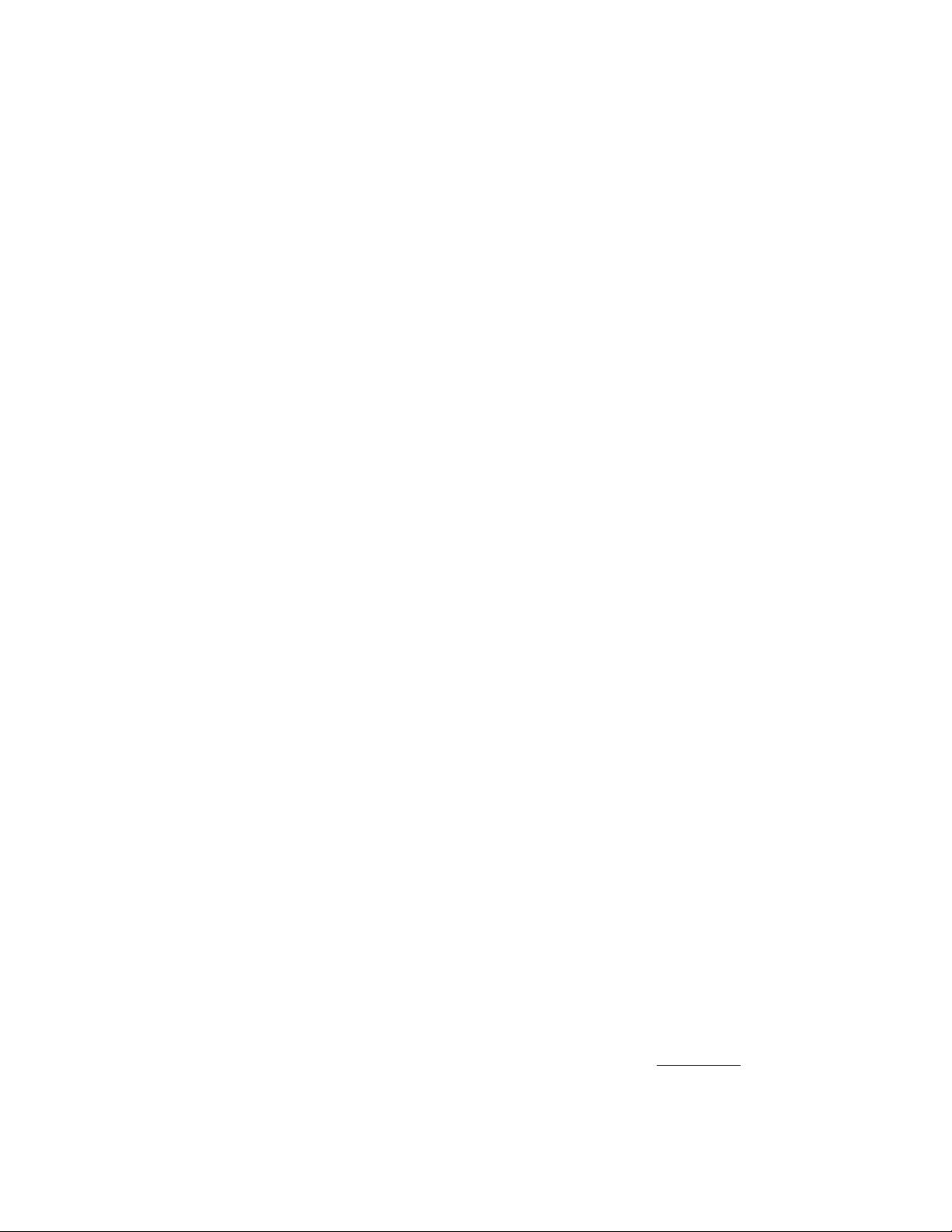
All Night Analysis of Snoring Signals by Formant Features
Kun Qian, Yuzhuo Fang, Zhiyong Xu*
School of Electronic and Optical Engineering,
Nanjing University of Science & Technology
Nanjing, China
ezyxu@mail.njust.edu.cn
Huijie Xu*
Otolaryngology Department,
Beijing Hospital
Beijing, China
xhj0531@163.com
Abstract—This paper presents our preliminary research on all
night analysis of formant features extracted from snoring
signals (SS), which can be used to find changes of anatomical
structure in the upper airway (UA) for patients suffering from
Obstructive Sleep Apnea-Hypopnea Symdrome (OSAHS). All
night analysis of formant features has a significance to reveal
the relationship between physiological or anatomical
mechanism and SS. It could be potentially helpful for doctors
to conduct an accurate and efficient surgical plan.
Keywords
-Obstructive Sleep Apnea-Hypopnea Symdrome
(OSAHS), snoring signals (SS), formant features, all night
analysis, clustering
I. INTRODUCTION
Obstructive Sleep Apnea-Hypopnea Symdrome (OSAHS)
is a serious chronic disorder which has a prevalent
community. It will impair the patient’s interpersonal
circumstance, lead traffic accidents, lower the quality of a
patient’s life, and even trigger high blood pressure, coronary
heart disease, pulmonary heart failure and some other life-
threatening diseases [1]. Polysomnogram (PSG) is regarded
as the gold standard in medical practice but has the
shortcomings of complexity, extravagance, unpleasant user’s
experience, etc. Therefore, numerous researchers are seeking
for a non-invasive method to be an alternative of PSG.
Among these ingenuities, the formant analysis based on
acoustic analysis shows a promising potential [2]-[3].
Azadeh et al. investigated the formants of SS and grouped it
into 7 clusters based on K-means clustering [2]. They found
that the first two formant frequencies (F1 and F2) are most
characteristic features in distinguishing the breath and snore
sounds from each other. Andrew et al. used formant features
to classify apneic and benign snores and they achieved a high
recognition rate (sensitivity: 88%, specificity: 82%) with
threshold value of F1 = 470 Hz via regression analysis [3].
However, it is more demanding for doctors to acquire
information about the obstructive site of the upper airway
(UA) of OSAHS patients rather than only diagnose this
disorder. Studies have also shown that the obstructive site of
the UA will experience a constant change during all night for
a patient [4]-[5], for instance, from palatal to non-palatal
parts of the UA. Hence, all night analysis of SS data should
be taken into practice. Hill et al. calculated acoustic crest
factor to show the snoring mechanism change in some
individuals during a night [4]. Mesquita et al., Raimon et al.,
and Duckitt et al. studied the all night SS data by time
interval, Apnea-Hypopnea Index (AHI), and Hidden Markov
Models (HMM), respectively [6]-[8].
This is a preliminary research for our group to embark an
unsupervised method to establish the SS database for further
study. We utilized K-means approach to group our SS data
into clusters based on the physical meaning indicated by
formant features. Combining the characterizations of the UA
structure variations (which imply the obstructive site changes
[5]) from formant features with all night analysis method of
an OSAHS patient, we cluster a 5-hour SS data into 8
clusters and selected the main 5 ones to analysis. The main 5
clusters are supposed to represent 5 typical states of the UA
structure.
II. M
ATERIALS AND METHODS
A. SS Data Acquisition and Pre-processing
This study received the approval of local ethics
committee. All night SS data of an OSAHS patient were
recorded in Beijing Hospital, China (People’s Republic of)
and we selected the most typical 5-hour part for analysis.
The experimental environment is a sleep laboratory. The
acquisition system consists of a low-noise microphone
(Studio-Project® B3, USA) positioned at roughly 0.5 m
above the patient’s mouth [9] and a data acquisition card (M-
Audio® Fast Track Ultra 8R, USA) configured at a sampling
frequency of 16 kHz with 16-bit resolution. Doctors
indicated that the cavity shape of the UA of this patient
varied during the whole night.
Based on Short-Time-Analysis method, the SS data were
framed with a Hamming window of 64ms by 50%
overlapping. Besides, an empirical Short-Time-Energy
threshold value was set to eliminate the background noise
before further analysis. As a result, we obtained 78,126
frames of SS data, namely 78,126 samples of SS for us to
analysis. Figure 1 illustrates the threshold for screening
snoring episodes.
B. Formant Features Extraction
Linear predictive coding (LPC) is used to model the
UA impulse respond function to generate SS, which is
depicted as follows [10]:
(1)