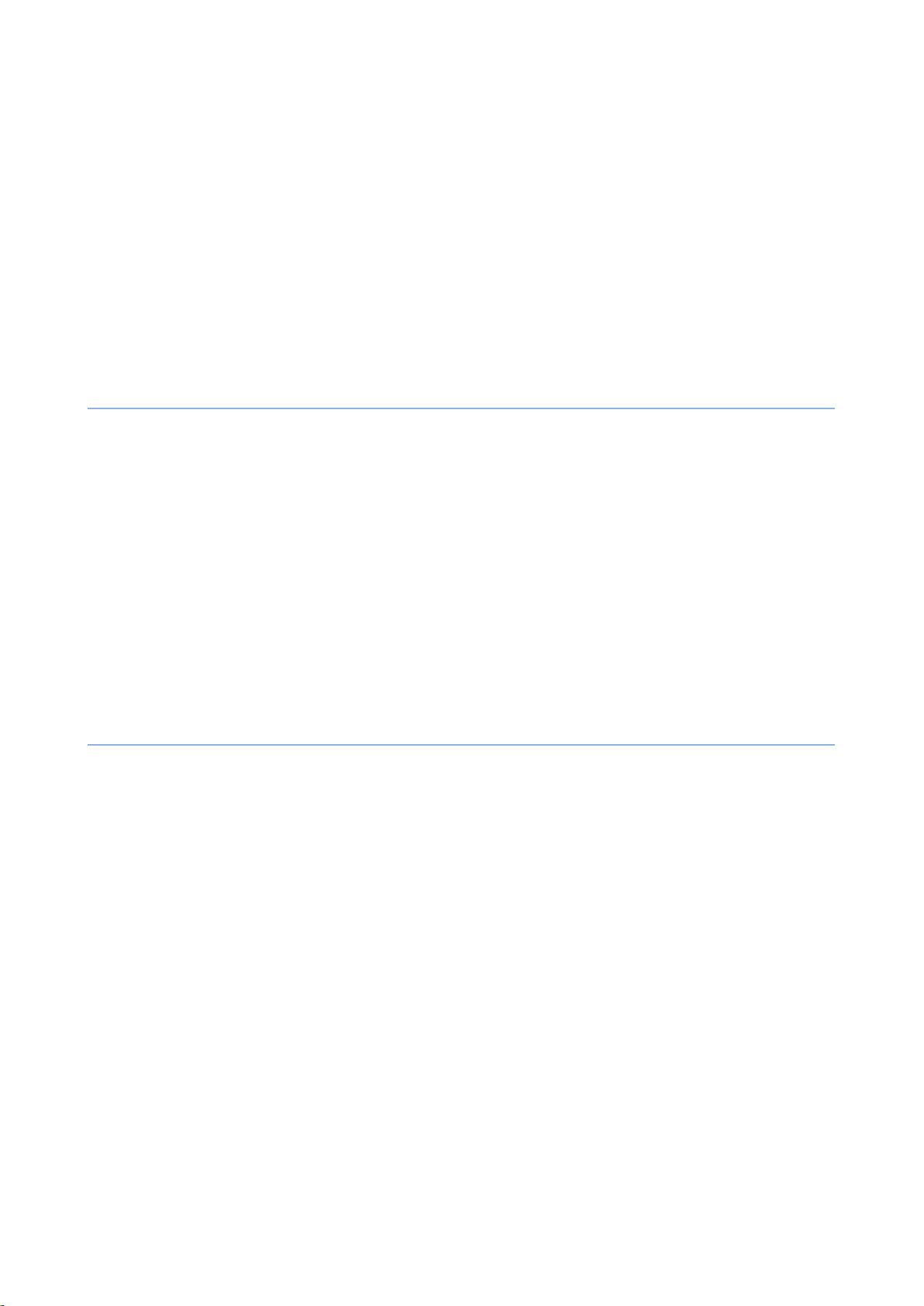
A Global Approach for Medical Image Denoising via Sparse
Representation
Yi Guo, Hanchao Chai
, Yuanyuan Wang
*
Department of Electronic Engineering, Fudan University, Shanghai, China.
* Corresponding author. Tel.: +86-21-65642756; email: yywang@fudan.edu.cn.
Manuscript submitted October 15, 2014; accepted December 20, 2014.
doi: 10.7763/ijbbb.2015.v5.391
Abstract: In this paper, a novel global noise reduction approach based on the sparse representation and
nonlocal means algorithm is proposed to enhance the image qualities of various medical imaging modalities,
including ultrasound images and magnetic resonance images. By using an overcomplete dictionary, a
medical image is decomposed into a sparsest coefficients matrix populated primarily with zeros. A nonlocal
means algorithm is developed to deal with these sparse coefficients to exploit the repetitive characters of
structures in the whole image, realizing a “truly” global denoising. With synthetic and clinical data of
ultrasound images and magnetic resonance images, this approach has been compared with other five
state-of-the-art denoising methods. The experiments quantitative results demonstrate the effectiveness of
our approach, especially superior in reducing the noise while well preserving the tissue details. It is
concluded that our proposed approach is capable of enhancing image quality in both ultrasound and
magnetic resonance images. It has a broad field of applications and will increase the diagnostic potential of
the medical images.
Key words: Global denoising, medical images, patches’ similarity, sparse representation.
1. Introduction
Due to the non-invasive, harmless and accurate nature, ultrasound imaging (US) and magnetic resonance
imaging (MRI) are widely used in diagnoses and treatments [1]. However, the imaging acquisition and
systems may introduce noises and artifacts, degrading contrast resolution and making medical images
difficult for non-specialists to interpret. Thus, to achieve the best diagnosis, it is essential to denoise medical
images without affecting important image features and destroying anatomical details [2].
In the past few decades, considerable efforts have been made in denoising the medical images. The local
statistics methods form an output by a weighted average calculation using sub-region statistics over
different pixel windows [3]-[5]. The median filter is a nonlinear operator, replacing the middle pixel in the
window with the median value of its neighbours, effectively in eliminating single and unrepresentative
pixels [6]. Anisotropic diffusion-based methods control the process of anisotropic diffusion based on
statistical characteristics of noise [7], [8]. The parametric estimation methods like the maximum-likelihood
filter and Bayesian denoising method establish noise models and adopt the robust parametric estimation
approaches [9], [10]. The wavelet-based denoising algorithms based on multi-scale decompositions of the
noisy images, apply soft thresholds to wavelet coefficients of different scales to eliminate the noise [11],
[12]. Although the above filters are capable of effectively suppressing the speckle pattern, they still remove
International Journal of Bioscience, Biochemistry and Bioinformatics
Volume 5, Number 1, January 2015