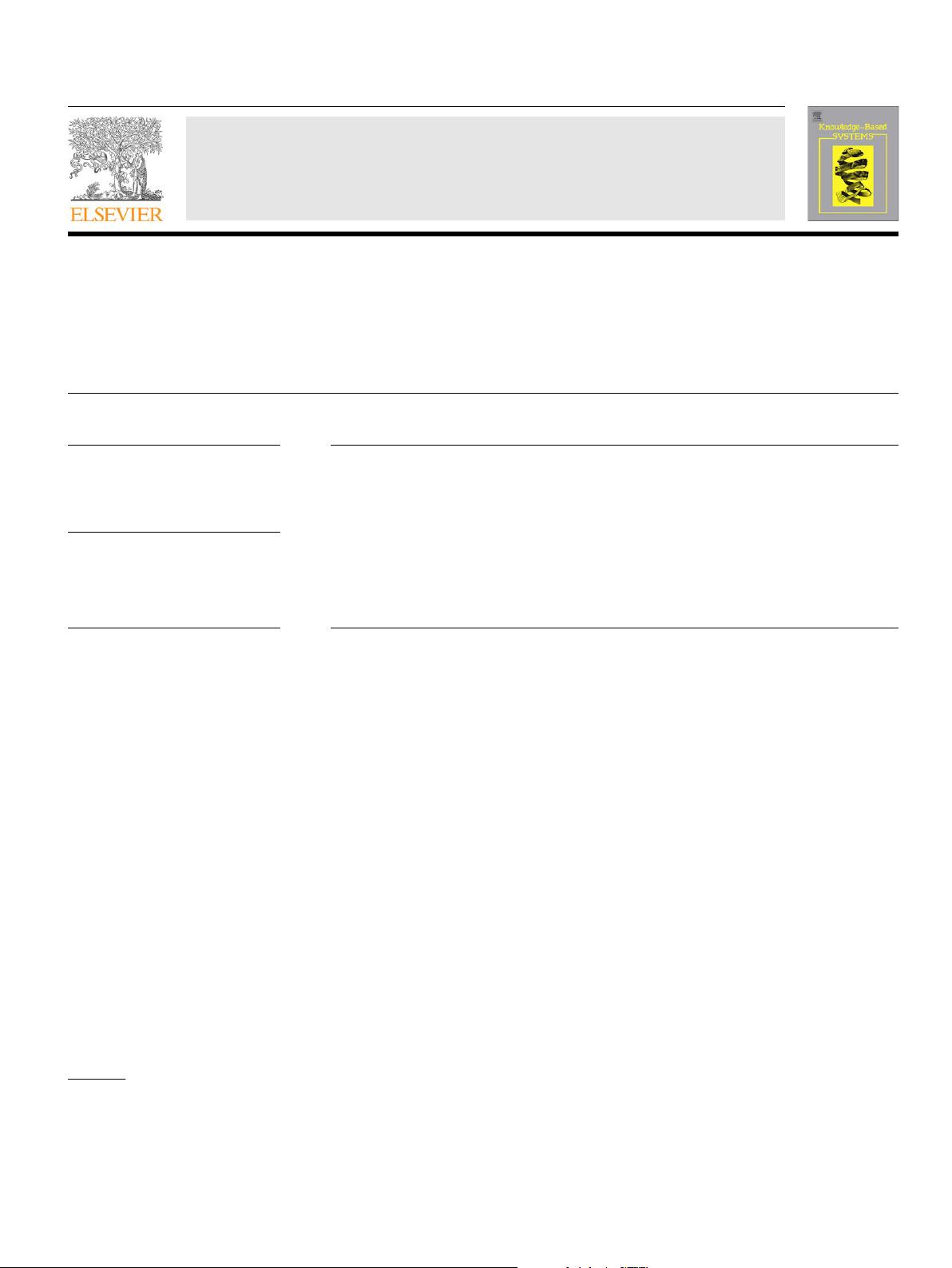
Approximation reduction in inconsistent incomplete decision tables
Yuhua Qian, Jiye Liang
*
, Deyu Li, Feng Wang, Nannan Ma
Key Laboratory of Computational Intelligence and Chinese, Information Processing of Ministry of Education, Taiyuan 030006, China
School of Computer and Information Technology, Shanxi University, Taiyuan, 030006 Shanxi, China
article info
Article history:
Received 30 June 2009
Received in revised form 8 January 2010
Accepted 16 February 2010
Available online 24 February 2010
Keywords:
Rough set theory
Inconsistent incomplete decision table
Maximal consistent block
Discernibility function
Approximation reduction
abstract
This article deals with approaches to attribute reductions in inconsistent incomplete decision table. The
main objective of this study is to extend a kind of attribute reductions called a lower approximation
reduct and an upper approximation reduct, which preserve the lower/upper approximation distribution
of a target decision. Several judgement theorems of a lower/upper approximation consistent set in incon-
sistent incomplete decision table are educed. Then, the discernibility matrices associated with the two
approximation reductions are examined as well, from which we can obtain approaches to attribute
reduction of an incomplete decision table in rough set theory.
Ó 2010 Elsevier B.V. All rights reserved.
1. Introduction
Since the original exposition of the rough set theory (RST) by
Pawlak [20–22] as a method of set approximation, it has continued
to flourish as a tool for data mining and data analysis
[2,3,24,25,31,34]. One fundamental aspect of RST involves a search
for particular subsets of condition attributes that provide the same
information for classification purposes as the full set of available
attributes. Such subsets are called attribute reductions [5,6].
Attribute reduction is performed in information systems (ISs)
by means of the notion of a reduct based on a specialization of
the general notion of independence because of Marczewski [15].
Many types of knowledge reductions have been proposed in the
area of rough sets [1–4,9,10,14,16–19,26–30,32,33,35], each of
the reductions aimed at some basic requirements. It is required
to provide their consistent classification. In the real world, most
decision information systems (also called decision tables) are
inconsistent because of various factors such as noise in data, com-
pact representation, prediction capability, etc. To acquire brief
decision rules from an inconsistent decision table, knowledge
reductions are needed. The inconsistence of a system makes it
infeasible to induce a set of certain definite rules covering all sys-
tem objects with confidence 1. Hence, one has to resort to obtain
rules from an inconsistent decision information system with confi-
dence less than 1 (usually called possible rules or probabilistic
rules). At present, rough set theory offers an unique approach for
generating such possible rules without a priori knowledge.
In recent years, more attention has been paid to knowledge
reduction in inconsistent systems in rough set research [9,10,
14,16,17,26,29,33,36]. b-reduct was studied in the variable preci-
sion rough set model proposed by Ziarko [32]. The notions of
a
-re-
duct and
a
-relative reduct for decision tables were defined. The
a
-
reduct allows the occurrence of additional inconsistency that is
controlled by means of
a
-parameter [19].In[29], Slezak presented
an attribute reduction that preserves the class membership distri-
bution for all objects in the ISs. It was shown by Slezak that the
attribute reduction preserving the membership distribution is
equivalent to the attribute reduction preserving the value of gener-
alized inference measure function [29]. A generalized attribute
reduction also was introduced in [28] that allows the value of gen-
eralized inference measure function after the attribute reduction to
be different from the original one by user-specified threshold. Five
kinds of attribute reductions and the relationships among them in
inconsistent systems were investigated by Kryszkiewicz [9],Li[10]
and Mi [16]. By eliminating the rigorous conditions required by
distribution reduct, maximum distribution reduct was introduced
by Zhang et al. in [33]. Unlike possible reduct [18], maximum dis-
tribution reduct can derive decision rules that are compatible with
the original systems.
According to whether or not there are missing data (null val-
ues), information systems can be classified into two categories:
complete and incomplete. By an incomplete information system
we mean a system with missing data (null values). We do not
0950-7051/$ - see front matter Ó 2010 Elsevier B.V. All rights reserved.
doi:10.1016/j.knosys.2010.02.004
* Corresponding author. Address: Key Laboratory of Computational Intelligence
and Chinese, Information Processing of Ministry of Education, Taiyuan 030006,
China.
E-mail addresses: jinchengqyh@126.com (Y. Qian), ljy@sxu.edu.cn (J. Liang),
ljdy@sxu.edu.cn (D. Li), sxuwangfeng@126.com (F. Wang), sxmanannan@126.com
(N. Ma).
Knowledge-Based Systems 23 (2010) 427–433
Contents lists available at ScienceDirect
Knowledge-Based Systems
journal homepage: www.elsevier.com/locate/knosys