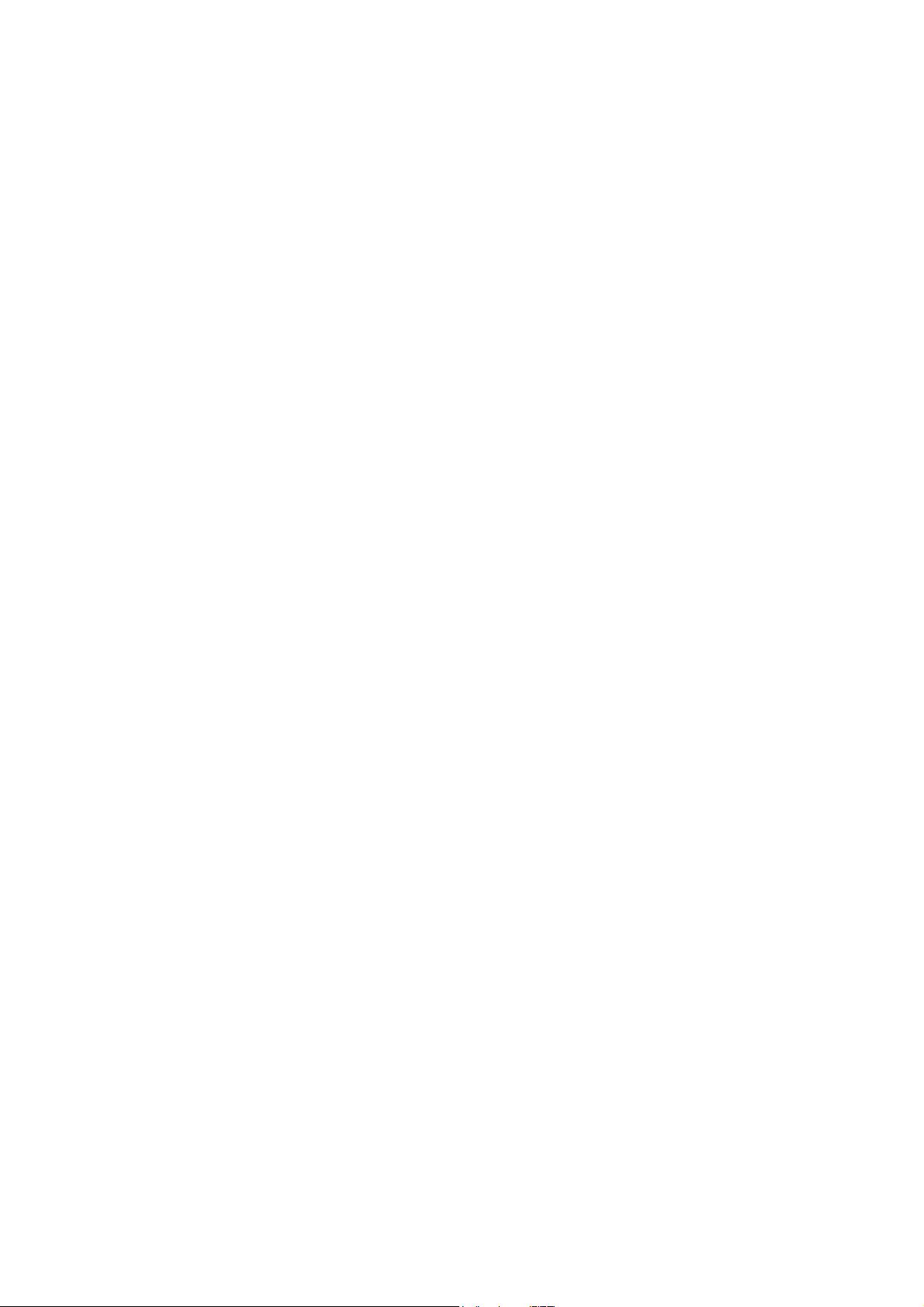
Measuring Photoplethysmogram-Based Stress-Induced Vascular
Response Index to Assess Cognitive Load and Stress
Yongqiang Lyu
, Xiaomin Luo
, Jun Zhou
, Chun Yu
, Congcong Miao
, Tong Wang
,
Yuanchun Shi
1
, Ken-ichi Kameyama
3
1
TNLIST, Research Institute of Information Technology, Tsinghua University, Beijing China
2
BGI (Wuhan) Translational Research Center, Wuhan, China
3
Interactive Media Lab., Corporate R&D Center, Toshiba Corp., Tokyo, Japan
luyq@tsinghua.edu.cn, luoxm@ luoxm@genomics.org.cn, kenichi.kameyama@toshiba.co.jp
ABSTRACT
Quantitative assessment for cognitive load and mental
stress is very important in optimizing human-computer
system designs to improve performance and efficiency.
Traditional physiological measures, such as heart rate
variation (HRV), blood pressure and electrodermal activity
(EDA), are widely used but still have limitations in
sensitivity, reliability and usability. In this study, we
propose a novel photoplethysmogram-based stress induced
vascular index (sVRI) to measure cognitive load and stress.
We also provide the basic methodology and detailed
algorithm framework. We employed a classic experiment
with three levels of task difficulty and three stages of
testing period to verify the new measure. Compared with
the blood pressure, heart rate and HRV components
recorded simultaneously, the sVRI reached the same level
of significance on the effect of task difficulty/period as the
most significant other measure. Our findings showed
sVRI’s potential as a sensitive, reliable and usable
parameter.
Author Keywords
Cognitive Load; Mental Effort; Stress; Stress-Induced
Vascular Response Index (sVRI); Photoplethysmogram.
ACM Classification Keywords
H.1.2 [User/Machine Systems Subjects]: Human
information processing.
INTRODUCTION
Cognitive load refers to the load that performing a
particular task imposes on the human cognitive system [1].
It reflects the demand for both the psychological and
physiological resources of cognition, such as working
memory, the processing units for visual/spatial and
auditory/verbal information, and mental intent. Assessing
the cognitive load that a particular system or task imposes
on users is very important in human-computer interaction
(HCI) design, as the information on cognitive load can be
used to improve these systems [2]. Dynamic and real-time
assessment of cognitive load is particularly useful for
capturing the detailed changes subjects undergo during a
task, which supports a precise analysis as well as
improvement on design components [2-4] and the
collection of continuous psychophysiological data for smart
HCI applications [5-7].
In practice, the mental effort, which refers to the cognitive
resources actually allocated to accommodate a task [1], is
often used to reflect the actual cognitive load while
participants are working on a task. Mental effort is linked to
both cognitive capacity (cognitive resources available) and
autonomic intent (arousal). Due to variations in arousal, the
same person may perform differently on the same task at
different times. Cognitive load or mental effort causes
mental stress, which occurs when the task’s cognitive load
exceeds the subject’s cognitive capacity, or may also occur
when the subjects feel the cognitive load will exceed their
cognitive capacity [8]. Mental stress often accompanies the
cognitive process when subjects exert mental effort, e.g.
executing tasks. This stress may give rise to emotions like
anxiety, fatigue, and anger and has an influence on human
health [5, 6]. Stress measurement is also very important for
affective computing and health-related applications.
Researchers may employ subjective work/task load
questionnaires [9, 10] to assess cognitive load, or stress
state questionnaires [11] to assess the stress. Compared to
these subjective measures, objective measures, including
behavioral methods, such as behavior measurement and
behavioral performance measurement [4, 12, 13], and
physiological measures [14-23], such as heart rate variation,
blood pressure and skin conductivity, can assess cognitive
load and stress more sensitively and accurately.
Nevertheless, these measures still suffer from some notable
limitations in sensitivity, reliability and usability.
Researchers often need to combine multiple measures
together to improve the sensitivity and reliability of these
measures. However, combining measures in this way is not
Permission to make d
igital or hard copies of all or part of this work for
personal or classroom use is granted without fee provided that copies are
not made or distributed for profit or commercial advantage and that copies
bear this notice and the full citation on the first p
components of this work owned by others than ACM must be honored.
Abstracting with credit is permitted. To copy otherwise, or republish, to
post on servers or to redistribute to lists, requires prior specific permission
and/or a fee. Reques
t permissions from Permissions@acm.org.
CHI 2015, April 18 -
23 2015, Seoul, Republic of Korea
Copyright 2015 ACM 978-1-4503-3145-
http://dx.doi.org/10.1145/2702123.2702399