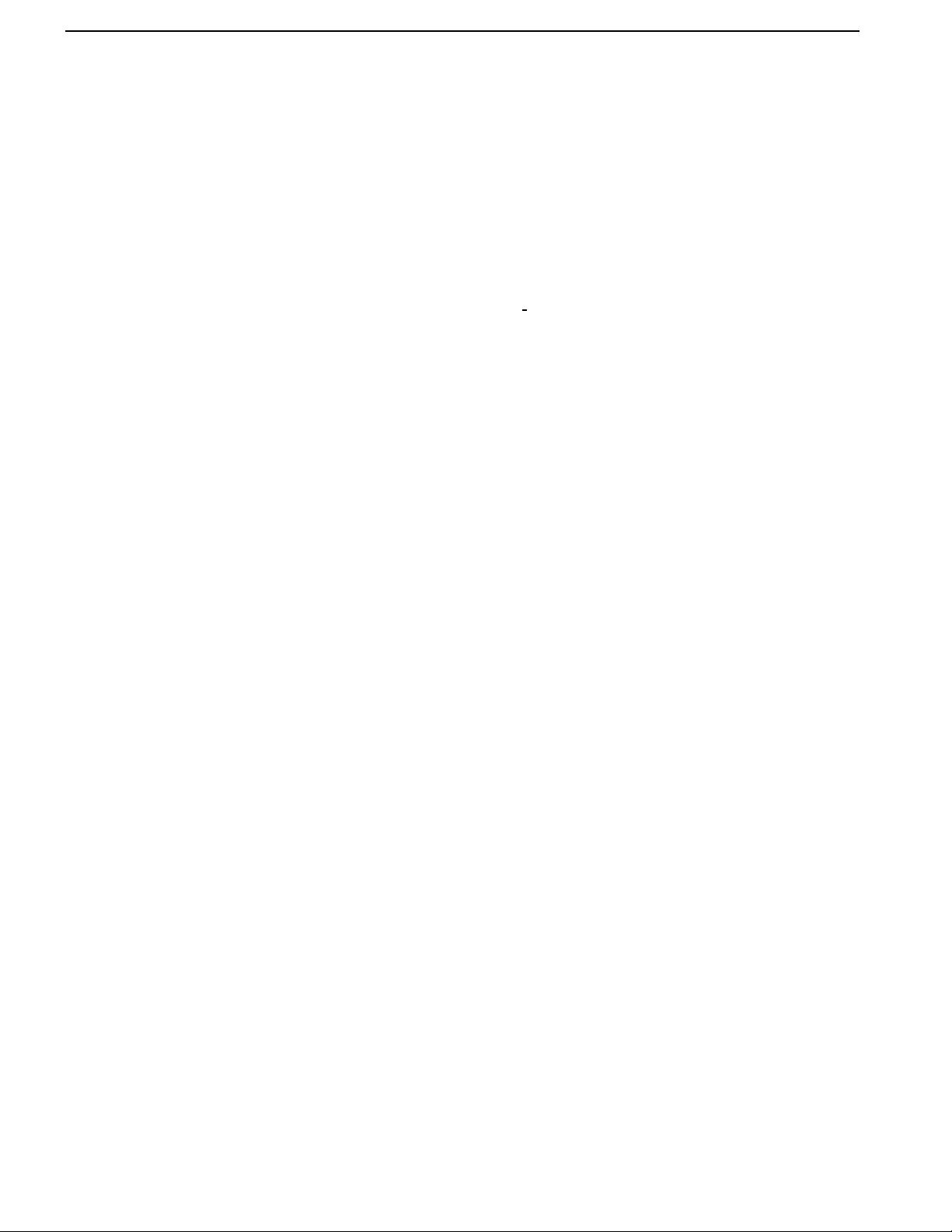
COL 10(Suppl.), S11005(2012) CHINESE OPTICS LETTERS June 30, 2012
Performance evaluation and segmentation for synthetic
aperture radar image using mixture multiscale
autoregressive model and bootstrap technique
Haixia Xu (MMM°°°___)
1,2∗
, Xianbin Wen (§§§wwwRRR)
1,2
, Yongliao Zou (qqq[[[)
3
, and Yongchun Zheng (xxx[[[SSS)
3
1
Tianjin Key Laboratory of Intelligence Computing and Novel Software Technology,
Tianjin University of Technology, Tianjin 300384, China
2
Key Laboratory of Computer Vision and System of Ministry of Education,
Tianjin University of Technology, Tianjin 300384, China
3
National Astronomical Observatories, Chinese Academy of Sciences, Beijing 100012, China
∗
Corresponding author: xuhaixia xhx@163.com
Received December 14, 2011; accepted January 18, 2012; posted online April 27, 2012
An unsupervised segmentation and its performance evaluation technique are proposed for synthetic aper-
ture radar (SAR) image based on th e mixture multiscale autoregressive (MMAR) mod el and the bootstrap
method. The segmentation-evaluation techniques consist of detecting the number of image regains, esti-
mating MMAR parameters by using bo otstrap stochastic annealing expectation-maximization (BSAEM)
algorithm, and classifying pixels into region by using Bayesian classifier. Experimental results demonstrate
that the evaluation operation is robust, and the proposed segmentation method is superior to the tradi-
tional single resolution techniques, and considerably reduces t he computing time over the EM algorithm.
OCIS codes: 100.0100, 280.6730.
doi: 10.3788/COL201210.S11005.
Synthetic aperture radar (SAR) imaging s ystems have
been widely used in the past several years for remote
sensing applications. One of the main advantages o f these
systems is the ability to operate at any time of day, in
any weather conditions, and to improve the image reso-
lution fo r a given ap e rture size. However, co herent active
imaging has the drawback of leading to images that are
degraded by speckle noise w hich makes the images grainy
and deteriorate the imag e processing performances. The
low-level step of image segmentation, i.e., the decompo-
sition of the ima ge in a tessellation of unifor m areas, is a
crucial point of SAR images processing.
Numerous segmentation methods have been proposed
in the res e arch literatur e , e.g., thresholding methods
[1,2]
,
clustering methods
[3,4]
, edge-based metho ds
[5]
, region
splitting and merging methods
[6,7]
, and multi-resolution
techniques
[8−16]
. Recently, to characterize and exploit
the scale-to- scale statistical variations in SAR image duo
to radar speck le
[11]
, various multiscale stochastic models
have been developed and used for segmentation of SAR
image. Basse rille et al. proposed a multiscale autoregres-
sive model for signal and image processing
[15]
. Fosgate
et al., Irving et al., and Kim et al. build the models rep-
resentative of each category of terra in of interest SAR
image and employ them in directing decisions on pixel
classification, segmentation behavior
[11−13]
. Comer et al.
proposed a segmentation algorithm based on the mul-
tiresolution Gaussia n autoregre ssive model for the ob-
served image pyramid
[17]
. The algorithm used a multires-
olution Gauss ian autoregressive model for the pyramid
representation of observed image, and assumes a mul-
tiscale Ma rkov random field model for the class lab e l
pyramid. Wen et al. proposed a mixture multiscale au-
toregres sive (MMAR) model for the segmentation of SAR
image
[18]
, and gave Bayesia n segmentation for SAR im-
age based on the MMAR model, which parameters are
estimated by using expectation-maximization (EM) al-
gorithm. MMAR model provides a p owerful multiscale
and semi-parameter framework fo r describing unknown
distributional shape of complex random field that evolve
in s c ale. However, the EM algorithm has the problem
that the solution converges to a local optimal due to the
dependence of the initial state of parameters in the pos-
terior distribution. On the other hand, in the statistical
segmentation, the time increases with the size of training
data set. In most of the real-world application, the size
of the training data is very large. As a result, the time
required by segmentation could be prohibitively large.
Finally, how good are these techniques in ter m of their
detection and c lassification performances is not known.
This letter addresses SAR ima ge segmenta tion and
performance evaluation bas e d Bootstrap technology and
mutiscale stochastic model on the tree without supervi-
sion. Firstly, the estimation algorithm by using Boot-
strap technique
[19]
, namely, bootstrap stochastic anneal-
ing EM (BSAEM) algorithm, is pro posed, which algo-
rithm improves the EM algorithm by adding a n o ptimal
Bootstrap sample selection and decor relation step to the
blind a pproach, and would converge more rapidly to the
good solution then the classical EM algorithm. Secondly,
the detection performance based on the MMAR model
is discussed, where the statistical probabilities o f over-
detection a nd under-detection of the number of image
regions are defined, and the corresponding formula in
terms of the model parameters and the image quality are
derived. Thirdly, we define misclassification probabil-
ity for the B ayesian classifier and give a simple formula
to evaluate segmentation errors based on the parameter
estimates and classified data. Finally, tests have been
conducted on three real SAR images for evaluating de-
1671-7694/2012/S11005(6) S11005-1
c
2012 Chinese Optics Letters