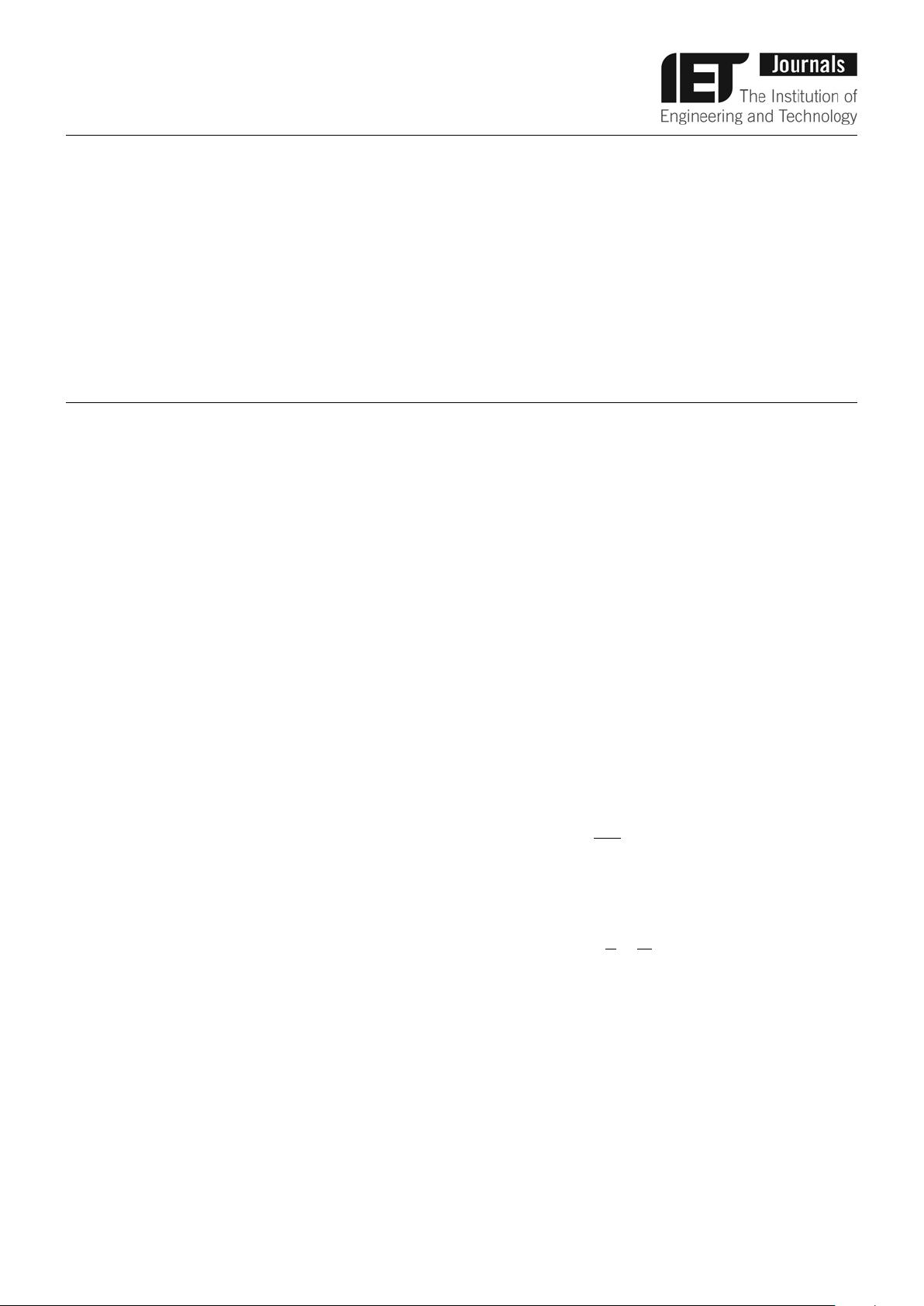
Zak transform and uncertainty principles
associated with the linear canonical transform
ISSN 1751-9675
Received on 21st November 2015
Revised on 2nd April 2016
Accepted on 13th April 2016
doi: 10.1049/iet-spr.2015.0514
www.ietdl.org
Qingyue Zhang
✉
College of Science, Tianjin University of Technology, Tianjin 300384, People’s Republic of China
✉ E-mail: jczhangqingyue@163.com
Abstract: Several new results about Zak transform and uncertain ty principles in the linear canonical transform (LCT)
domains are presented. The results obtained rely mainly on relationship b etween the LCT and the classical Fourier
transform. The findings will likely have potential applications in optics and signal processing.
1 Introduction
The linear canonical transform (LCT) is the genera lised form of the
classical Fourier transfo rm (FT), the fractional Fourier transform (FrFT)
and the Fresnel transform [1]. As a pow erful analysing tool, LCT has
been widely used in many fields such as optics and signal processin g
[1, 2]. In this paper, we discuss the Zak transform and the uncertainty
principle (Heisenberg–Pauli–W eyl inequality) in the LCT domains.
The classical Zak transform is a certain operation which takes as
input a function of one variable and produces as output a function
of two variables. It has been used successfully in quantum physics
field [3], in time-frequency representation of signals [4], and in
sampling theorem [5]. The results about the classical Zak
transform in the LCT domains appear to be missing in the
literature and we want to fill this gap.
The classical Poisson summation formula is a very important
concept in signal processing community. Li et al.[6] investigated
the classical Poisson summation formula in the FrFT domains.
Zhang and Hou studied the classical Poisson summation formula
associated with the LCT [7]. We derive Poisson summation formula
in the LCT domains by a property of Zak transform. Although
Zhang and Hou had given the classical Poisson summation formula
for the LCT in [7], we reduce the requirements on the signal.
In digital signal and image processing and digital communications,
a continuous signal is usually represented and processed by using its
discrete samples. Then a fundamental problem is how to represent a
continuous signal in terms of a discrete sequence. By the famous
classical Shannon sampling theorem [8, Theorem 1.2], for a
band-limited signal of finite energy, it is completely characterised
by its samples. By a property of Zak transform, we obtain a result,
which generalises classical Shannon sampling theorem.
The uncertainty principle is any of a variety of mathematical
inequalities asserting a fundamental limit to the precision with
which certain pairs of physical properties of a particle, known as
complementary variables, such as position x and momentum p,
can be known simultaneously. It was first introduced by the
German physicist Werner Heisenberg in 1927, and it states that the
more precisely the position of some particle is determined, the less
precisely its momentum can be known, and vice versa [9]. Hence,
the uncertainty principle is also known as Heisenberg’s uncertainty
principle in quantum mechanics. The formal inequality relating the
standard deviation of position σ
x
and the standard deviation of
momentum σ
p
was obtained by Kennard [10] later that year and
by Weyl [11] in 1928
s
x
s
p
≥ h
−
.
Here, h
−
is the reduced Planck constant h/2π.
Recently, researchers discussed the classical uncertainty principle
(Heisenberg–Pauli–Weyl inequality) for LCT [2, 12–22]. However,
to the best of our knowledge, there are no results published about the
Amrein–Berthier–Benedicks qualitative uncertainty principle, the
Donoho–Stark uncertainty principle, the Nazarov uncertainty
principle, the Hardy uncertainty principle and the Beurling
uncertainty principle in the LCT domains. In this paper, we study
these five uncertainty principles in the LCT domains.
Before stating our results, we give some definitions. The LCT with
parameter
A =
ab
cd
of a signal f(t)isdefined as in [2, 16, 18, 19, 22, 23]
L
A
f (u) =
R
f (t)K
A
(t, u)dt, b = 0,
d
√
e
i(cd/2)u
2
f (du), b = 0,
where a, b, c, d are real numbers satisfying ad − bc = 1, i.e. detA =1,
and the kernel of LCT is
K
A
(t, u) =
1
i2
p
b
e
(i/2) (a/b)t
2
−(2/b)tu+(d/b)u
2
,(b = 0).
Denote (U
α
f )(x)=e
iαx
2
f(x), (D
a
f )(x)=|a|
1/2
f(ax), where a ≠ 0.
Then for b ≠ 0, we have
L
A
f =
1
ib
1
|b|
−1
U
d /2b
D
1/b
FU
a/2b
f , (1)
where (Ff )(u) = (1/
2
p
√
)
R
f (x)e
−ixu
dx is the FT of f.
Let
A
1
=
a
1
b
1
c
1
d
1
, A
2
=
a
2
b
2
c
2
d
2
,
and det A
1
= det A
2
= 1.
In Section 2, we give an important property of Zak transform in the
LCT domains and the property’s applications. In Section 3, five
uncertainty principles (the Amrein–Berthier–Benedicks qualitative
uncertainty principle, the Donoho–Stark uncertainty principle, the
Nazarov uncertainty principle, the Hardy uncertainty principle and
the Beurling uncertainty principle) in the LCT domains are
IET Signal Processing
Research Article
IET Signal Process., 2016, Vol. 10, Iss. 7, pp. 791–797
791
&
The Institution of Engineering and Technology 2016