没有合适的资源?快使用搜索试试~ 我知道了~
首页ClickHouse:高性能列式数据库管理系统详解
ClickHouse:高性能列式数据库管理系统详解
需积分: 10 1 下载量 94 浏览量
更新于2024-07-09
收藏 7.94MB PDF 举报
"clickhouse_zh.pdf"
ClickHouse是专为联机分析(OLAP)设计的列式数据库管理系统(DBMS),其主要目的是处理大规模数据的快速分析和查询。相较于传统的行式数据库系统,如MySQL、Postgres和MSSQL Server,ClickHouse在处理大量数据分析时表现出更高的性能和效率。
在行式数据库中,数据按照记录的顺序存储,每一行包含所有列的数据,例如在上述例子中,每一行包含了WatchID、JavaEnable、Title、GoodEvent和EventTime等字段。这样的存储方式适合频繁对整行数据进行增删改查的操作,因为读取或修改一行数据时,可以一次性获取或修改所有列。
然而,在列式数据库系统中,如ClickHouse、Vertica、Amazon Redshift等,数据按照列来组织,同一列的数据被物理地存储在一起。这种结构优化了对大量数据的分析查询,因为在OLAP场景下,通常只需要处理部分列,而无需遍历整个行。例如,在上述列式存储的例子中,WatchID、JavaEnable等列的值分别独立存储,便于快速聚合或筛选特定列的数据。列式存储减少了不必要的I/O操作,提高了查询速度,尤其在进行大数据分析时优势明显。
选择行式还是列式数据库,需要根据实际的业务需求来决定。考虑的因素包括但不限于:查询类型(选择性查询、聚合查询等)、查询频率、数据读取与更新的比例、数据集大小、是否需要事务支持、数据复制策略、数据完整性要求,以及对延迟和吞吐量的需求。在高负载的系统中,列式数据库如ClickHouse能更好地处理大量并发查询,提供高效的分析能力。
ClickHouse的设计还支持分布式处理,可以扩展到多台服务器,通过并行计算进一步提升查询性能。此外,它支持多种数据类型,包括数值、字符串、日期时间等,并且提供了丰富的SQL接口,使得用户能够方便地进行数据导入、查询和管理。
ClickHouse是针对大数据分析需求而设计的高效列式数据库,它的出现为企业的大数据分析工作提供了强大的工具,尤其适合实时或近实时的分析任务,帮助企业快速洞察业务数据,驱动业务决策。
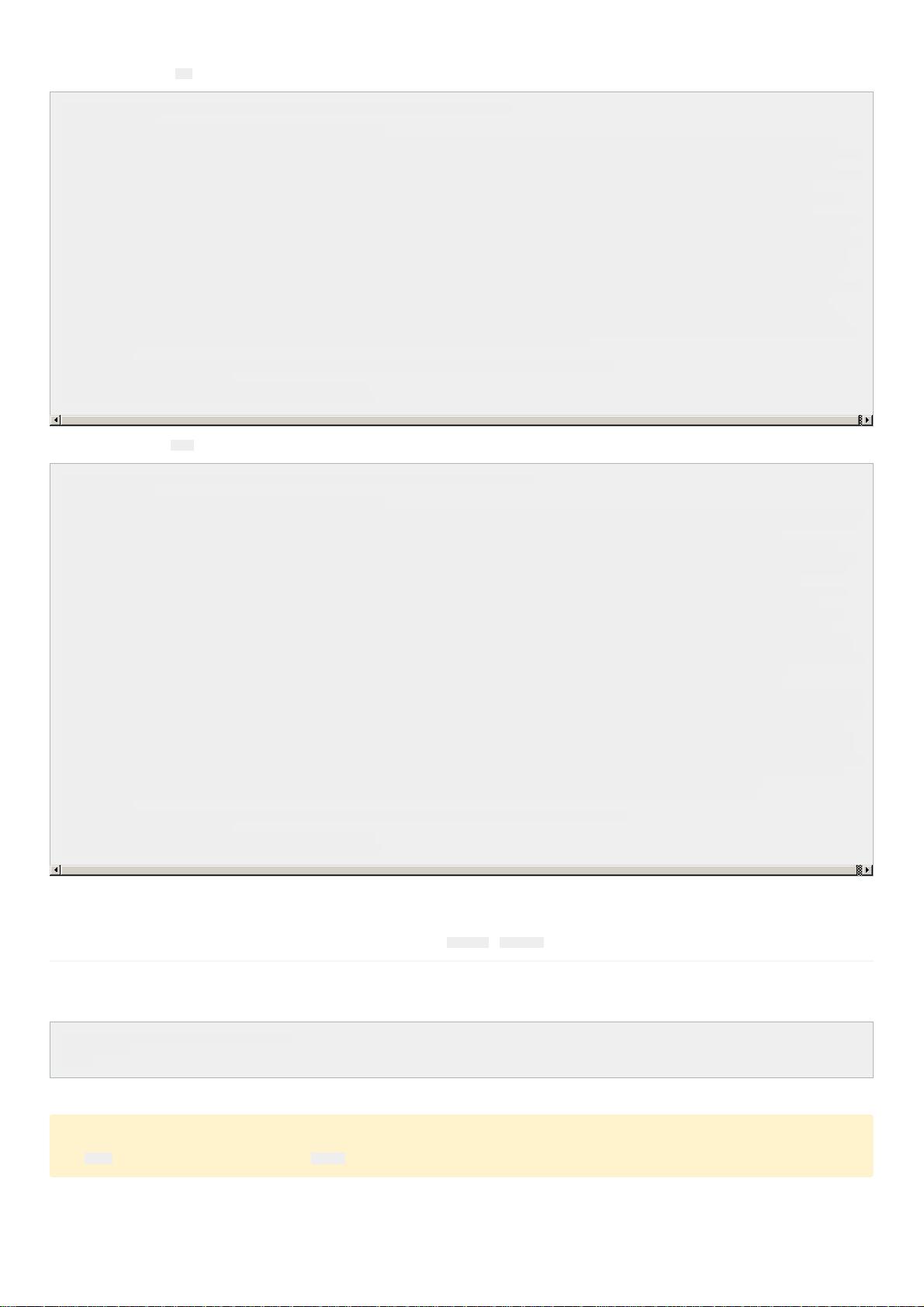
从TSV压缩文件获取表
从TSV压缩文件下载并导入hits:
curl https://datasets.clickhouse.tech/hits/tsv/hits_v1.tsv.xz | unxz --threads=`nproc` > hits_v1.tsv
## now create table
clickhouse-client --query "CREATE DATABASE IF NOT EXISTS datasets"
clickhouse-client --query "CREATE TABLE datasets.hits_v1 ( WatchID UInt64, JavaEnable UInt8, Title String, GoodEvent Int16, EventTime DateTime, EventDate Date,
CounterID UInt32, ClientIP UInt32, ClientIP6 FixedString(16), RegionID UInt32, UserID UInt64, CounterClass Int8, OS UInt8, UserAgent UInt8, URL String, Referer String,
URLDomain String, RefererDomain String, Refresh UInt8, IsRobot UInt8, RefererCategories Array(UInt16), URLCategories Array(UInt16), URLRegions Array(UInt32),
RefererRegions Array(UInt32), ResolutionWidth UInt16, ResolutionHeight UInt16, ResolutionDepth UInt8, FlashMajor UInt8, FlashMinor UInt8, FlashMinor2 String, NetMajor
UInt8, NetMinor UInt8, UserAgentMajor UInt16, UserAgentMinor FixedString(2), CookieEnable UInt8, JavascriptEnable UInt8, IsMobile UInt8, MobilePhone UInt8,
MobilePhoneModel String, Params String, IPNetworkID UInt32, TraficSourceID Int8, SearchEngineID UInt16, SearchPhrase String, AdvEngineID UInt8, IsArtifical UInt8,
WindowClientWidth UInt16, WindowClientHeight UInt16, ClientTimeZone Int16, ClientEventTime DateTime, SilverlightVersion1 UInt8, SilverlightVersion2 UInt8,
SilverlightVersion3 UInt32, SilverlightVersion4 UInt16, PageCharset String, CodeVersion UInt32, IsLink UInt8, IsDownload UInt8, IsNotBounce UInt8, FUniqID UInt64, HID
UInt32, IsOldCounter UInt8, IsEvent UInt8, IsParameter UInt8, DontCountHits UInt8, WithHash UInt8, HitColor FixedString(1), UTCEventTime DateTime, Age UInt8, Sex
UInt8, Income UInt8, Interests UInt16, Robotness UInt8, GeneralInterests Array(UInt16), RemoteIP UInt32, RemoteIP6 FixedString(16), WindowName Int32, OpenerName
Int32, HistoryLength Int16, BrowserLanguage FixedString(2), BrowserCountry FixedString(2), SocialNetwork String, SocialAction String, HTTPError UInt16, SendTiming
Int32, DNSTiming Int32, ConnectTiming Int32, ResponseStartTiming Int32, ResponseEndTiming Int32, FetchTiming Int32, RedirectTiming Int32, DOMInteractiveTiming
Int32, DOMContentLoadedTiming Int32, DOMCompleteTiming Int32, LoadEventStartTiming Int32, LoadEventEndTiming Int32, NSToDOMContentLoadedTiming Int32,
FirstPaintTiming Int32, RedirectCount Int8, SocialSourceNetworkID UInt8, SocialSourcePage String, ParamPrice Int64, ParamOrderID String, ParamCurrency FixedString(3),
ParamCurrencyID UInt16, GoalsReached Array(UInt32), OpenstatServiceName String, OpenstatCampaignID String, OpenstatAdID String, OpenstatSourceID String,
UTMSource String, UTMMedium String, UTMCampaign String, UTMContent String, UTMTerm String, FromTag String, HasGCLID UInt8, RefererHash UInt64, URLHash
UInt64, CLID UInt32, YCLID UInt64, ShareService String, ShareURL String, ShareTitle String, ParsedParams Nested(Key1 String, Key2 String, Key3 String, Key4 String,
Key5 String, ValueDouble Float64), IslandID FixedString(16), RequestNum UInt32, RequestTry UInt8) ENGINE = MergeTree() PARTITION BY toYYYYMM(EventDate) ORDER
BY (CounterID, EventDate, intHash32(UserID)) SAMPLE BY intHash32(UserID) SETTINGS index_granularity = 8192"
## import data
cat hits_v1.tsv | clickhouse-client --query "INSERT INTO datasets.hits_v1 FORMAT TSV" --max_insert_block_size=100000
## optionally you can optimize table
clickhouse-client --query "OPTIMIZE TABLE datasets.hits_v1 FINAL"
clickhouse-client --query "SELECT COUNT(*) FROM datasets.hits_v1"
从压缩tsv文件下载和导入visits:
curl https://datasets.clickhouse.tech/visits/tsv/visits_v1.tsv.xz | unxz --threads=`nproc` > visits_v1.tsv
## now create table
clickhouse-client --query "CREATE DATABASE IF NOT EXISTS datasets"
clickhouse-client --query "CREATE TABLE datasets.visits_v1 ( CounterID UInt32, StartDate Date, Sign Int8, IsNew UInt8, VisitID UInt64, UserID UInt64, StartTime DateTime,
Duration UInt32, UTCStartTime DateTime, PageViews Int32, Hits Int32, IsBounce UInt8, Referer String, StartURL String, RefererDomain String, StartURLDomain String,
EndURL String, LinkURL String, IsDownload UInt8, TraficSourceID Int8, SearchEngineID UInt16, SearchPhrase String, AdvEngineID UInt8, PlaceID Int32,
RefererCategories Array(UInt16), URLCategories Array(UInt16), URLRegions Array(UInt32), RefererRegions Array(UInt32), IsYandex UInt8, GoalReachesDepth Int32,
GoalReachesURL Int32, GoalReachesAny Int32, SocialSourceNetworkID UInt8, SocialSourcePage String, MobilePhoneModel String, ClientEventTime DateTime, RegionID
UInt32, ClientIP UInt32, ClientIP6 FixedString(16), RemoteIP UInt32, RemoteIP6 FixedString(16), IPNetworkID UInt32, SilverlightVersion3 UInt32, CodeVersion UInt32,
ResolutionWidth UInt16, ResolutionHeight UInt16, UserAgentMajor UInt16, UserAgentMinor UInt16, WindowClientWidth UInt16, WindowClientHeight UInt16,
SilverlightVersion2 UInt8, SilverlightVersion4 UInt16, FlashVersion3 UInt16, FlashVersion4 UInt16, ClientTimeZone Int16, OS UInt8, UserAgent UInt8, ResolutionDepth
UInt8, FlashMajor UInt8, FlashMinor UInt8, NetMajor UInt8, NetMinor UInt8, MobilePhone UInt8, SilverlightVersion1 UInt8, Age UInt8, Sex UInt8, Income UInt8,
JavaEnable UInt8, CookieEnable UInt8, JavascriptEnable UInt8, IsMobile UInt8, BrowserLanguage UInt16, BrowserCountry UInt16, Interests UInt16, Robotness UInt8,
GeneralInterests Array(UInt16), Params Array(String), Goals Nested(ID UInt32, Serial UInt32, EventTime DateTime, Price Int64, OrderID String, CurrencyID UInt32),
WatchIDs Array(UInt64), ParamSumPrice Int64, ParamCurrency FixedString(3), ParamCurrencyID UInt16, ClickLogID UInt64, ClickEventID Int32, ClickGoodEvent Int32,
ClickEventTime DateTime, ClickPriorityID Int32, ClickPhraseID Int32, ClickPageID Int32, ClickPlaceID Int32, ClickTypeID Int32, ClickResourceID Int32, ClickCost UInt32,
ClickClientIP UInt32, ClickDomainID UInt32, ClickURL String, ClickAttempt UInt8, ClickOrderID UInt32, ClickBannerID UInt32, ClickMarketCategoryID UInt32, ClickMarketPP
UInt32, ClickMarketCategoryName String, ClickMarketPPName String, ClickAWAPSCampaignName String, ClickPageName String, ClickTargetType UInt16,
ClickTargetPhraseID UInt64, ClickContextType UInt8, ClickSelectType Int8, ClickOptions String, ClickGroupBannerID Int32, OpenstatServiceName String,
OpenstatCampaignID String, OpenstatAdID String, OpenstatSourceID String, UTMSource String, UTMMedium String, UTMCampaign String, UTMContent String, UTMTerm
String, FromTag String, HasGCLID UInt8, FirstVisit DateTime, PredLastVisit Date, LastVisit Date, TotalVisits UInt32, TraficSource Nested(ID Int8, SearchEngineID UInt16,
AdvEngineID UInt8, PlaceID UInt16, SocialSourceNetworkID UInt8, Domain String, SearchPhrase String, SocialSourcePage String), Attendance FixedString(16), CLID UInt32,
YCLID UInt64, NormalizedRefererHash UInt64, SearchPhraseHash UInt64, RefererDomainHash UInt64, NormalizedStartURLHash UInt64, StartURLDomainHash UInt64,
NormalizedEndURLHash UInt64, TopLevelDomain UInt64, URLScheme UInt64, OpenstatServiceNameHash UInt64, OpenstatCampaignIDHash UInt64, OpenstatAdIDHash
UInt64, OpenstatSourceIDHash UInt64, UTMSourceHash UInt64, UTMMediumHash UInt64, UTMCampaignHash UInt64, UTMContentHash UInt64, UTMTermHash UInt64,
FromHash UInt64, WebVisorEnabled UInt8, WebVisorActivity UInt32, ParsedParams Nested(Key1 String, Key2 String, Key3 String, Key4 String, Key5 String, ValueDouble
Float64), Market Nested(Type UInt8, GoalID UInt32, OrderID String, OrderPrice Int64, PP UInt32, DirectPlaceID UInt32, DirectOrderID UInt32, DirectBannerID UInt32,
GoodID String, GoodName String, GoodQuantity Int32, GoodPrice Int64), IslandID FixedString(16)) ENGINE = CollapsingMergeTree(Sign) PARTITION BY
toYYYYMM(StartDate) ORDER BY (CounterID, StartDate, intHash32(UserID), VisitID) SAMPLE BY intHash32(UserID) SETTINGS index_granularity = 8192"
## import data
cat visits_v1.tsv | clickhouse-client --query "INSERT INTO datasets.visits_v1 FORMAT TSV" --max_insert_block_size=100000
## optionally you can optimize table
clickhouse-client --query "OPTIMIZE TABLE datasets.visits_v1 FINAL"
clickhouse-client --query "SELECT COUNT(*) FROM datasets.visits_v1"
查询示例
使用教程是以Yandex.Metrica数据集开始教程。
可以在ClickHouse的stateful tests 中找到对这些表的查询的其他示例(它们被命名为test.hists和test.visits)。
Star Schema Benchmark
编译 dbgen:
$ git clone git@github.com:vadimtk/ssb-dbgen.git
$ cd ssb-dbgen
$ make
开始生成数据:
注意
使用-s 100dbgen将生成6亿行数据(67GB), 如果使用-s 1000它会生成60亿行数据(这需要很多时间))
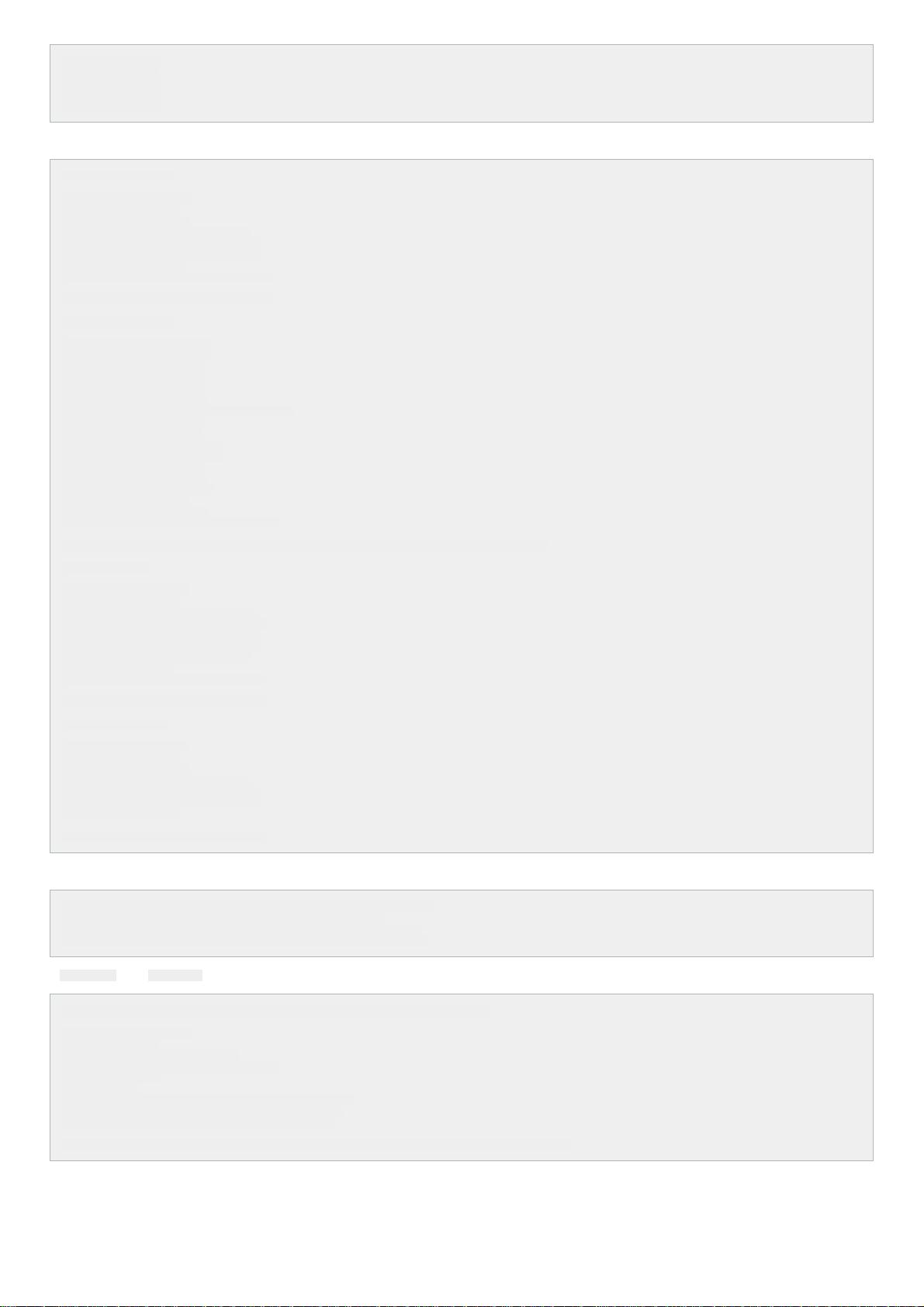
$ ./dbgen -s 1000 -T c
$ ./dbgen -s 1000 -T l
$ ./dbgen -s 1000 -T p
$ ./dbgen -s 1000 -T s
$ ./dbgen -s 1000 -T d
在ClickHouse中创建数据表:
CREATE TABLE customer
(
C_CUSTKEY UInt32,
C_NAME String,
C_ADDRESS String,
C_CITY LowCardinality(String),
C_NATION LowCardinality(String),
C_REGION LowCardinality(String),
C_PHONE String,
C_MKTSEGMENT LowCardinality(String)
)
ENGINE = MergeTree ORDER BY (C_CUSTKEY);
CREATE TABLE lineorder
(
LO_ORDERKEY UInt32,
LO_LINENUMBER UInt8,
LO_CUSTKEY UInt32,
LO_PARTKEY UInt32,
LO_SUPPKEY UInt32,
LO_ORDERDATE Date,
LO_ORDERPRIORITY LowCardinality(String),
LO_SHIPPRIORITY UInt8,
LO_QUANTITY UInt8,
LO_EXTENDEDPRICE UInt32,
LO_ORDTOTALPRICE UInt32,
LO_DISCOUNT UInt8,
LO_REVENUE UInt32,
LO_SUPPLYCOST UInt32,
LO_TAX UInt8,
LO_COMMITDATE Date,
LO_SHIPMODE LowCardinality(String)
)
ENGINE = MergeTree PARTITION BY toYear(LO_ORDERDATE) ORDER BY (LO_ORDERDATE, LO_ORDERKEY);
CREATE TABLE part
(
P_PARTKEY UInt32,
P_NAME String,
P_MFGR LowCardinality(String),
P_CATEGORY LowCardinality(String),
P_BRAND LowCardinality(String),
P_COLOR LowCardinality(String),
P_TYPE LowCardinality(String),
P_SIZE UInt8,
P_CONTAINER LowCardinality(String)
)
ENGINE = MergeTree ORDER BY P_PARTKEY;
CREATE TABLE supplier
(
S_SUPPKEY UInt32,
S_NAME String,
S_ADDRESS String,
S_CITY LowCardinality(String),
S_NATION LowCardinality(String),
S_REGION LowCardinality(String),
S_PHONE String
)
ENGINE = MergeTree ORDER BY S_SUPPKEY;
写入数据:
$ clickhouse-client --query "INSERT INTO customer FORMAT CSV" < customer.tbl
$ clickhouse-client --query "INSERT INTO part FORMAT CSV" < part.tbl
$ clickhouse-client --query "INSERT INTO supplier FORMAT CSV" < supplier.tbl
$ clickhouse-client --query "INSERT INTO lineorder FORMAT CSV" < lineorder.tbl
将star schema转换为flat schema:
SET max_memory_usage = 20000000000, allow_experimental_multiple_joins_emulation = 1;
CREATE TABLE lineorder_flat
ENGINE = MergeTree
PARTITION BY toYear(LO_ORDERDATE)
ORDER BY (LO_ORDERDATE, LO_ORDERKEY) AS
SELECT l.*, c.*, s.*, p.*
FROM lineorder l
ANY INNER JOIN customer c ON (c.C_CUSTKEY = l.LO_CUSTKEY)
ANY INNER JOIN supplier s ON (s.S_SUPPKEY = l.LO_SUPPKEY)
ANY INNER JOIN part p ON (p.P_PARTKEY = l.LO_PARTKEY);
ALTER TABLE lineorder_flat DROP COLUMN C_CUSTKEY, DROP COLUMN S_SUPPKEY, DROP COLUMN P_PARTKEY;
运行查询:
Q1.1
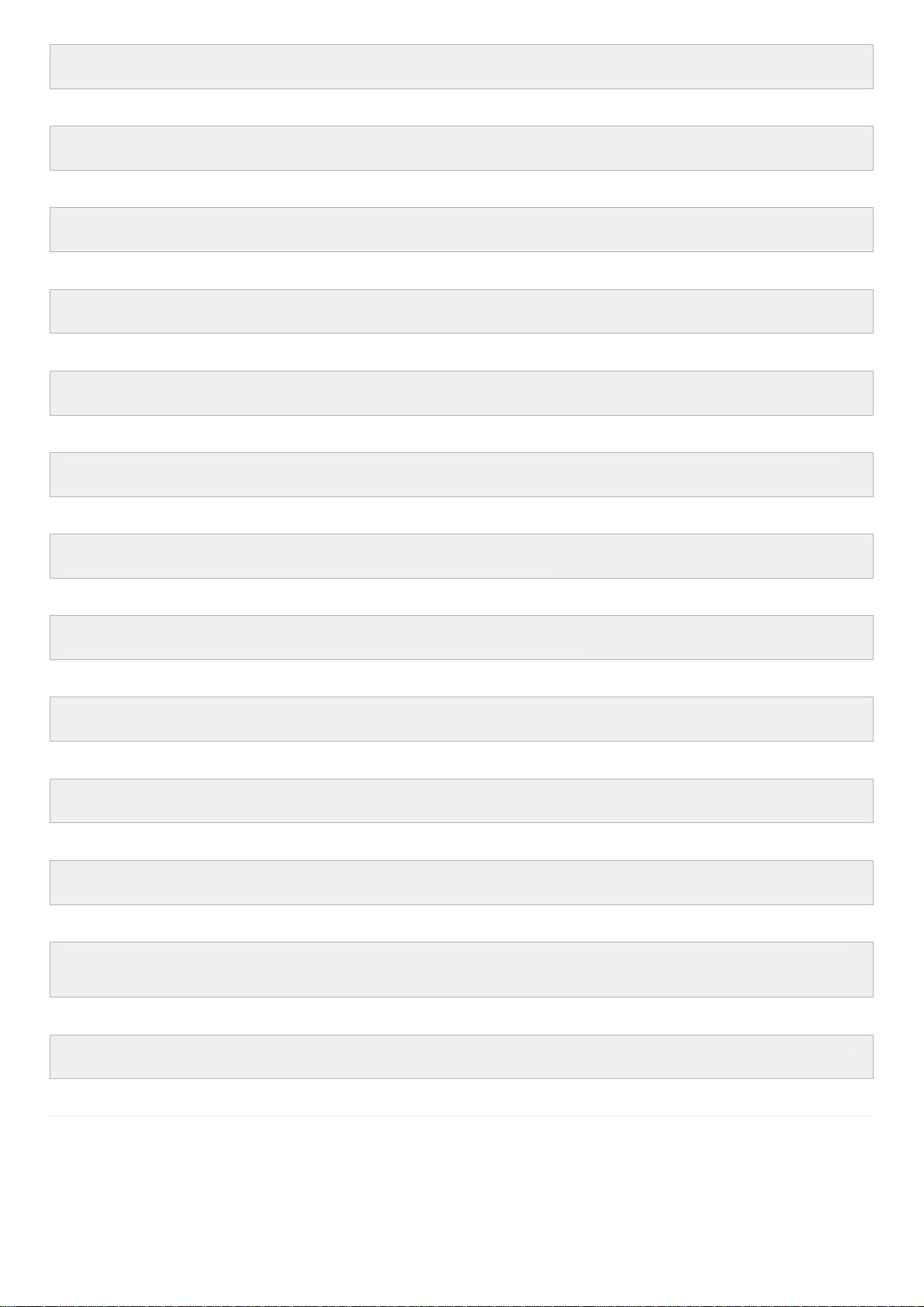
SELECT sum(LO_EXTENDEDPRICE * LO_DISCOUNT) AS revenue FROM lineorder_flat WHERE toYear(LO_ORDERDATE) = 1993 AND LO_DISCOUNT BETWEEN 1 AND 3 AND
LO_QUANTITY < 25;
Q1.2
SELECT sum(LO_EXTENDEDPRICE * LO_DISCOUNT) AS revenue FROM lineorder_flat WHERE toYYYYMM(LO_ORDERDATE) = 199401 AND LO_DISCOUNT BETWEEN 4 AND 6 AND
LO_QUANTITY BETWEEN 26 AND 35;
Q1.3
SELECT sum(LO_EXTENDEDPRICE * LO_DISCOUNT) AS revenue FROM lineorder_flat WHERE toISOWeek(LO_ORDERDATE) = 6 AND toYear(LO_ORDERDATE) = 1994 AND
LO_DISCOUNT BETWEEN 5 AND 7 AND LO_QUANTITY BETWEEN 26 AND 35;
Q2.1
SELECT sum(LO_REVENUE), toYear(LO_ORDERDATE) AS year, P_BRAND FROM lineorder_flat WHERE P_CATEGORY = 'MFGR#12' AND S_REGION = 'AMERICA' GROUP BY year,
P_BRAND ORDER BY year, P_BRAND;
Q2.2
SELECT sum(LO_REVENUE), toYear(LO_ORDERDATE) AS year, P_BRAND FROM lineorder_flat WHERE P_BRAND BETWEEN 'MFGR#2221' AND 'MFGR#2228' AND S_REGION =
'ASIA' GROUP BY year, P_BRAND ORDER BY year, P_BRAND;
Q2.3
SELECT sum(LO_REVENUE), toYear(LO_ORDERDATE) AS year, P_BRAND FROM lineorder_flat WHERE P_BRAND = 'MFGR#2239' AND S_REGION = 'EUROPE' GROUP BY year,
P_BRAND ORDER BY year, P_BRAND;
Q3.1
SELECT C_NATION, S_NATION, toYear(LO_ORDERDATE) AS year, sum(LO_REVENUE) AS revenue FROM lineorder_flat WHERE C_REGION = 'ASIA' AND S_REGION = 'ASIA' AND
year >= 1992 AND year <= 1997 GROUP BY C_NATION, S_NATION, year ORDER BY year asc, revenue desc;
Q3.2
SELECT C_CITY, S_CITY, toYear(LO_ORDERDATE) AS year, sum(LO_REVENUE) AS revenue FROM lineorder_flat WHERE C_NATION = 'UNITED STATES' AND S_NATION = 'UNITED
STATES' AND year >= 1992 AND year <= 1997 GROUP BY C_CITY, S_CITY, year ORDER BY year asc, revenue desc;
Q3.3
SELECT C_CITY, S_CITY, toYear(LO_ORDERDATE) AS year, sum(LO_REVENUE) AS revenue FROM lineorder_flat WHERE (C_CITY = 'UNITED KI1' OR C_CITY = 'UNITED KI5') AND
(S_CITY = 'UNITED KI1' OR S_CITY = 'UNITED KI5') AND year >= 1992 AND year <= 1997 GROUP BY C_CITY, S_CITY, year ORDER BY year asc, revenue desc;
Q3.4
SELECT C_CITY, S_CITY, toYear(LO_ORDERDATE) AS year, sum(LO_REVENUE) AS revenue FROM lineorder_flat WHERE (C_CITY = 'UNITED KI1' OR C_CITY = 'UNITED KI5') AND
(S_CITY = 'UNITED KI1' OR S_CITY = 'UNITED KI5') AND toYYYYMM(LO_ORDERDATE) = '199712' GROUP BY C_CITY, S_CITY, year ORDER BY year asc, revenue desc;
Q4.1
SELECT toYear(LO_ORDERDATE) AS year, C_NATION, sum(LO_REVENUE - LO_SUPPLYCOST) AS profit FROM lineorder_flat WHERE C_REGION = 'AMERICA' AND S_REGION =
'AMERICA' AND (P_MFGR = 'MFGR#1' OR P_MFGR = 'MFGR#2') GROUP BY year, C_NATION ORDER BY year, C_NATION;
Q4.2
SELECT toYear(LO_ORDERDATE) AS year, S_NATION, P_CATEGORY, sum(LO_REVENUE - LO_SUPPLYCOST) AS profit FROM lineorder_flat WHERE C_REGION = 'AMERICA' AND
S_REGION = 'AMERICA' AND (year = 1997 OR year = 1998) AND (P_MFGR = 'MFGR#1' OR P_MFGR = 'MFGR#2') GROUP BY year, S_NATION, P_CATEGORY ORDER BY year,
S_NATION, P_CATEGORY;
Q4.3
SELECT toYear(LO_ORDERDATE) AS year, S_CITY, P_BRAND, sum(LO_REVENUE - LO_SUPPLYCOST) AS profit FROM lineorder_flat WHERE S_NATION = 'UNITED STATES' AND
(year = 1997 OR year = 1998) AND P_CATEGORY = 'MFGR#14' GROUP BY year, S_CITY, P_BRAND ORDER BY year, S_CITY, P_BRAND;
原始文章
WikiStat
参考: http://dumps.wikimedia.org/other/pagecounts-raw/
创建表结构:
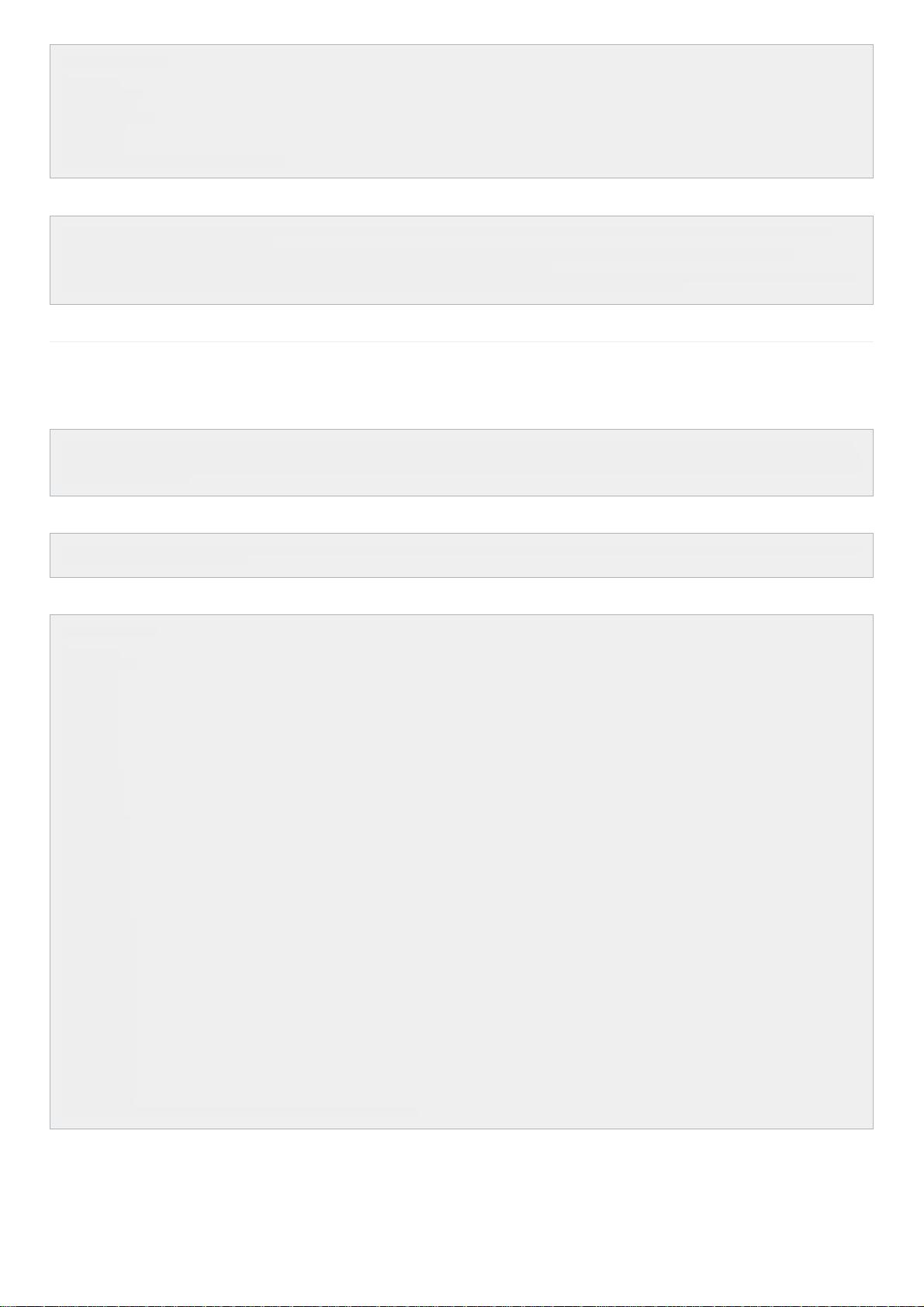
CREATE TABLE wikistat
(
date Date,
time DateTime,
project String,
subproject String,
path String,
hits UInt64,
size UInt64
) ENGINE = MergeTree(date, (path, time), 8192);
加载数据:
$ for i in {2007..2016}; do for j in {01..12}; do echo $i-$j >&2; curl -sSL "http://dumps.wikimedia.org/other/pagecounts-raw/$i/$i-$j/" | grep -oE 'pagecounts-[0-9]+-[0-
9]+\.gz'; done; done | sort | uniq | tee links.txt
$ cat links.txt | while read link; do wget http://dumps.wikimedia.org/other/pagecounts-raw/$(echo $link | sed -r 's/pagecounts-([0-9]{4})([0-9]{2})[0-9]{2}-[0-
9]+\.gz/\1/')/$(echo $link | sed -r 's/pagecounts-([0-9]{4})([0-9]{2})[0-9]{2}-[0-9]+\.gz/\1-\2/')/$link; done
$ ls -1 /opt/wikistat/ | grep gz | while read i; do echo $i; gzip -cd /opt/wikistat/$i | ./wikistat-loader --time="$(echo -n $i | sed -r 's/pagecounts-([0-9]{4})([0-9]{2})([0-9]{2})-
([0-9]{2})([0-9]{2})([0-9]{2})\.gz/\1-\2-\3 \4-00-00/')" | clickhouse-client --query="INSERT INTO wikistat FORMAT TabSeparated"; done
原始文章
Terabyte of Click Logs from Criteo
可以从 http://labs.criteo.com/downloads/download-terabyte-click-logs/ 上下载数据
创建原始数据对应的表结构:
CREATE TABLE criteo_log (date Date, clicked UInt8, int1 Int32, int2 Int32, int3 Int32, int4 Int32, int5 Int32, int6 Int32, int7 Int32, int8 Int32, int9 Int32, int10 Int32, int11 Int32,
int12 Int32, int13 Int32, cat1 String, cat2 String, cat3 String, cat4 String, cat5 String, cat6 String, cat7 String, cat8 String, cat9 String, cat10 String, cat11 String, cat12 String,
cat13 String, cat14 String, cat15 String, cat16 String, cat17 String, cat18 String, cat19 String, cat20 String, cat21 String, cat22 String, cat23 String, cat24 String, cat25 String,
cat26 String) ENGINE = Log
下载数据:
$ for i in {00..23}; do echo $i; zcat datasets/criteo/day_${i#0}.gz | sed -r 's/^/2000-01-'${i/00/24}'\t/' | clickhouse-client --host=example-perftest01j --query="INSERT INTO
criteo_log FORMAT TabSeparated"; done
创建转换后的数据对应的表结构:
CREATE TABLE criteo
(
date Date,
clicked UInt8,
int1 Int32,
int2 Int32,
int3 Int32,
int4 Int32,
int5 Int32,
int6 Int32,
int7 Int32,
int8 Int32,
int9 Int32,
int10 Int32,
int11 Int32,
int12 Int32,
int13 Int32,
icat1 UInt32,
icat2 UInt32,
icat3 UInt32,
icat4 UInt32,
icat5 UInt32,
icat6 UInt32,
icat7 UInt32,
icat8 UInt32,
icat9 UInt32,
icat10 UInt32,
icat11 UInt32,
icat12 UInt32,
icat13 UInt32,
icat14 UInt32,
icat15 UInt32,
icat16 UInt32,
icat17 UInt32,
icat18 UInt32,
icat19 UInt32,
icat20 UInt32,
icat21 UInt32,
icat22 UInt32,
icat23 UInt32,
icat24 UInt32,
icat25 UInt32,
icat26 UInt32
) ENGINE = MergeTree(date, intHash32(icat1), (date, intHash32(icat1)), 8192)
将第一张表中的原始数据转化写入到第二张表中去:
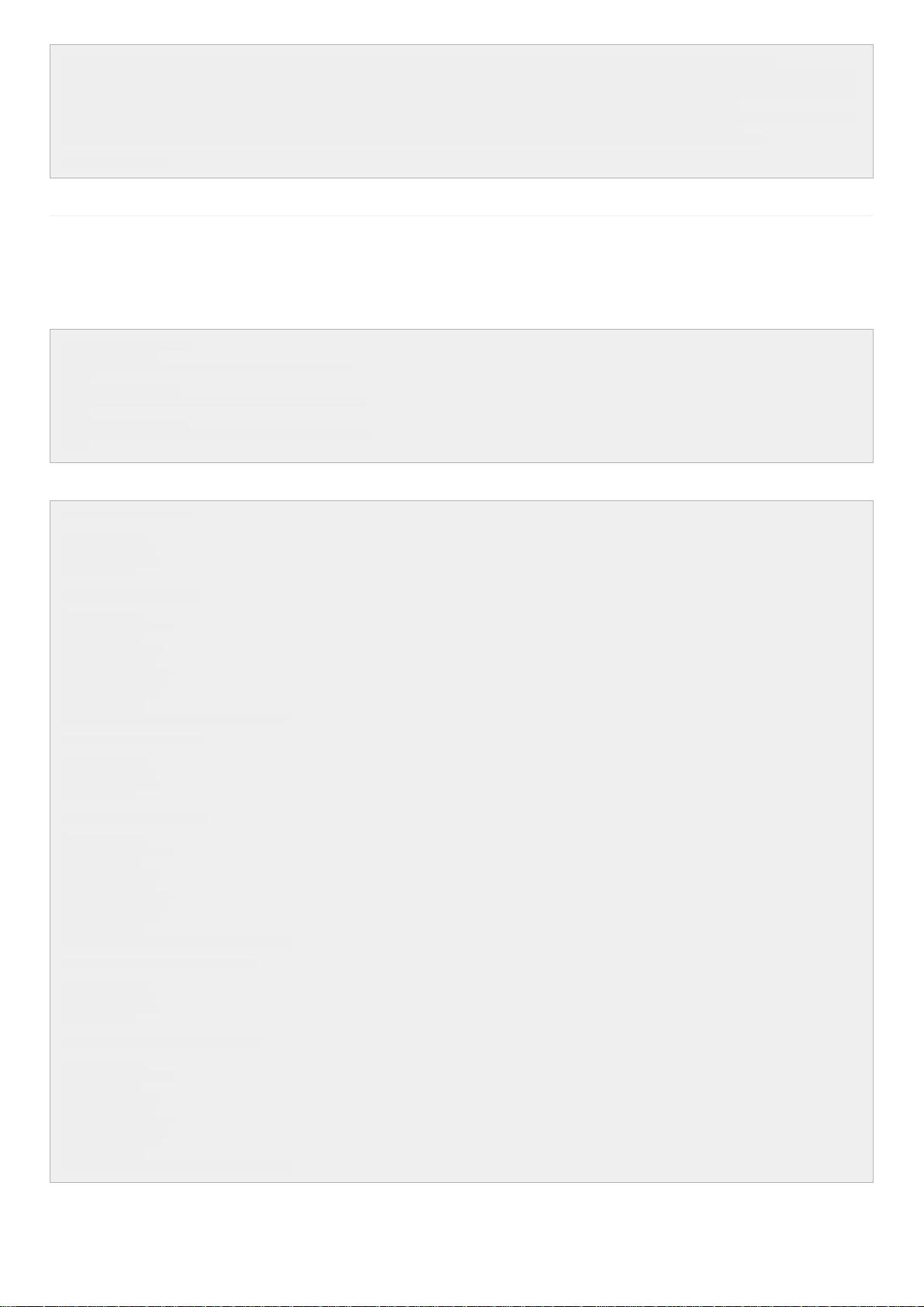
INSERT INTO criteo SELECT date, clicked, int1, int2, int3, int4, int5, int6, int7, int8, int9, int10, int11, int12, int13, reinterpretAsUInt32(unhex(cat1)) AS icat1,
reinterpretAsUInt32(unhex(cat2)) AS icat2, reinterpretAsUInt32(unhex(cat3)) AS icat3, reinterpretAsUInt32(unhex(cat4)) AS icat4, reinterpretAsUInt32(unhex(cat5)) AS icat5,
reinterpretAsUInt32(unhex(cat6)) AS icat6, reinterpretAsUInt32(unhex(cat7)) AS icat7, reinterpretAsUInt32(unhex(cat8)) AS icat8, reinterpretAsUInt32(unhex(cat9)) AS icat9,
reinterpretAsUInt32(unhex(cat10)) AS icat10, reinterpretAsUInt32(unhex(cat11)) AS icat11, reinterpretAsUInt32(unhex(cat12)) AS icat12, reinterpretAsUInt32(unhex(cat13))
AS icat13, reinterpretAsUInt32(unhex(cat14)) AS icat14, reinterpretAsUInt32(unhex(cat15)) AS icat15, reinterpretAsUInt32(unhex(cat16)) AS icat16,
reinterpretAsUInt32(unhex(cat17)) AS icat17, reinterpretAsUInt32(unhex(cat18)) AS icat18, reinterpretAsUInt32(unhex(cat19)) AS icat19, reinterpretAsUInt32(unhex(cat20))
AS icat20, reinterpretAsUInt32(unhex(cat21)) AS icat21, reinterpretAsUInt32(unhex(cat22)) AS icat22, reinterpretAsUInt32(unhex(cat23)) AS icat23,
reinterpretAsUInt32(unhex(cat24)) AS icat24, reinterpretAsUInt32(unhex(cat25)) AS icat25, reinterpretAsUInt32(unhex(cat26)) AS icat26 FROM criteo_log;
DROP TABLE criteo_log;
原始文章
AMPLab Big Data Benchmark
参考 https://amplab.cs.berkeley.edu/benchmark/
需要您在Amazon注册一个免费的账号。注册时需要您提供信用卡、邮箱、电话等信息。之后可以在Amazon AWS Console获取新的访问密钥
在控制台运行以下命令:
$ sudo apt-get install s3cmd
$ mkdir tiny; cd tiny;
$ s3cmd sync s3://big-data-benchmark/pavlo/text-deflate/tiny/ .
$ cd ..
$ mkdir 1node; cd 1node;
$ s3cmd sync s3://big-data-benchmark/pavlo/text-deflate/1node/ .
$ cd ..
$ mkdir 5nodes; cd 5nodes;
$ s3cmd sync s3://big-data-benchmark/pavlo/text-deflate/5nodes/ .
$ cd ..
在ClickHouse运行如下查询:
CREATE TABLE rankings_tiny
(
pageURL String,
pageRank UInt32,
avgDuration UInt32
) ENGINE = Log;
CREATE TABLE uservisits_tiny
(
sourceIP String,
destinationURL String,
visitDate Date,
adRevenue Float32,
UserAgent String,
cCode FixedString(3),
lCode FixedString(6),
searchWord String,
duration UInt32
) ENGINE = MergeTree(visitDate, visitDate, 8192);
CREATE TABLE rankings_1node
(
pageURL String,
pageRank UInt32,
avgDuration UInt32
) ENGINE = Log;
CREATE TABLE uservisits_1node
(
sourceIP String,
destinationURL String,
visitDate Date,
adRevenue Float32,
UserAgent String,
cCode FixedString(3),
lCode FixedString(6),
searchWord String,
duration UInt32
) ENGINE = MergeTree(visitDate, visitDate, 8192);
CREATE TABLE rankings_5nodes_on_single
(
pageURL String,
pageRank UInt32,
avgDuration UInt32
) ENGINE = Log;
CREATE TABLE uservisits_5nodes_on_single
(
sourceIP String,
destinationURL String,
visitDate Date,
adRevenue Float32,
UserAgent String,
cCode FixedString(3),
lCode FixedString(6),
searchWord String,
duration UInt32
) ENGINE = MergeTree(visitDate, visitDate, 8192);
回到控制台运行如下命令:
剩余452页未读,继续阅读
1237 浏览量
2021-01-03 上传
188 浏览量
298 浏览量
138 浏览量
408 浏览量

十元女士
- 粉丝: 2
上传资源 快速赚钱
我的内容管理 展开
我的资源 快来上传第一个资源
我的收益
登录查看自己的收益我的积分 登录查看自己的积分
我的C币 登录后查看C币余额
我的收藏
我的下载
下载帮助

最新资源
- 深入探索Unix/Linux壳脚本编程艺术
- Java面试必备知识点:String、异常处理与集合框架
- 代码托管与平台无关性:IL与Java字节码的比较
- C#实现的在线新华字典系统开发与实现
- 优化Oracle 9i SGA:共享池与librarycache策略
- HTML Meta标签详解与应用
- ATL COM编程经验:ActiveX与接口连接
- ARM汇编详解:六种模式与37个寄存器详解
- C/S模式高校图书管理系统设计——VB+SQLServer实现
- Struts 2实战指南:2008年最新版
- 计算机图形学基础知识与原理详解
- C#编程操作Word指南
- 89.0*90.协议在流媒体传输中的应用
- TestDirector 8.0:Web测试管理系统与Bug管理详解
- Mercury LoadRunner 8.1 教程:性能测试指南
- Boson NetSim 实验指南:静态路由与缺省路由配置
安全验证
文档复制为VIP权益,开通VIP直接复制
