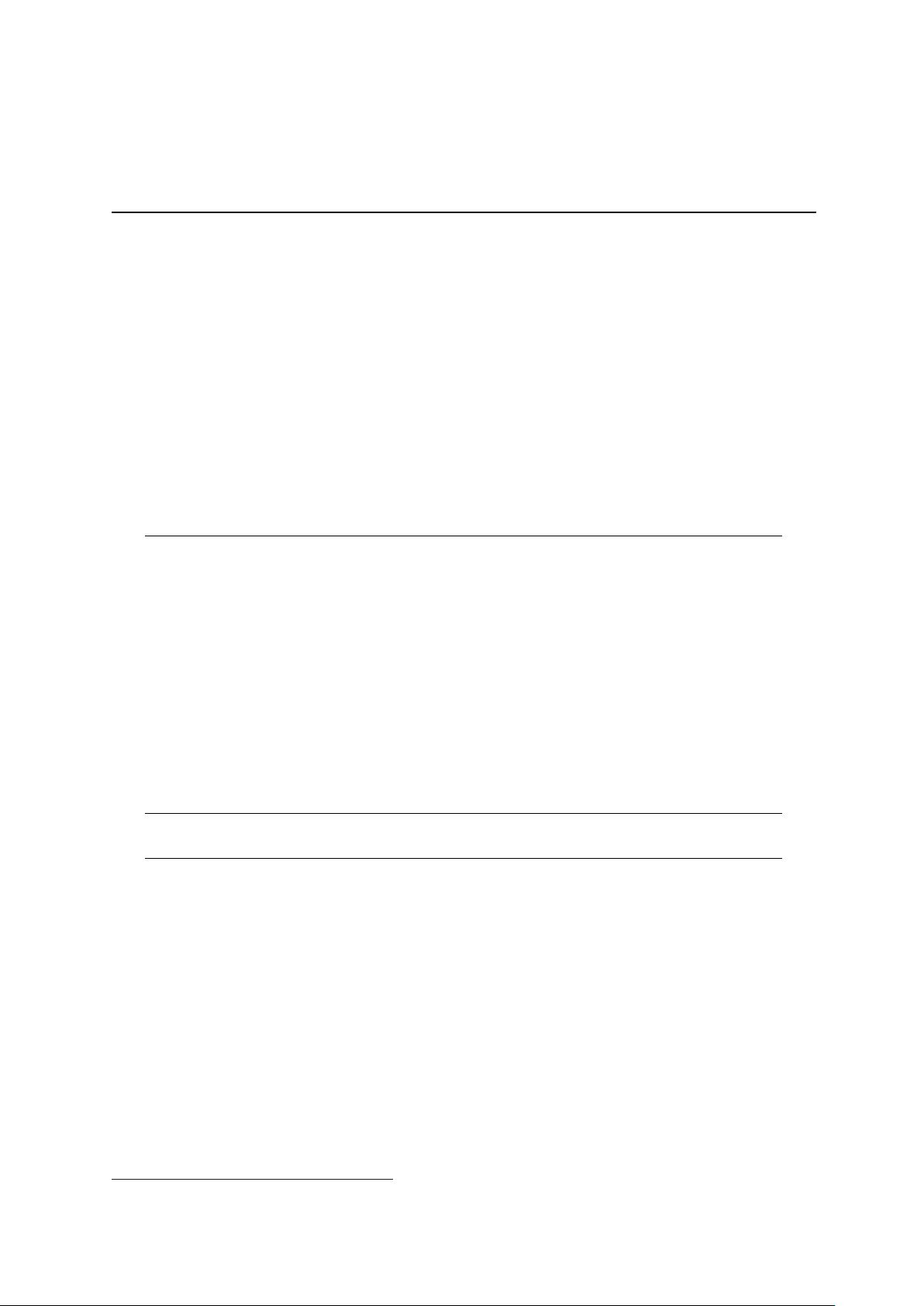
.
RESEARCH PAPERS
.
SCIENCE CHINA
Information Sciences
November 2010 Vol. 53 No. 11: 2343–2351
doi: 10.1007/s11432-010-4080-z
c
° Science China Press and Springer-Verlag Berlin Heidelberg 2010 info.scichina.com www.springerlink.com
CFAR assessment of covariance matrix estimators
for non-Gaussian clutter
HE You, JIAN Tao
∗
, SU Feng, QU ChangWen & PING DianFa
Research Institute of Information Fusion, Naval Aeronautical and Astronautical University,
Yantai 264001, China
Received November 14, 2009; accepted May 11, 2010; published online September 14, 2010
Abstract In the non-Gaussian clutter modeled as independent and identically distributed spherically invariant
random vectors, three estimators of sample covariance matrix (SCM), normalized sample covariance matrix
(NSCM) and the corresponding recursive estimator (NSCM-RE) are analyzed. Based on the uniform theorem,
three corresponding adaptive normalized matched filters (ANMF) are evaluated from the standpoint of constant
false alarm rate (CFAR) property. The theoretical results demonstrate that the SCM-ANMF is only CFAR
to the normalized clutter covariance matrix (NCCM); the NSCM-ANMF is only CFAR to the clutter power
level; and the NSCM-RE-ANMF with finite number of iterations is still not CFAR to the NCCM. To ensure
CFAR property of ANMF, an adaptive estimator (AE) is devised. Moreover, with AE as the initialization
matrix for the iterations, the AE-RE is proposed. With finite number of iterations, the corresponding AE-RE-
ANMF guarantees CFAR property to both of the NCCM and the clutter power level. Finally, the performance
assessment conducted by Monte Carlo simulation confirms the effectiveness of the proposed detectors.
Keywords non-Gaussian background, covariance matrix estimate, normalized matched filter, constant false
alarm rate, recursive estimate
Citation He Y, Jian T, Su F, et al. CFAR assessment of covariance matrix estimators for non-Gaussian clutter.
Sci China Inf Sci, 2010, 53: 2343–2351, doi: 10.1007/s11432-010-4080-z
1 Introduction
In the radar target adaptive detection applications, the unknown clutter covariance matrix usually needs
to be estimated [1], based on the data from the range cells adjacent to the cell under test. For the low
resolution radar, the clutter statistics can be modeled as Gaussian distribution well [2]. However, in
situations such as high-resolution radars or low grazing angles, the background clutter may no longer be
modeled accurately as a Gaussian random variable (RV) [3]. On-field measurements have shown that the
high-resolution radar system [4] receives non-Gaussian observations, which can be suitably modeled as a
spherically invariant random vector (SIRV) [5]. In most radar applications, the statistics of SIRV are not
known and need to be estimated, especially the normalized clutter covariance matrix (NCCM).
With the known NCCM, the normalized matched filter (NMF) is derived in [6]. It is noted that, an
adaptive normalized matched filter (ANMF) can be obtained by replacing the unknown NCCM with
a suitable estimate in NMF. It is known that the traditional sample covariance matrix (SCM) is the
∗
Corresponding author (email: iamjiantao@yahoo.com.cn)