"基于多尺度YOLOv5的高效实时交通灯检测算法优化研究"
2.虚拟产品一经售出概不退款(资源遇到问题,请及时私信上传者)
Traffic light detection is a crucial task in the field of autonomous driving, as it directly impacts the safety of vehicles on the road. The difficulty in detecting traffic lights due to their small scale and complex environmental conditions has prompted the development of a multi-scale YOLOv5 traffic light detection algorithm. This approach involves utilizing a combination of data augmentation techniques to enhance the complexity of the model input, as well as training the model at multiple scales instead of a fixed scale to improve its learning capabilities. Additionally, a multi-scale feature fusion network is constructed to merge information from downsampled scales of 4x, 8x, 16x, and 32x, creating a multi-scale detection layer. To enhance the feature fusion capability, long-range skip connections are introduced to transfer information between different levels, significantly improving the model's ability to detect small objects. Experimental results demonstrate that the improved YOLOv5 algorithm achieves a detection speed of up to 9.5ms and an mAP of 99.8% on the collected dataset, representing a 17% improvement over the original YOLOv5 model. Furthermore, on the Bosch dataset, the mAP increases by 6.5%, enabling real-time and high-precision traffic light detection.
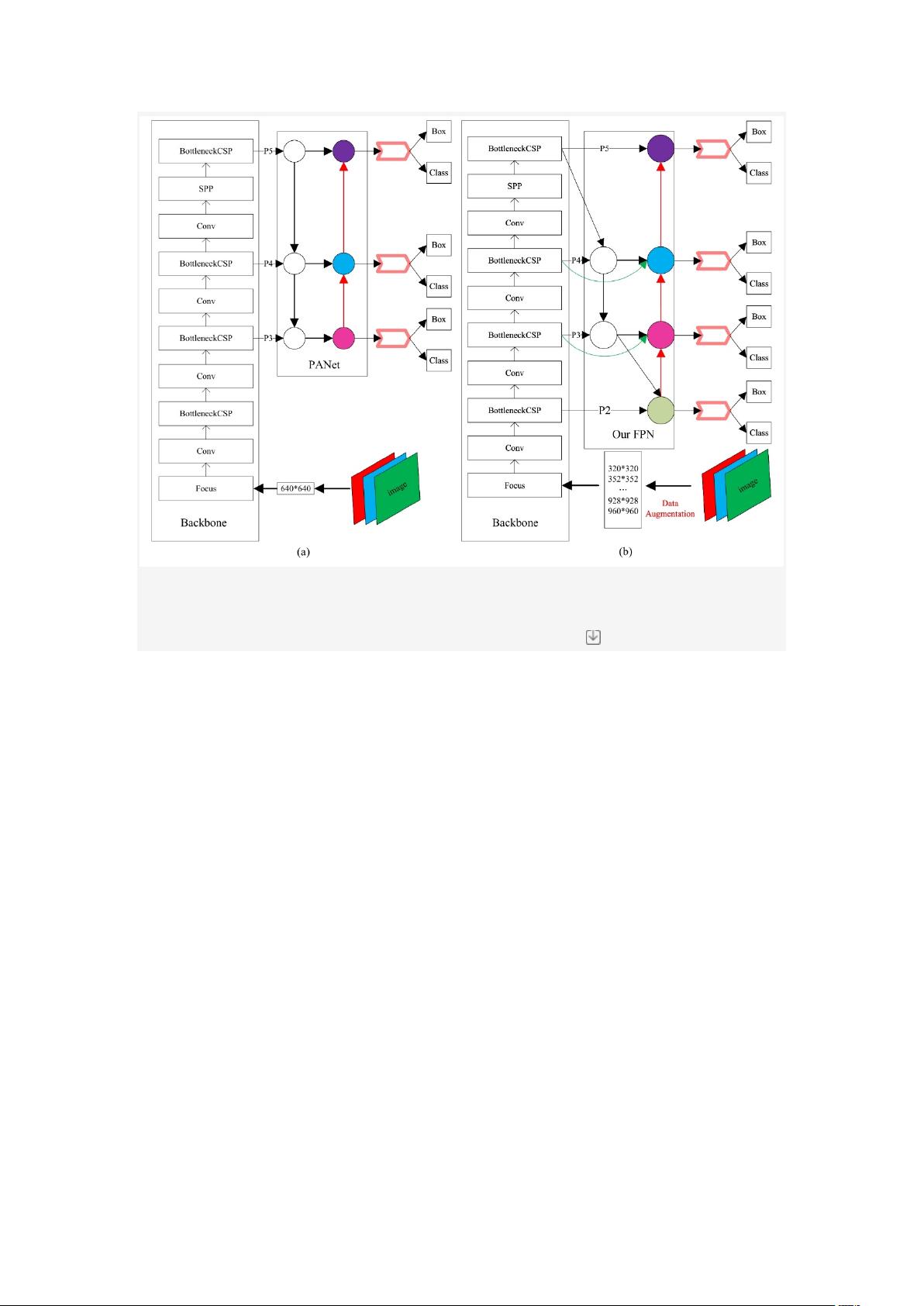
剩余15页未读,继续阅读
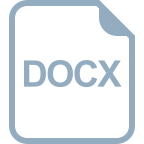
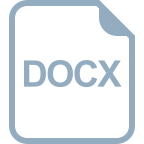

















- 粉丝: 4129
- 资源: 1万+





我的内容管理 收起
我的资源 快来上传第一个资源
我的收益
登录查看自己的收益我的积分 登录查看自己的积分
我的C币 登录后查看C币余额
我的收藏
我的下载
下载帮助

会员权益专享
最新资源
- 京瓷TASKalfa系列维修手册:安全与操作指南
- 小波变换在视频压缩中的应用
- Microsoft OfficeXP详解:WordXP、ExcelXP和PowerPointXP
- 雀巢在线媒介投放策划:门户网站与广告效果分析
- 用友NC-V56供应链功能升级详解(84页)
- 计算机病毒与防御策略探索
- 企业网NAT技术实践:2022年部署互联网出口策略
- 软件测试面试必备:概念、原则与常见问题解析
- 2022年Windows IIS服务器内外网配置详解与Serv-U FTP服务器安装
- 中国联通:企业级ICT转型与创新实践
- C#图形图像编程深入解析:GDI+与多媒体应用
- Xilinx AXI Interconnect v2.1用户指南
- DIY编程电缆全攻略:接口类型与自制指南
- 电脑维护与硬盘数据恢复指南
- 计算机网络技术专业剖析:人才培养与改革
- 量化多因子指数增强策略:微观视角的实证分析

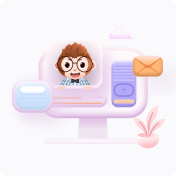
