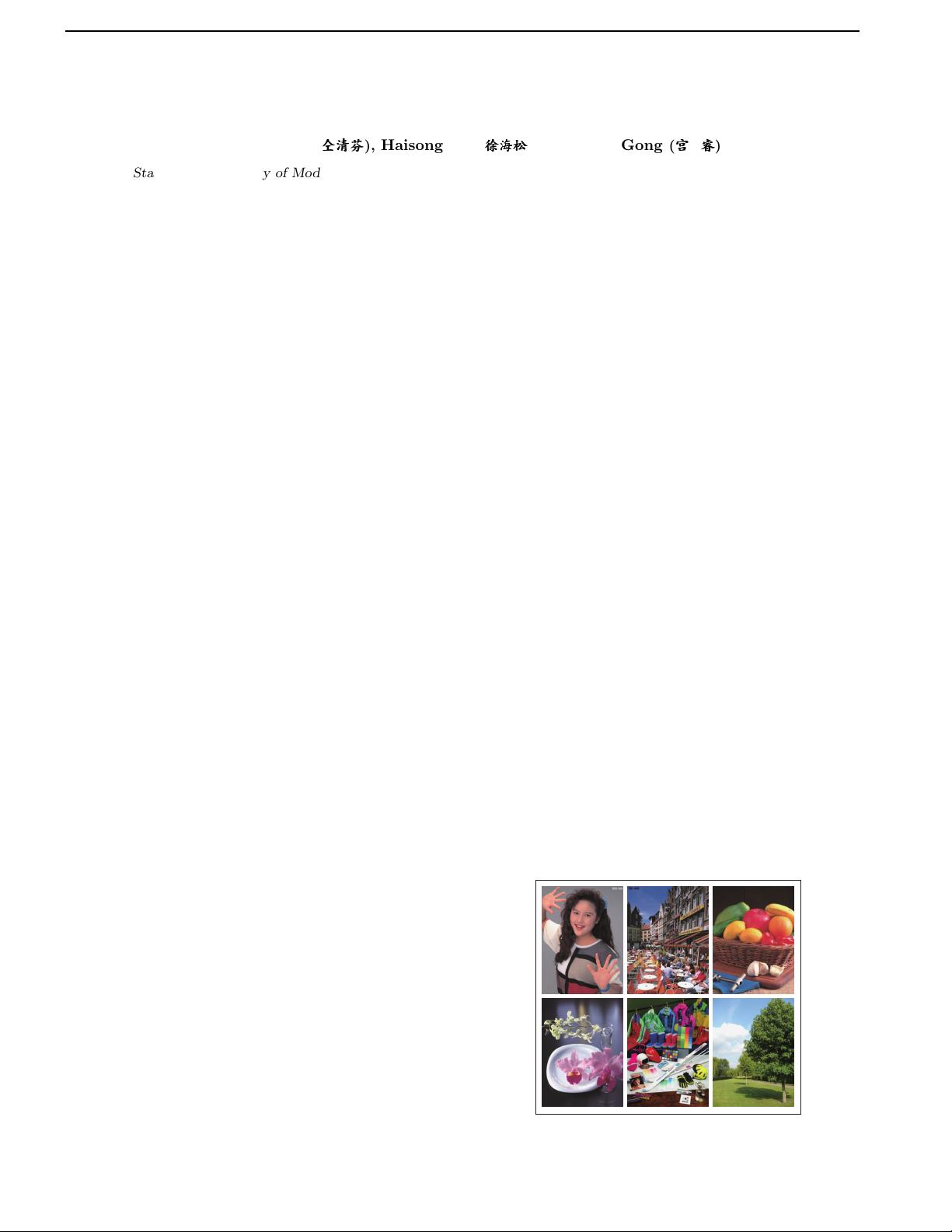
COL 11(7), 073301(2013) CHINESE OPTICS LETTERS July 10, 2013
Testing color difference evaluation methods
for color digital images
Qingfen Tong (
ééé
¥¥¥
), Haisong Xu (
MMM
°°°
ttt
)*, and Rui Gong (
ûûû
HHH
)
State Key Laboratory of Modern Optical Instrumentation, Zhejiang University, Hangzhou 310027, China
∗
Corresponding author: chsxu@zju.edu.cn
Received January 29, 2013; accepted April 12, 2013; posted online July 3, 2013
A psychophysical experiment is carried out to evaluate the color d ifference between pairs of color digital
images and their modulated versions, which are displayed on a professional liquid crystal display (LCD)
monitor, using the category judgment method. The modulated images for six original images are generated
with variations in five attributes, namely, lightness, chroma, hue, resolution, and sharpness, considering
both their chromatic and spatial characteristics. Several color difference evaluation methods, namely,
CIELAB, CIEDE2 000, CAM02-UCS, S-CIELAB, and iCAM, are compared based on the experimental
data. The results demonstrate that the p erformance of iCAM is the best for predicting image color
difference.
OCIS codes: 330.0330, 330.1720, 330.6110.
doi: 10.3788/COL201311.073301.
There are various methods reported on the traditional
color difference c alculation such as CIELAB
[1]
, CIEDE-
2000
[2]
, and CAM02-UCS
[3]
. These metho ds are all
established bas ed on uniform color patch samples. How-
ever, an ima ge is formed by a large number of pixels
with different colors, making it quite different from eva l-
uating the uniform color samples; such samples are also
difficult to measure directly with physical instruments.
As such, a method must be developed to evaluate the
color differe nce for c olor digital images.
In their research on image color difference evaluation,
Song et al.
[4−6]
, tested traditional color difference formu-
las in the image applications. Their results show that the
mean perceived color difference is roughly 2.5∆E
∗
ab
, but
those for different test images are quite different depend-
ing on the image contents, indicating that it is necessary
to consider the spatial characteristics of human vision
system and the complexity of image contents for image
color difference evaluation. Zhang et al.
[7]
proposed the
S-CIELAB image color difference model using an image
space filter according to the human visual contrast sen-
sitivity function. Moreover, Johnson et al.
[8]
proposed
a framework as a color image differe nce metric. The
framework extends the idea of spatial extension in the S-
CIELAB model by adding several pre-pro cessing steps,
including spatial filtering, adaptation, localization, as
well as local and global contrast detection. The mod-
ular image difference metric is then incorporated into
the image color appearance model (iCAM) to address
image appearance , difference, and quality within a single
model
[9,10]
.
In this letter, the color differences among C IELAB,
CIEDE2 000, CAM02-UCS, S-CIE L AB, and iCAM for
each image pair, w hich are base d on the visual experi-
ment for the standard test imag e s and their modulated
versions, are calculated and compared with the subjec-
tive evaluation results of the observers. The calculation
and comparisons are performed to further investigate the
image color difference evaluation methods.
Six test images were se le c ted to cover the colors of
the familiar objects, four of these were ISO SCID 300
images, including N1 (Woman), N2 (Street), N3 (Fruits),
N6 (Flower), one from a CIE TC8-03 sRGB image named
Ski, to gether with an additional image “Tree” for the col-
ors of sky and plants (Fig. 1). These images were all
clipped to ensure they had the same size of 15 × 20
(cm). To generate test images similar to the original im-
ages under a limited extent of difference, the six original
images were manipulated in terms of five attributes, i.e.,
lightness, chroma, and hue angle corresponding to L
∗
, C,
and H of CIELAB color space, respec tively; resolution
(R); and sharpness (S), according to the functions and
parameters listed in Table 1. Five linear manipulations
in the L
∗
and C channels were performed to investigate
the effect of linear changes in lightness and chroma. Re-
ductions and increases in lightness and chroma contrast
were simulated using the sigmoid and inverse-sigmo id
functions in L
∗
and C, respectively
[11]
. The variations
of image resolution were manipulated using the bicubic
resampling method, equivalently regarding 2 × 2, 3 ×
3, 4 × 4 pixels as 1 × 1 pixels. One method based on
a high frequency emphasis filter
[11]
, was applied to in-
crease sharpness. A total of 216 test images (6 images ×
36 manipulations) were produced.
The visual experiment was conducted in a dark room.
Fig. 1. Six original test images for the psychophysical evalu-
ation.
1671-7694/2013/073301(4) 073301-1
c
2013 Chinese Optics Letters