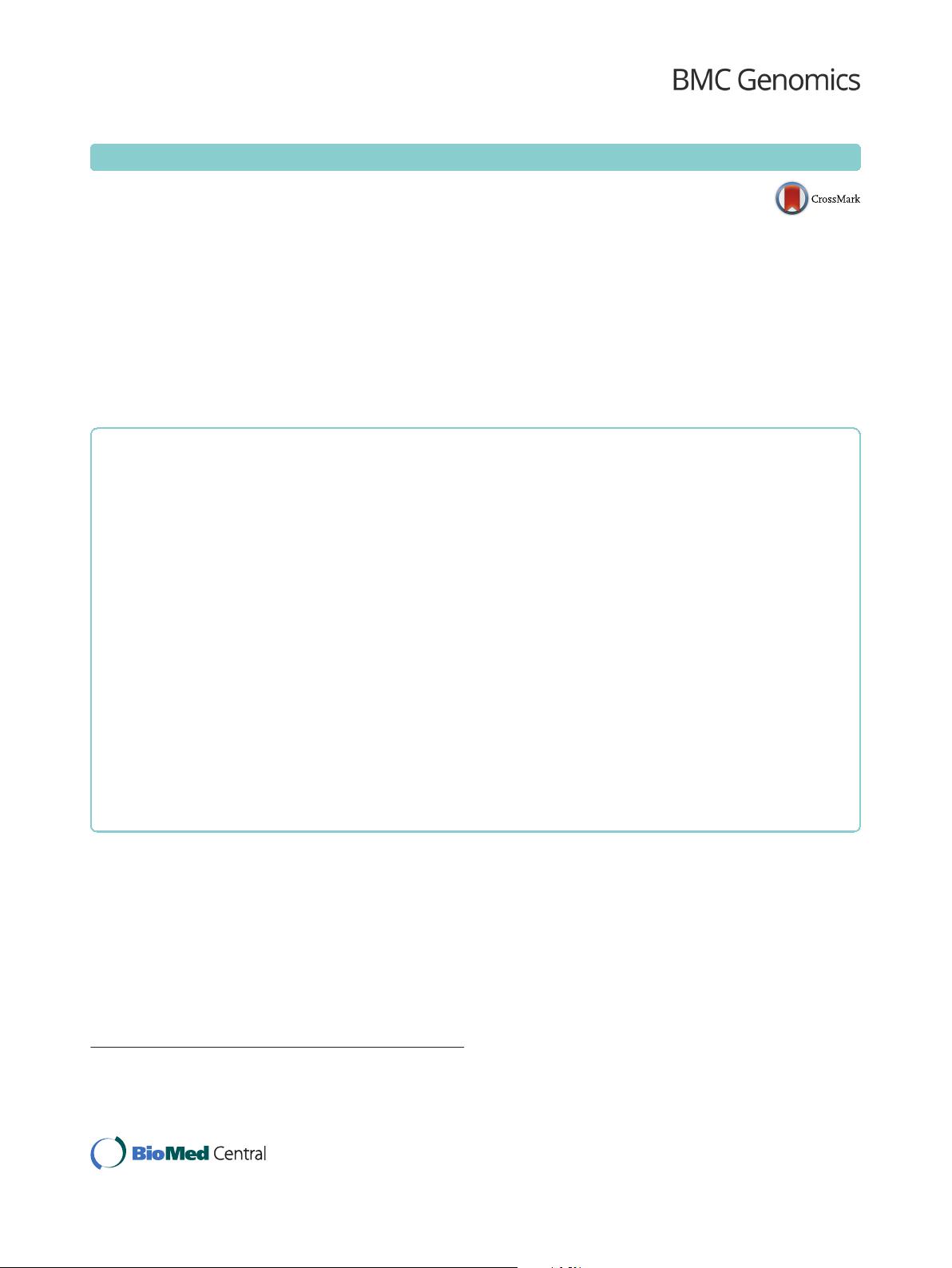
M ETHODOLOGY AR TICLE Open Access
Fast dimension reduction and integrative
clustering of multi-omics data using low-
rank approximation: application to cancer
molecular classification
Dingming Wu
1
, Dongfang Wang
1
, Michael Q. Zhang
1,2*
and Jin Gu
1*
Abstract
Background: One major goal of large-scale cancer omics study is to identify molecular subtypes for more accurat e
cancer diagnoses and treatments. To deal with high-dimensional cancer multi-omics data, a promising strategy is to
find an effective low-dimensional subspace of the original data and then cluster cancer samples in the reduced
subspace. However, due to data-type diversity and big data volume, few methods can integrative and efficiently
find the principal low-dimensional manifold of the high-dimensional cancer multi-omics data.
Results: In this study, we proposed a novel low-rank approximation based integrative probabilistic model to fast
find the shared principal subspace across multiple data types: the convexity of the low-rank regularized likelihood
function of the probabilistic model ensures efficient and stable model fitting. Candidate molecular subtypes can be
identified by unsupervised clustering hundreds of cancer samples in the reduced low-dimensional subspace. On
testing datasets, our method LRAcluster (low-rank approximation based multi-omics data clustering) runs much
faster with better clustering performances than the existing method. Then, we applied LRAcluster on large-scale
cancer multi-omics data from TCGA. The pan-cancer analysis results show that the cancers of different tissue origins
are generally grouped as independent clusters, except squamous-like carcinomas. While the single cancer type
analysis suggests that the omics data have different subtyping abilities for different cancer types.
Conclusions: LRAcluster is a very useful method for fast dimension reduction and unsupervised clustering of large-
scale multi-omics data. LRAcluster is implemented in R and freely available via http://bioinfo.au.tsinghua.edu.cn/
software/lracluster/.
Keywords: Mutli-omics, Cancer, Low-rank approximation, Clustering, Dimension reduction, Algorithm
Background
Cancer is a large family of lethal diseases which are kill-
ing millions of lives each year [1, 2]. Highly genetic het-
erogeneity makes it hard to develop general and effective
treatments against cancer [3, 4]. One of the major goal
of cancer multi-omics study is to disc over possible can-
cer subtypes using molecule-level signatures, which can
be used for more accurate diagnoses and treatments
[5–8]. Several international collaborated projects, such
as TCGA [9], ICGC [10], and CCLE [11] generated
tons of cancer multi-omics data. However, we still
face several challenge s for analyzing such large-sca le
cancer multi-omics data: 1) n eed to handle different
data types of different platforms at the same time,
such as count ba sed data of sequencing, continuous
data of microarray and binary data of g enetic varia-
tions; 2) the data dimension (the number of the mo-
lecular features) is much higher than the sample
number; and 3) the big data volumes require efficient
and robust computational algorithms.
The molecules involved in the same biological pro-
cesses are usually highly correlated. It is commonly
* Correspondence: michael.zhang@utdallas.edu; jgu@tsinghua.edu.cn
1
MOE Key Laboratory of Bioinformatics, TNLIST Bioinformatics Division &
Center for Synthetic and Systems Biology, Department of Automation,
Tsinghua University, Beijing 100084, China
Full list of author information is available at the end of the article
© 2015 Wu et al. Open Access This article is distributed under the terms of the Creative Commons Attribution 4.0
International License (http://creativecommons.org/licenses/by/4.0/), which permits unrestricted use, distribution, and
reproduction in any medium, provided you give appropriate credit to the original author(s) and the source, provide a link to
the Creative Commons license, and indicate if changes were made. The Creative Commons Public Domain Dedication waiver
(http://creativecommons.org/publicdomain/zero/1.0/) applies to the data made available in this article, unless otherwise stated.
Wu et al. BMC Genomics (2015) 16:1022
DOI 10.1186/s12864-015-2223-8