没有合适的资源?快使用搜索试试~ 我知道了~
首页利用Spark构建可扩展的机器学习应用
"《机器学习与Spark》是一本专为数据驱动业务提供强大支持的英文书籍,由Nick Pentreath撰写,由BIRMINGHAM-MUMBAI出版。本书旨在帮助读者利用Apache Spark构建可扩展的机器学习应用,使之成为现代商业环境中不可或缺的一部分。Spark是一个开源的大数据处理框架,以其高效的数据处理能力和内存计算能力在大数据分析领域中占据重要地位。 作者通过深入浅出的方式,介绍了如何利用Spark的分布式计算能力加速机器学习算法的执行,包括但不限于分类、聚类、回归和深度学习等核心机器学习技术。书中不仅涵盖了理论概念,还提供了大量的实践案例和代码示例,使读者能够在实际项目中快速理解和应用这些技术。 版权方面,这本书受2015年Packt Publishing所有,未经事先书面许可,禁止任何形式的复制、存储或传输,除非用于学术引用。尽管作者和出版社努力确保信息的准确性,但书中提供的信息是按“现状”销售,不提供任何形式的明示或默示担保,不会对因本书内容导致或声称的任何直接或间接损失承担责任。 《机器学习与Spark》的首次出版日期为2015年2月,反映了当时的技术发展水平。该书是Spark用户和机器学习爱好者的重要参考资料,对于希望将Spark技术与机器学习相结合以提升业务分析能力的读者来说,是一本不可多得的实用指南。通过阅读这本书,读者不仅能掌握Spark的基础知识,还能掌握如何在实际场景中优化和部署机器学习模型,从而推动企业向数据驱动决策转变。"
资源详情
资源推荐
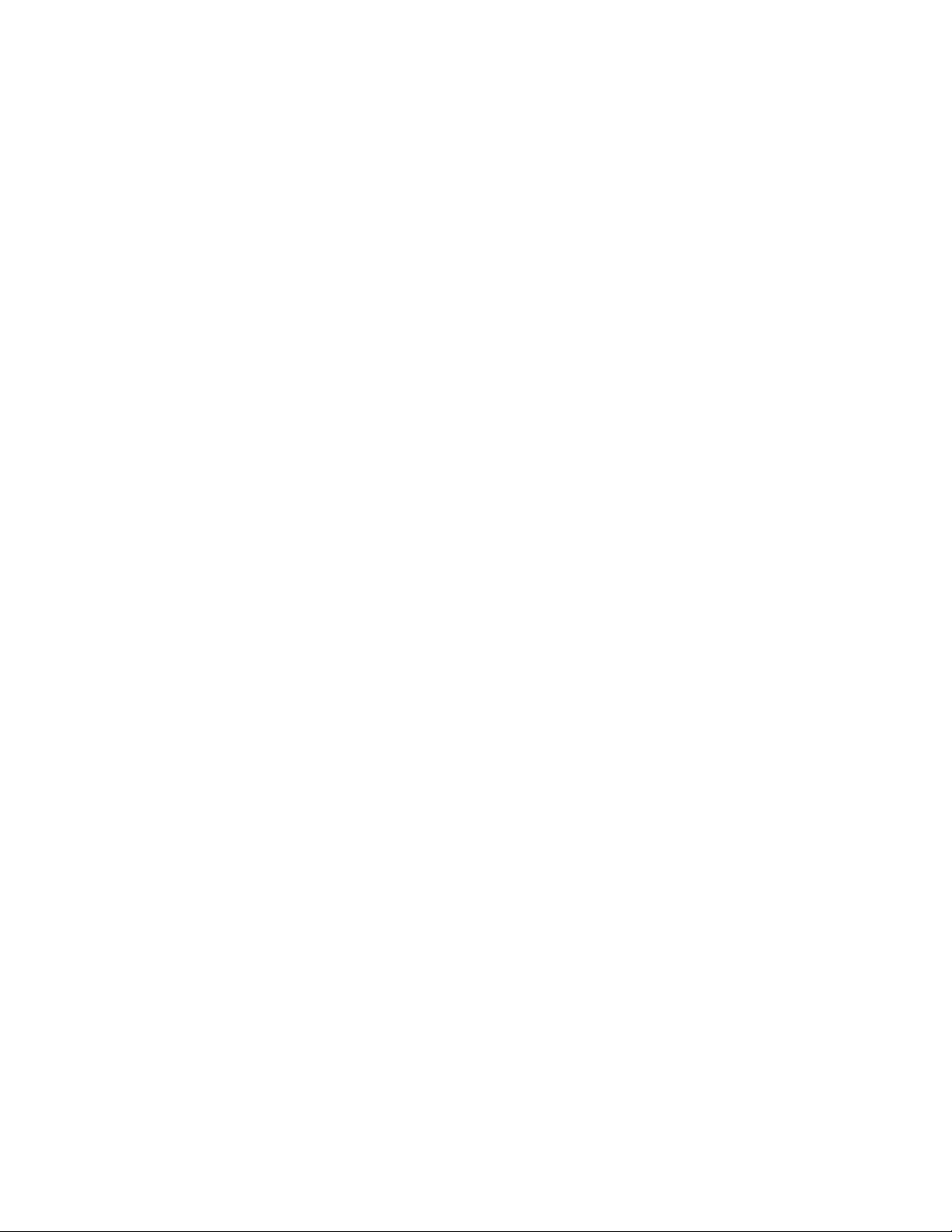
Preface
In recent years, the volume of data being collected, stored, and analyzed has
exploded, in particular in relation to the activity on the Web and mobile devices, as
well as data from the physical world collected via sensor networks. While previously
large-scale data storage, processing, analysis, and modeling was the domain of the
largest institutions such as Google, Yahoo!, Facebook, and Twitter, increasingly,
many organizations are being faced with the challenge of how to handle a massive
amount of data.
When faced with this quantity of data and the common requirement to utilize it in
real time, human-powered systems quickly become infeasible. This has led to a rise
in the so-called big data and machine learning systems that learn from this data to
make automated decisions.
In answer to the challenge of dealing with ever larger-scale data without any
prohibitive cost, new open source technologies emerged at companies such as
Google, Yahoo!, Amazon, and Facebook, which aimed at making it easier to handle
massive data volumes by distributing data storage and computation across a cluster
of computers.
The most widespread of these is Apache Hadoop, which made it signicantly easier
and cheaper to both store large amounts of data (via the Hadoop Distributed File
System, or HDFS) and run computations on this data (via Hadoop MapReduce,
a framework to perform computation tasks in parallel across many nodes in a
computer cluster).
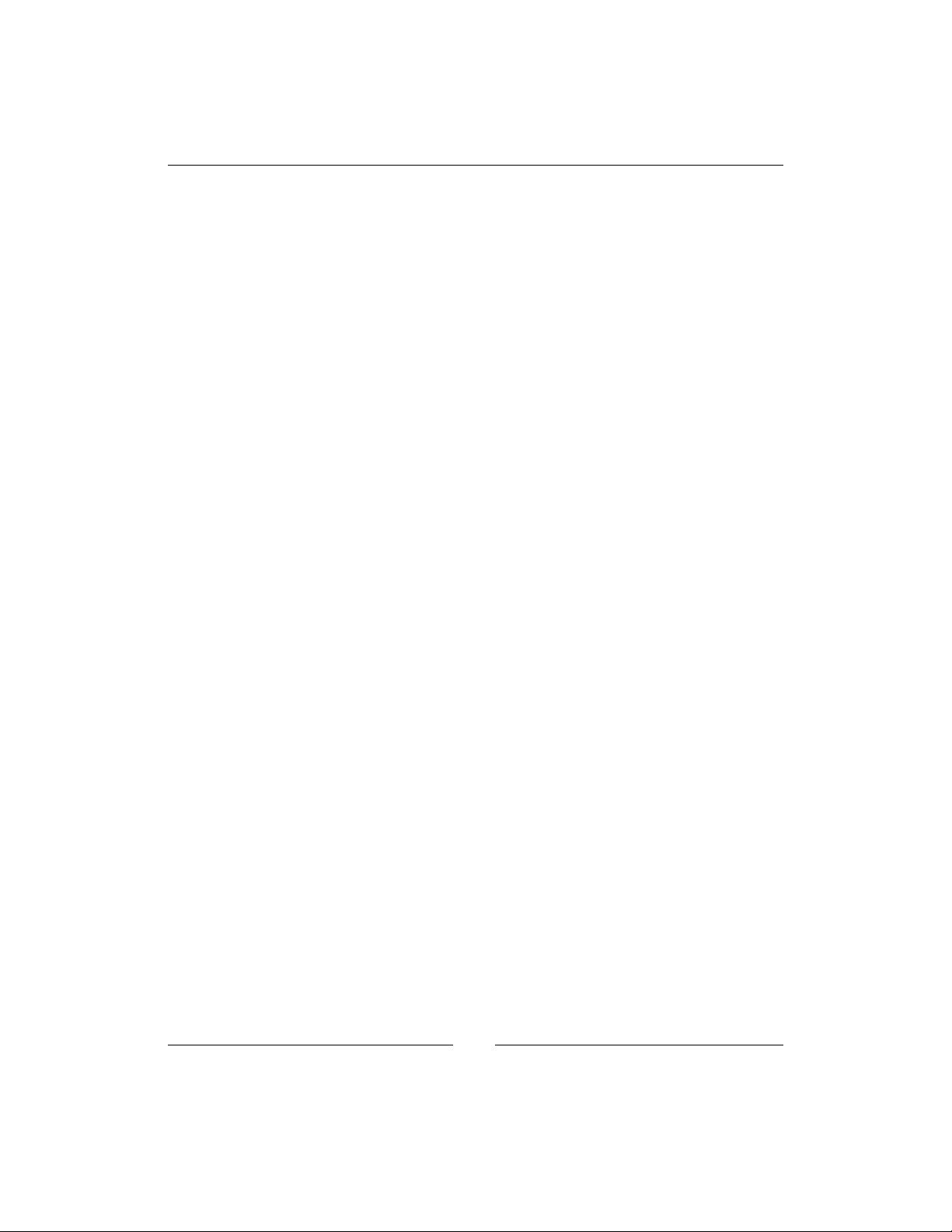
Preface
[ 2 ]
However, MapReduce has some important shortcomings, including high overheads
to launch each job and reliance on storing intermediate data and results of the
computation to disk, both of which make Hadoop relatively ill-suited for use cases of
an iterative or low-latency nature. Apache Spark is a new framework for distributed
computing that is designed from the ground up to be optimized for low-latency
tasks and to store intermediate data and results in memory, thus addressing some of
the major drawbacks of the Hadoop framework. Spark provides a clean, functional,
and easy-to-understand API to write applications and is fully compatible with the
Hadoop ecosystem.
Furthermore, Spark provides native APIs in Scala, Java, and Python. The Scala and
Python APIs allow all the benets of the Scala or Python language, respectively,
to be used directly in Spark applications, including using the relevant interpreter
for real-time, interactive exploration. Spark itself now provides a toolkit (called
MLlib) of distributed machine learning and data mining models that is under heavy
development and already contains high-quality, scalable, and efcient algorithms for
many common machine learning tasks, some of which we will delve into in this book.
Applying machine learning techniques to massive datasets is challenging, primarily
because most well-known machine learning algorithms are not designed for parallel
architectures. In many cases, designing such algorithms is not an easy task. The
nature of machine learning models is generally iterative, hence the strong appeal
of Spark for this use case. While there are many competing frameworks for parallel
computing, Spark is one of the few that combines speed, scalability, in-memory
processing, and fault tolerance with ease of programming and a exible, expressive,
and powerful API design.
Throughout this book, we will focus on real-world applications of machine learning
technology. While we may briey delve into some theoretical aspects of machine
learning algorithms, the book will generally take a practical, applied approach with
a focus on using examples and code to illustrate how to effectively use the features
of Spark and MLlib, as well as other well-known and freely available packages for
machine learning and data analysis, to create a useful machine learning system.
What this book covers
Chapter 1, Getting Up and Running with Spark, shows how to install and set up a local
development environment for the Spark framework as well as how to create a Spark
cluster in the cloud using Amazon EC2. The Spark programming model and API will
be introduced, and a simple Spark application will be created using each of Scala,
Java, and Python.
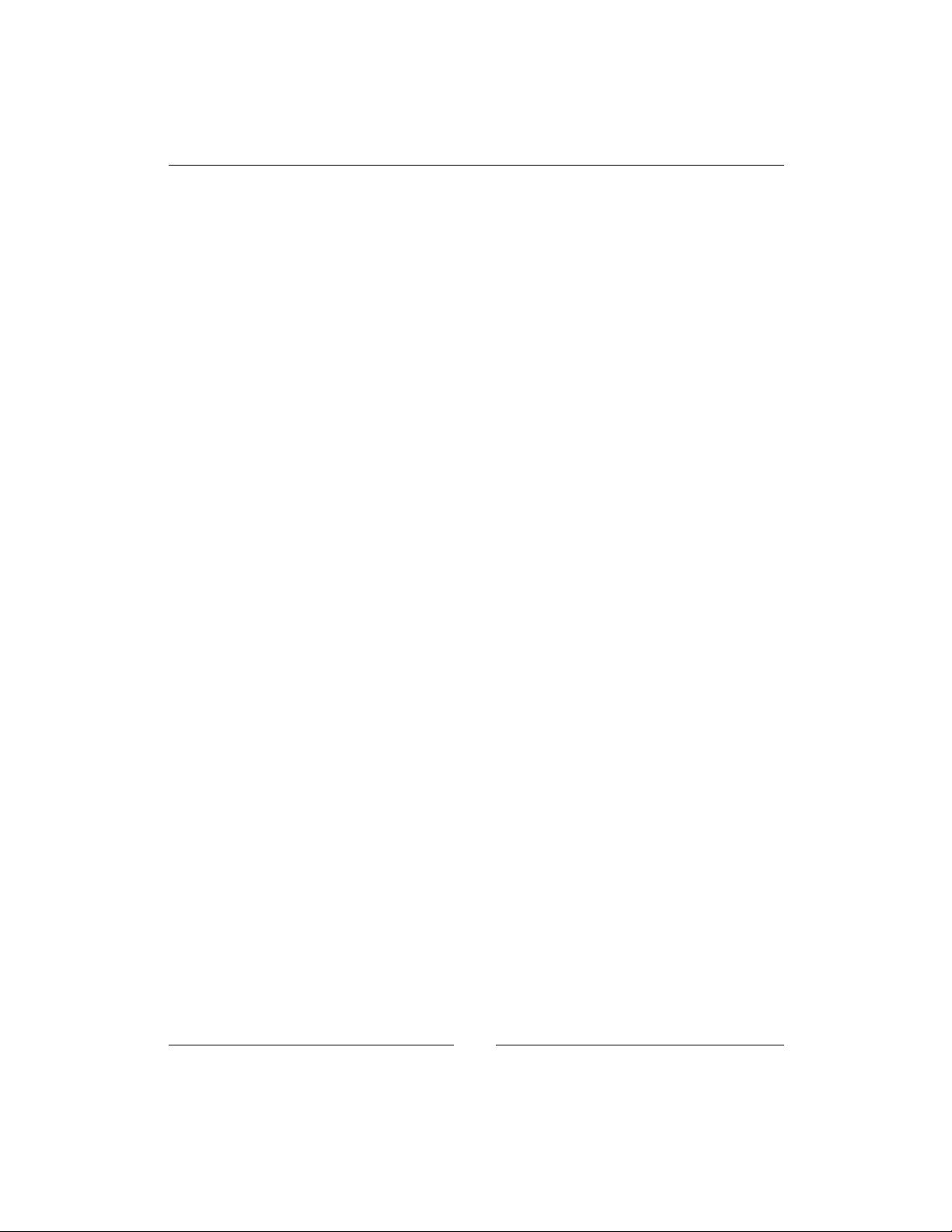
Preface
[ 3 ]
Chapter 2, Designing a Machine Learning System, presents an example of a real-world
use case for a machine learning system. We will design a high-level architecture for
an intelligent system in Spark based on this illustrative use case.
Chapter 3, Obtaining, Processing, and Preparing Data with Spark, details how to go about
obtaining data for use in a machine learning system, in particular from various freely
and publicly available sources. We will learn how to process, clean, and transform
the raw data into features that may be used in machine learning models, using
available tools, libraries, and Spark's functionality.
Chapter 4, Building a Recommendation Engine with Spark, deals with creating a
recommendation model based on the collaborative ltering approach. This model
will be used to recommend items to a given user as well as create lists of items
that are similar to a given item. Standard metrics to evaluate the performance of a
recommendation model will be covered here.
Chapter 5, Building a Classication Model with Spark, details how to create a model
for binary classication as well as how to utilize standard performance-evaluation
metrics for classication tasks.
Chapter 6, Building a Regression Model with Spark, shows how to create a model
for regression, extending the classication model created in Chapter 5, Building a
Classication Model with Spark. Evaluation metrics for the performance of regression
models will be detailed here.
Chapter 7, Building a Clustering Model with Spark, explores how to create a clustering
model as well as how to use related evaluation methodologies. You will learn how to
analyze and visualize the clusters generated.
Chapter 8, Dimensionality Reduction with Spark, takes us through methods to extract
the underlying structure from and reduce the dimensionality of our data. You will
learn some common dimensionality-reduction techniques and how to apply and
analyze them, as well as how to use the resulting data representation as input to
another machine learning model.
Chapter 9, Advanced Text Processing with Spark, introduces approaches to deal with
large-scale text data, including techniques for feature extraction from text and
dealing with the very high-dimensional features typical in text data.
Chapter 10, Real-time Machine Learning with Spark Streaming, provides an overview
of Spark Streaming and how it ts in with the online and incremental learning
approaches to apply machine learning on data streams.
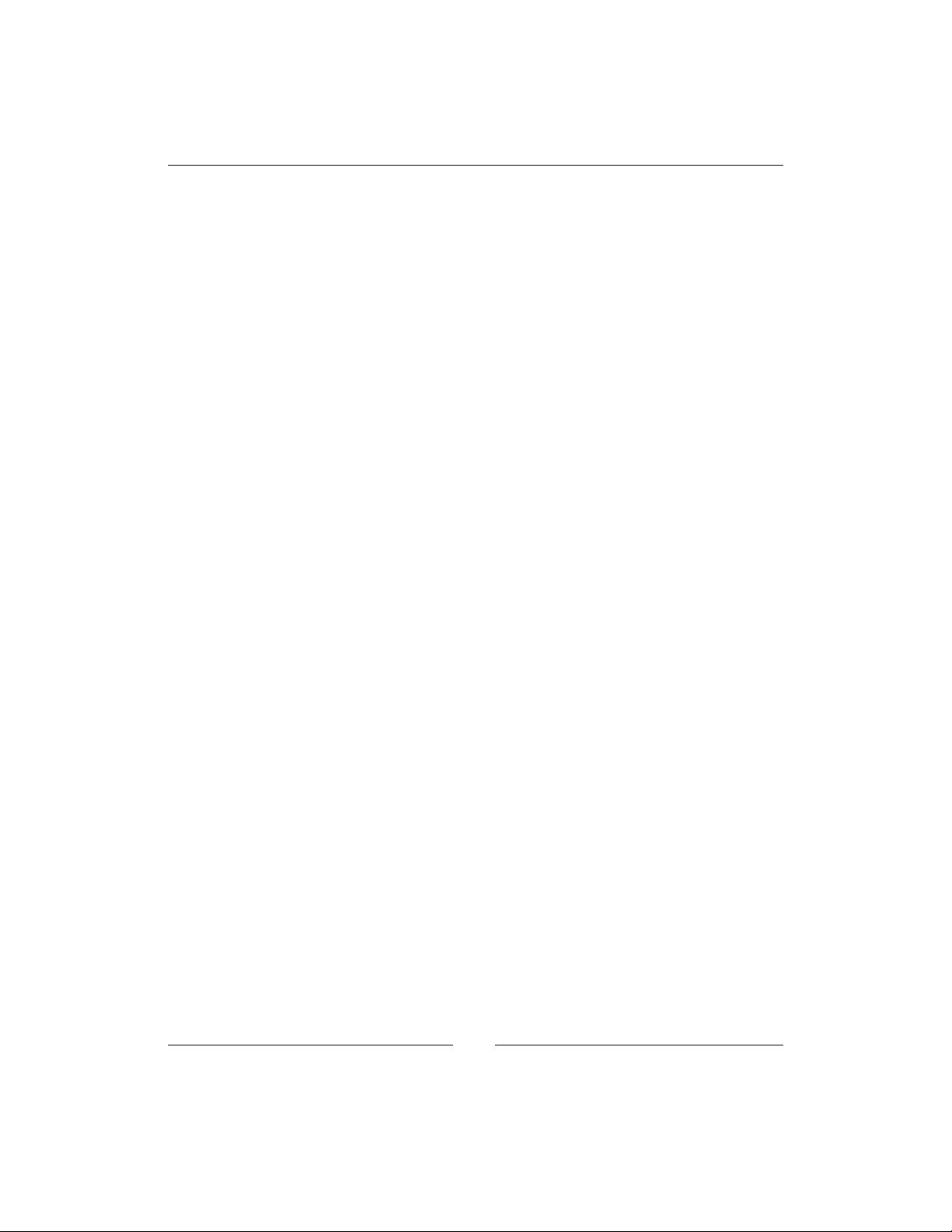
Preface
[ 4 ]
What you need for this book
Throughout this book, we assume that you have some basic experience with
programming in Scala, Java, or Python and have some basic knowledge of
machine learning, statistics, and data analysis.
Who this book is for
This book is aimed at entry-level to intermediate data scientists, data analysts, software
engineers, and practitioners involved in machine learning or data mining with an
interest in large-scale machine learning approaches, but who are not necessarily
familiar with Spark. You may have some experience of statistics or machine learning
software (perhaps including MATLAB, scikit-learn, Mahout, R, Weka, and so on) or
distributed systems (perhaps including some exposure to Hadoop).
Conventions
In this book, you will nd a number of styles of text that distinguish between
different kinds of information. Here are some examples of these styles, and an
explanation of their meaning.
Code words in text, database table names, folder names, lenames, le extensions,
pathnames, dummy URLs, user input, and Twitter handles are shown as follows:
"Spark places user scripts to run Spark in the
bin directory."
A block of code is set as follows:
val conf = new SparkConf()
.setAppName("Test Spark App")
.setMaster("local[4]")
val sc = new SparkContext(conf)
Any command-line input or output is written as follows:
>tar xfvz spark-1.2.0-bin-hadoop2.4.tgz
>cd spark-1.2.0-bin-hadoop2.4
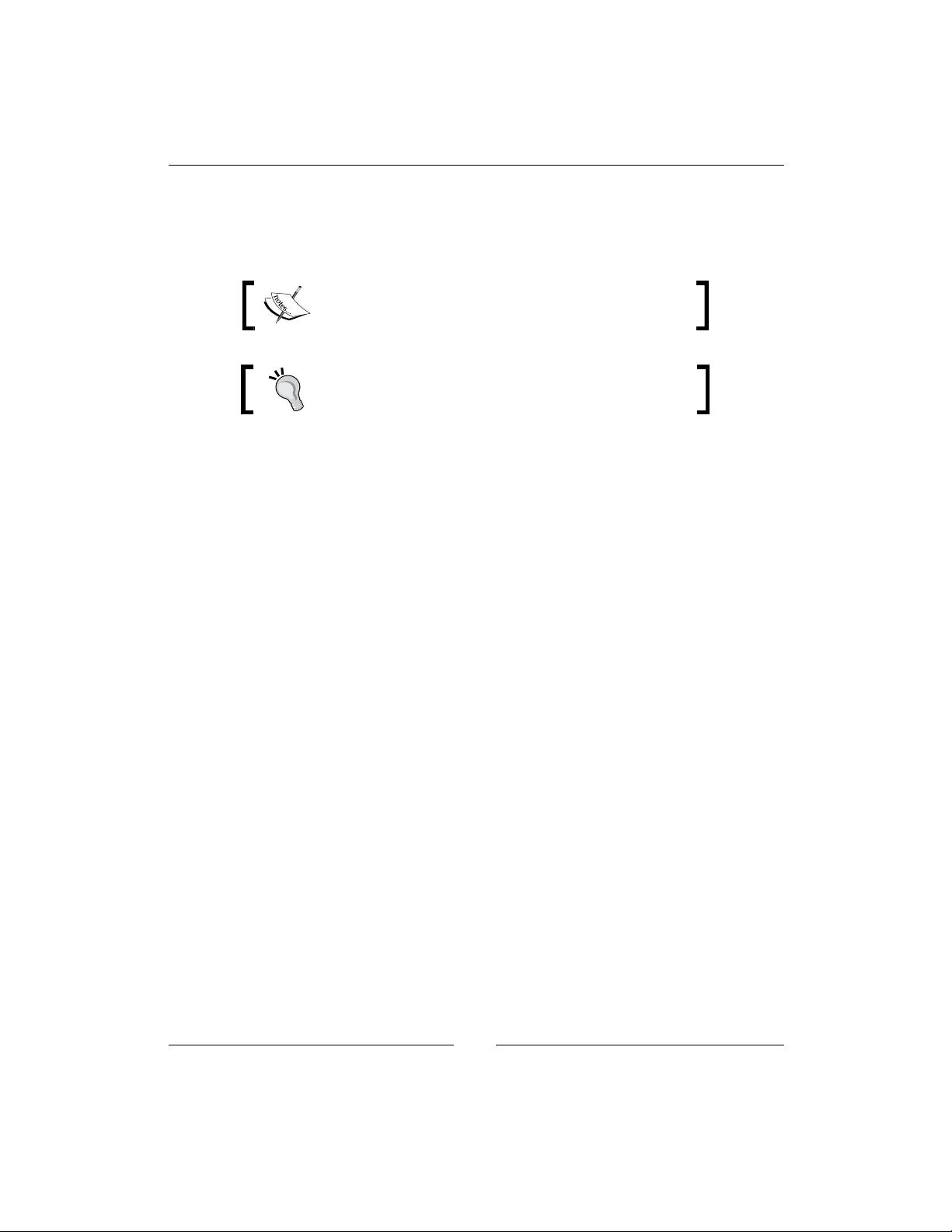
Preface
[ 5 ]
New terms and important words are shown in bold. Words that you see on the
screen, in menus or dialog boxes for example, appear in the text like this: "These can
be obtained from the AWS homepage by clicking Account | Security Credentials |
Access Credentials."
Warnings or important notes appear in a box like this.
Tips and tricks appear like this.
Reader feedback
Feedback from our readers is always welcome. Let us know what you think about
this book—what you liked or may have disliked. Reader feedback is important for
us to develop titles that you really get the most out of.
To send us general feedback, simply send an e-mail to
feedback@packtpub.com,
and mention the book title through the subject of your message.
If there is a topic that you have expertise in and you are interested in either writing
or contributing to a book, see our author guide on
www.packtpub.com/authors.
Customer support
Now that you are the proud owner of a Packt book, we have a number of things to
help you to get the most from your purchase.
Downloading the example code
You can download the example code les for all Packt books you have purchased
from your account at http://www.packtpub.com. If you purchased this book
elsewhere, you can visit http://www.packtpub.com/support and register to have
the les e-mailed directly to you.
剩余337页未读,继续阅读
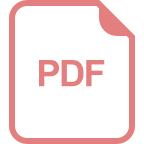










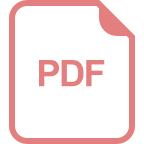
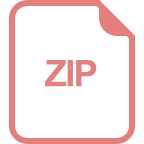
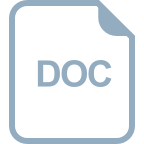
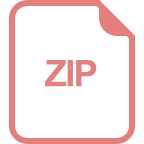
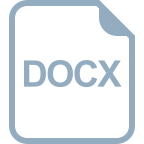
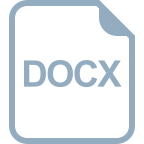
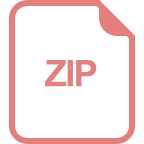

wjj_cadn
- 粉丝: 2
- 资源: 9
上传资源 快速赚钱
我的内容管理 展开
我的资源 快来上传第一个资源
我的收益
登录查看自己的收益我的积分 登录查看自己的积分
我的C币 登录后查看C币余额
我的收藏
我的下载
下载帮助

最新资源
- 多模态联合稀疏表示在视频目标跟踪中的应用
- Kubernetes资源管控与Gardener开源软件实践解析
- MPI集群监控与负载平衡策略
- 自动化PHP安全漏洞检测:静态代码分析与数据流方法
- 青苔数据CEO程永:技术生态与阿里云开放创新
- 制造业转型: HyperX引领企业上云策略
- 赵维五分享:航空工业电子采购上云实战与运维策略
- 单片机控制的LED点阵显示屏设计及其实现
- 驻云科技李俊涛:AI驱动的云上服务新趋势与挑战
- 6LoWPAN物联网边界路由器:设计与实现
- 猩便利工程师仲小玉:Terraform云资源管理最佳实践与团队协作
- 类差分度改进的互信息特征选择提升文本分类性能
- VERITAS与阿里云合作的混合云转型与数据保护方案
- 云制造中的生产线仿真模型设计与虚拟化研究
- 汪洋在PostgresChina2018分享:高可用 PostgreSQL 工具与架构设计
- 2018 PostgresChina大会:阿里云时空引擎Ganos在PostgreSQL中的创新应用与多模型存储
资源上传下载、课程学习等过程中有任何疑问或建议,欢迎提出宝贵意见哦~我们会及时处理!
点击此处反馈


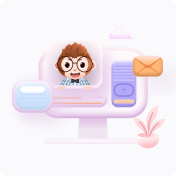
安全验证
文档复制为VIP权益,开通VIP直接复制
