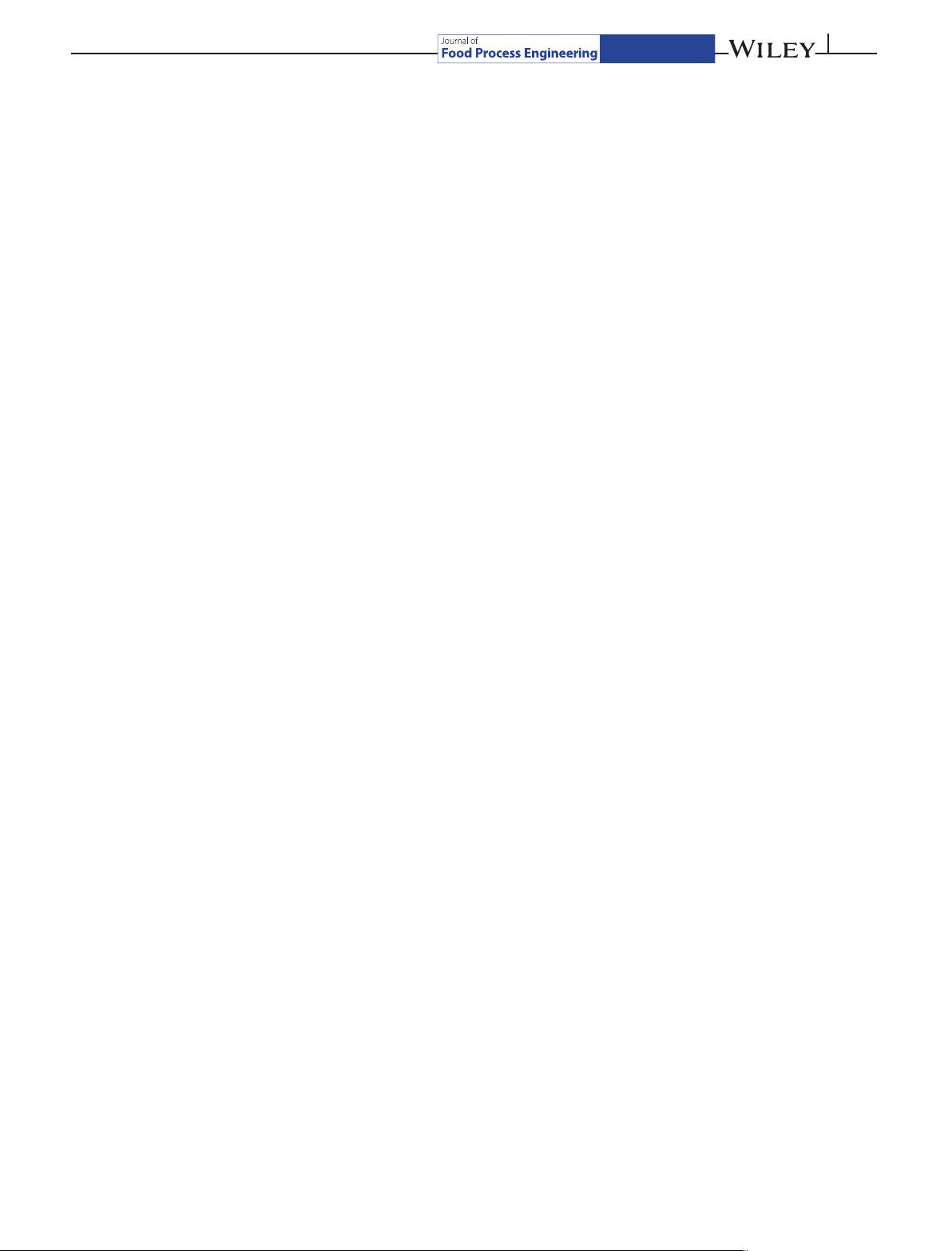
of white radishes for detecting hollowness defects, respectively. The
detection results indicated that transmittance and semi-transmittance
imaging modes coupled with the back propagation artificial neural
network model could get more satisfactory identification accuracies
for classifying between normal and hollowness. All these studies
showed that hyperspectral imaging with transmittance mode was
potential for internal quality assessment of horticultural products due
to the fact that the deeper information from the detected object can
be obtained. However, as far as we know, few works have focused on
measuring the SSC of horticultural products by using hyperspectral
transmittance imaging technique. Therefore, it might be the first time
that this technique was utilized to quantitatively determinate the SSC
in processing tomatoes. In addition, in terms of hyperspectral imaging
for SSC detection of horticultural products, it should be pointed out
that most of researches used samples with similar size for avoiding
size influence on the detection accuracy of models. This is clearly less
attractive for on-line real grading application. In this work, we used
different size of samples for more closely aligned with the actual
applications. However, transmittance spectra of tomatoes with differ-
ent sizes have the different optical path (Clark, McGlone, & Jordan,
2003; Guthrie, Walsh, Reid, & Liebenberg, 2005; Krivoshiev,
Chalucova, & Moukarev, 2000; Magwaza, Opara, Cronje, Saeys, &
Nicolaï, 2012). Moreover, in the preliminary experiments, we also
found that processing tomatoes with the same sizes may have differ-
ent weights, showing the densities of different tomatoes have a dif-
ference. Density difference also affects the optical path and further
affects the prediction accuracy of models. Therefore, this study also
tried to develop the multi-parameter compensation model by combin-
ing with spectrum, size and weight information of processing toma-
toes. The main goals of this article were as follows:
1. To set up a hyperspectral imaging system with the transmittance
mode providing spectral information of 400–1,000 nm and evalu-
ate its capacity of using hyperspectral transmittance imaging to
quantitatively analyze the SSC in processing tomatoes;
2. To identify those important wavelengths that represent the most
useful information for the measurement of SSC of processing
tomatoes by using competitive adaptive reweighted sampling
(CARS) algorithm for development of low-priced and on-line multi-
spectral imaging detection equipment;
3. To establish the multi-parameter compensation linear PLSs models
and nonlinear least squares-support vector machine (LS-SVM)
models and further compare performance of these models in the
SSC evaluation of processing tomatoes.
2 | MATERIALS AND METHODS
2.1 | Processing tomatoes
In total, 200 processing tomatoes with all red peel were collected
from local plantation in Shihezi City (Xinjiang province, China) during
the harvest season in August 2017. Because defects can affect the
spectral characteristics, the used samples have no any surface defects
or bruises. However, two samples were found with the internal rot-
tenness when all samples were destructively measured for obtaining
the real SSC. Thus, 198 processing tomatoes were used in this study.
Different from existing works, samples with similar sizes were usually
used to establish the models for reducing the influence of sizes on
SSC detection accuracy. In this study, all processing tomatoes with
the transverse equatorial diameter range of 45–76 mm were directly
used to acquire the hyperspectral images according to real application
of on-line grading. Before images data acquisition, samples were first
marked and then placed in the lab with 60% relative humidity and
20
C environment temperature for more than 2 days (Cozzolino
et al., 2007).
2.2 | Three-dimensional (3D) data acquisition system
A laboratory Vis-NIR transmittance hyperspectral imaging system was
constructed and utilized to acquire spectral images of processing
tomatoes. The constructed imaging system and the acquired 3D data
were shown in the left and right of Figure 1, respectively. The sys-
tem consisted of an imaging spectrograph (ImSpector V10E-QE,
Spectral Imaging Ltd., Oulu, Finland), a high sensitive CCD camera
(C8484-05G01, Hamamatsu Photonics, Japan) with a standard C-
mount zoom lens (V23-f/2.4, Specim Ltd., Finland), an illumination
unit with 150 W halogen lamp (SCHOTT DCRIII, SCHOTT North
America, Inc., Elmsford, NY, USA), an electronically-controlled
motorized horizontal stage (PSA-200-11-X,Zolix Inc. Beijing, China)
and a computer (Lenovo, Inter(R)Core(TM)i3-3240 CPU@3.4 GHz,
RAM 4.00 GB). The image acquisition software (SpecViev Isuzu
Optics Corp., Taiwan) and movement stage control softwar e (Isuzu
Optics Corp., Taiwan) were installed on the computer. In the terms
of transmittance mode, the halogen lamp was fixed directly below
the tested sample. Sample horizontal movement speed and camera
exposure time were set at 15 mm/s and 5 ms, respectively, to avoid
image distortion. I n order to reduce interference from ambient light,
a black rubber seal was set in the optical round hole between halo-
gen lamp and the tested sample. The image acquisition system can
obtain the images covering the spectral range of 400–1,000 nm with
the wavelength interval of ~1.27 nm. Thus, a total of 473 single-
wavelength images were produced in a r aw hyperspectral image.
Hype rspectral image of each sample was recorded as a 3D data
block including image information in the direction of axis x-y and
spectral information in the direction of axis λ, a s shown in the left
side of Figure 1.
2.3 | Original hyperspectral image correction
Halogen lamp produces a spatial intensity variation in the field of view
of camera and the dark current also exists in the CCD camera, which
could result in the obvious noises at both ends of wavelengths due to
relative low illumination. Therefore, the original spectral images (R
0
)
were necessary to perform the flat field calibration on the basis of
dark reference (R
d
) and white reference (R
w
). The image without any
light was collected as dark reference by covering the camera lens and
WANG ET AL. 3of11