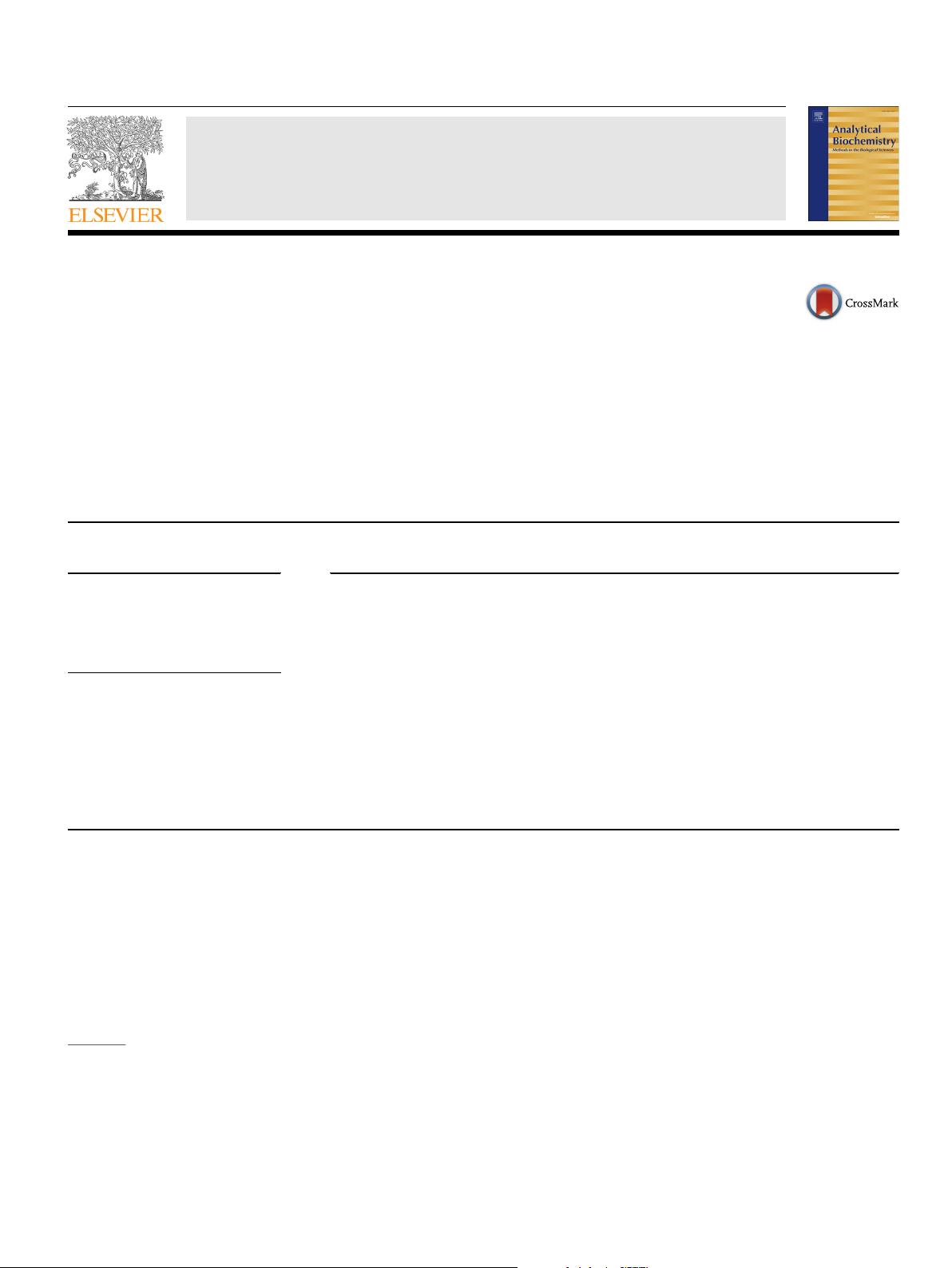
DRME: Count-based differential RNA methylation analysis at small
sample size scenario
Lian Liu
a
, Shao-Wu Zhang
a
,
**
, Fan Gao
b
, Yixin Zhang
c
, Yufei Huang
d
,
Runsheng Chen
a
,
e
, Jia Meng
f
,
*
a
Key Laboratory of Information Fusion Technology of Ministry of Education, School of Automation, Northwestern Polytechnical University, Xi'an 710072,
China
b
Picower Institute for Learning and Memory, Department of Brain and Cognitive Science, Massachusetts Institute of Technology, Cambridge, MA 02139, USA
c
Suzhou Urban and Environmental Research Institute, Huai'an Research Institute of New-type Urbanization, Department of Environmental Sciences, Xi'an
JiaotongeLiverpool University, Suzhou 215123, China
d
Department of Electrical and Computer Engineering, University of Texas at San Antonio, San Antonio, TX 78230, USA
e
Institute of Biophysics, Chinese Academy of Sciences, Beijing 100101, China
f
Department of Biological Sciences, Xi'an JiaotongeLiverpool University, Suzhou 215123, China
article info
Article history:
Received 7 August 2015
Received in revised form
22 January 2016
Accepted 25 January 2016
Available online 4 February 2016
Keywords:
N
6
-Methyladenosine (m
6
A)
RNA methylation
MeRIP-Seq
Differential methylation
R/Bioconductor package
Negative binomial distribution
abstract
Differential methylation, which concerns difference in the degree of epigenetic regulation via methyl-
ation between two conditions, has been formulated as a beta or beta-binomial distribution to address the
within-group biological variability in sequencing data. However, a beta or beta-binomial model is usually
difficult to infer at small sample size scenario with discrete reads count in sequencing data. On the other
hand, as an emerging research field, RNA methylation has drawn more and more attention recently, and
the differential analysis of RNA methylation is significantly different from that of DNA methylation due to
the impact of transcriptional regulation. We developed DRME to better address the differential RNA
methylation problem. The proposed model can effectively describe within-group biological variability at
small sample size scenario and handles the impact of transcriptional regulation on RNA methylation. We
tested the newly developed DRME algorithm on simulated and 4 MeRIP-Seq caseecontrol studies and
compared it with Fisher's exact test. It is in principle widely applicable to several other RNA-related data
types as well, including RNA Bisulfite sequencing and PAReCLIP. The code together with an MeRIP-Seq
dataset is available online (https://github.com/lzcyzm/DRME) for evaluation and reproduction of the
figures shown in this article.
© 2016 Elsevier Inc. All rights reserved.
Differential methylation analysis concerns the difference in the
degree of methylation between two conditions, which has shown
to be of crucial biological significance [1]. When sequencing
approachesdsuch as ChIP-Seq, BS-Seq, and MeRIP-Seqdare used,
the differential methylation problem is often formulated as either a
2 by 2 contingency table or a beta or beta-binomial distribution
[2e5]. Whereas testing the independence of a 2 by 2 contingency
table with Fisher's exact test cannot incorporate within-group
variability and so does not effectively take advantage of biological
replicates, beta-binomial distribution is often very intricate to infer,
especially at small sample size scenario. Recently, a number of ef-
forts have been made to address the differential methylation
problems [6e8]. Despite the recent prosperity in DNA methylation
analysis methodologies [8e16], count-based small sample estima-
tion of biological variability methylation analysis remains a difficult
problem.
As a newly emerging research field, RNA methylation has drawn
a significant amount of attention recently for its role in various
biological functions [17e27]. A key technique developed for global
unbiased profiling of the RNA methylome is MeRIP-Seq, which is an
affinity-based sequencing approach that captures the RNA frag-
ments with the post-transcriptional modification mark of interests
in the so-called IP sample; meanwhile, an Input sample is often
generated by sequencing the basal expression of all genes as the
background information [18,25,28]. Besides apparent functional
Abbreviations used: K/O, knockout; W T, wild-type; DAA, 3-deazaadenosine;
GEO, Gene Expression Omnibus; m
6
A, N
6
-methyladenosine; FTO, Fat mass and
obesity-associated gene.
* Corresponding author.
** Corresponding author.
E-mail addresses: zhangsw@nwpu.edu.cn (S.-W. Zhang), jia.meng@xjtlu.edu.cn
(J. Meng).
Contents lists available at ScienceDirect
Analytical Biochemistry
journal homepage: www.elsevier.com/locate/yabio
http://dx.doi.org/10.1016/j.ab.2016.01.014
0003-2697/© 2016 Elsevier Inc. All rights reserved.
Analytical Biochemistry 499 (2016) 15e23