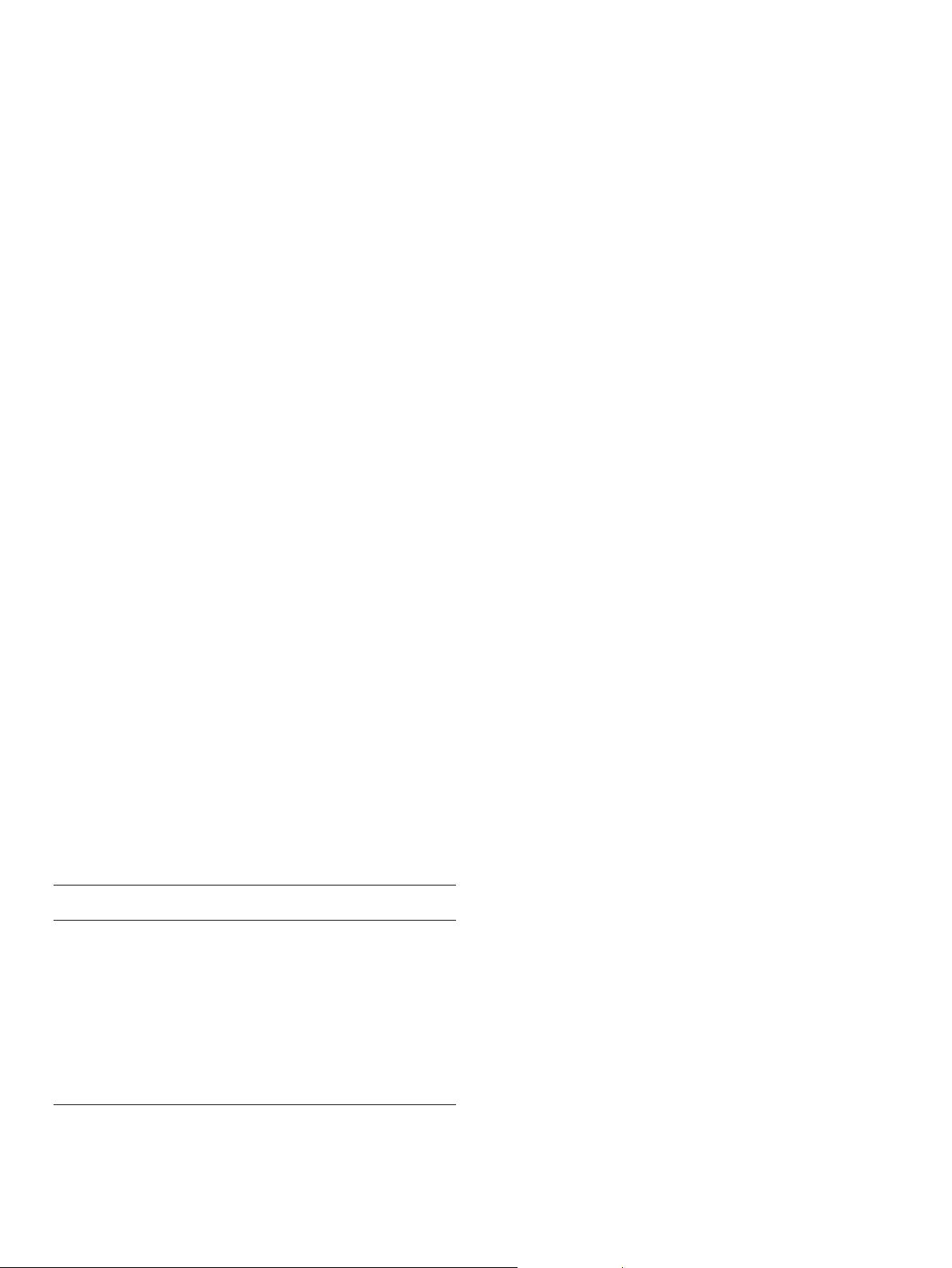
majority of the population residing within urban areas. The rapid
population rise has repercussions such as enhanced risks of climate
change effects, excessive use of energy resources, water scarcity, pol-
lution, loss of wildlife and agricultural land, and lack of employment
or underemployment among others. Due to heterogeneity in land
cover and sealing of soil surface, the SOC estimates within urban en-
vironments are unknown, or are difficult to measure. The massive
SOC losses from urban soils (Lal, 2003a,b), will require accurate SOC
estimation to make science driven decisions aimed at conserving
the soil and water resources (Platt, 1994).
2. Development of methods for SOC estimation
Laboratory methods such as the Walkley and Black (1934), and
the dry combustion (DC) (Kalembasa and Jenkinson, 1973; Nelson
and Sommers, 1982) have been the standard approaches for SOC de-
termination. The SOC pool can also be quantified in situ, for example
through the Inelastic Neutron Scattering (INS) method (Wielopolski
et al., 2011). However, determination of SOC using laboratory or
field based methods may be expensive and time consuming especially
for C inventory over large spatial extents. Remote sensing technology
is emerging as a promising tool for environmental and public sector
applications, given the availability of historical data, the reduction in
data cost, and increased resolution from satellite platforms (GOFC-
GOLD, 2012; Rowland et al., 2007). Remote sensing exploits the fact
that objects on the earth's surface reflect, absorb, and emit electromag-
netic radiation in a different way (i.e., each object has a specific spectral
response) depending on their molecular composition, texture, size and
shape. A major limitation in most remote sensors especially when used
to acquire information in-situ is that they provide only spectral re-
sponse of objects on the earth's surface. However, analytical spectral de-
vices (ASD) e.g., the FieldSpec exist which may be used to acquire
spectral reflectance of soils below the earth surface in the laboratory.
Remotely sensed data may be acquired using ground, aerial or
space based sensors. Ground based systems although applicable for
small area assessments are not only accurate, but have relatively
less atmospheric errors due to decreased atmospheric path length,
compared with aerial or satellite sensors which in turn are suitable
for regional mapping. Ground based sensors are useful for calibrating
airborne or satellite sensor data. Table 3 provides some examples of
the relative instrumentation cost of ground based sensors e.g., the
ASD FieldSPec (350–2500 nm) and CropScan 16 handheld multispec-
tral radiometer (MSR), airborne and satellite sensors, all of which
vary depending on the spatial, spectral or temporal resolution specifica-
tions (Ben-Dor et al., 2008; Chang et al., 2005; Reeves, 2010; Rossel et
al., 2009). Coarse spatial resolution satellite data are generally less ex-
pensive than higher resolution, exception include the moderate spatial
resolution satellite sensors such as Landsat which are currently free
(Roy et al., 2008).
Depending on the energy sources involved in the data acquisition, re-
mote sensing imaging instruments may be classified as active (i.e. emits
and detects own energy, to and from target), or passive (i.e. sun is the
energy source). The use of passive optical remotely sensed spectral
band data from the visible and near infra-red is being proposed to
characterize soil quality (Bricklemyer and Brown, 2010; Croft et al.,
2012; McCarty et al., 2002; Stevens et al., 2008). However, among the
principal limitations of individual spectral bands are that they are not
only highly correlated, but also vary with solar zenith, and topographic
slope (Holben and Justice, 1981; Huete, 1987; Huete and Tucker, 1991).
An alternative would be to use spectral indices which are neither highly
correlated nor influenced by solar zenith (
Haboudane et al., 2004; Huete
et al., 1994). Spectral indices are computed from a combination of
individual spectral bands from at least two different regions within the
electromagnetic spectrum.
The SOC, a proxy of soil quality may be measured in the laboratory
or field and upscaled to cover large spatial extents using aerial or sat-
ellite data (Fig. 1). This can be undertaken for example, by measuring
the spectral reflectance of surface and sub-surface soils in the labora-
tory, and then developing models that relate SOC concentration at
different depths with reflectance. These developed models can then
be applied directly on to the satellite data to extrapolate over large
spatial extents. Alternately, the temporal variability in crop yield may
also be a useful indicator of soil quality variability across space and time.
Validation of remotely sensed products with actual field measure-
ments may be conducted through statistical analysis, for example by
regression analysis or analysis of variance (ANOVA). If a large disagree-
ment exists between modeled estimates and actual measurements, the
algorithm or model is modified and re-applied to generate another SOC
estimate which is evaluated, and the process of model and product de-
velopment repeated until satisfactory results are achieved. The accuracy
of the final SOC values is noted for comparison, or reference. A discus-
sion of the methods applied to measure SOC an indicator of soil quality,
and limitations are provided in the next sub-section and summarized in
Table 4.
2.1. Laboratory methods
The Walkley and Black (1934) method is a chemical oxidation
procedure for measuring SOC concentration. Although the Walkley–
Black procedure is simple, rapid with minimal equipment needs, the
results may vary depending on the land uses, soil depth, and soil tex-
ture (De Vos et al., 2007). On the other hand, the dry combustion (DC)
instruments e.g., the Vario Max CN analyzer (Nelson and Sommers,
1996), give the C/N ratios, and determine the SOC concentration of
air-dried and sieved soils (e.g., through 2 mm diameter) at 900 °C. Al-
ternately, the weight loss on ignition method is a DC approach that
gravimetrically determines SOC from soil samples heated in a furnace
at 430 °C, for 24 h (Chatterjee et al., 2009). The Walkley–Black, and
the weight loss on ignition method provide SOC estimates that vary
widely with soil depths. Tivet et al. (2012) demonstrated that the
DC method provides less uncertainty in SOC estimates for different
land uses and depth compared with Walkley–Black, and proposed con-
version equations from Walkley–Black to DC.
Laboratory methods such as the DC compute SOC as mass fraction
by weight (g kg
− 1
)(Smith and Tabatabai, 2004a). For estimating the
spatial variation in SOC, it is important to initially determine the soil
Table 3
Estimated acquisition cost for remotely sensed data.
Ground sensor (spatial resolution depends
on height of sensor above ground)
Wavelength
range (nm)
Purchase price
for sensor(US $)
ASD Fieldspec Pro JR
a
350–2500 42,000–70,000
Apogee/StellarNet SPEC-PAR/NIR 350–950 3600
StellarNet EPP2000-NIR-InGaAs 580–1700 17,000
Cropscan 16 MSR 450–1750 3000
Airborne sensors
b
Spatial
resolution (m)
Imagery cost
(US $ km
− 2
)
Aerial photo (scanned, non rectified) 1 4000–5000
Aerial photo (orthorectified) 1 10,000–15,000
Satellite sensors
b
Very high resolution b 4 3000–10,000
High resolution passive optical b 20 120–400
Medium resolution optical 20–250 0.08
Coarse resolution passive optical >250 0
a
Analytical spectral device (ASD). Source: http://www.usu.edu/cpl/PDF/Comparison
%20of%20Spectrometers.pdf.
b
Modified from Hyde (2005), Rogan and Chen (2004),andhttp://land.umn.edu/
documents/FS3.pdf. Prices do not include pre-flight (e.g. transportation or GPS) costs
or post-flight processing (optical airborne and satellite sensors acquire data in spectral
bands between 400 and 2500 nm wavelength range).
80 V. de Paul Obade, R. Lal / Catena 104 (2013) 77–92