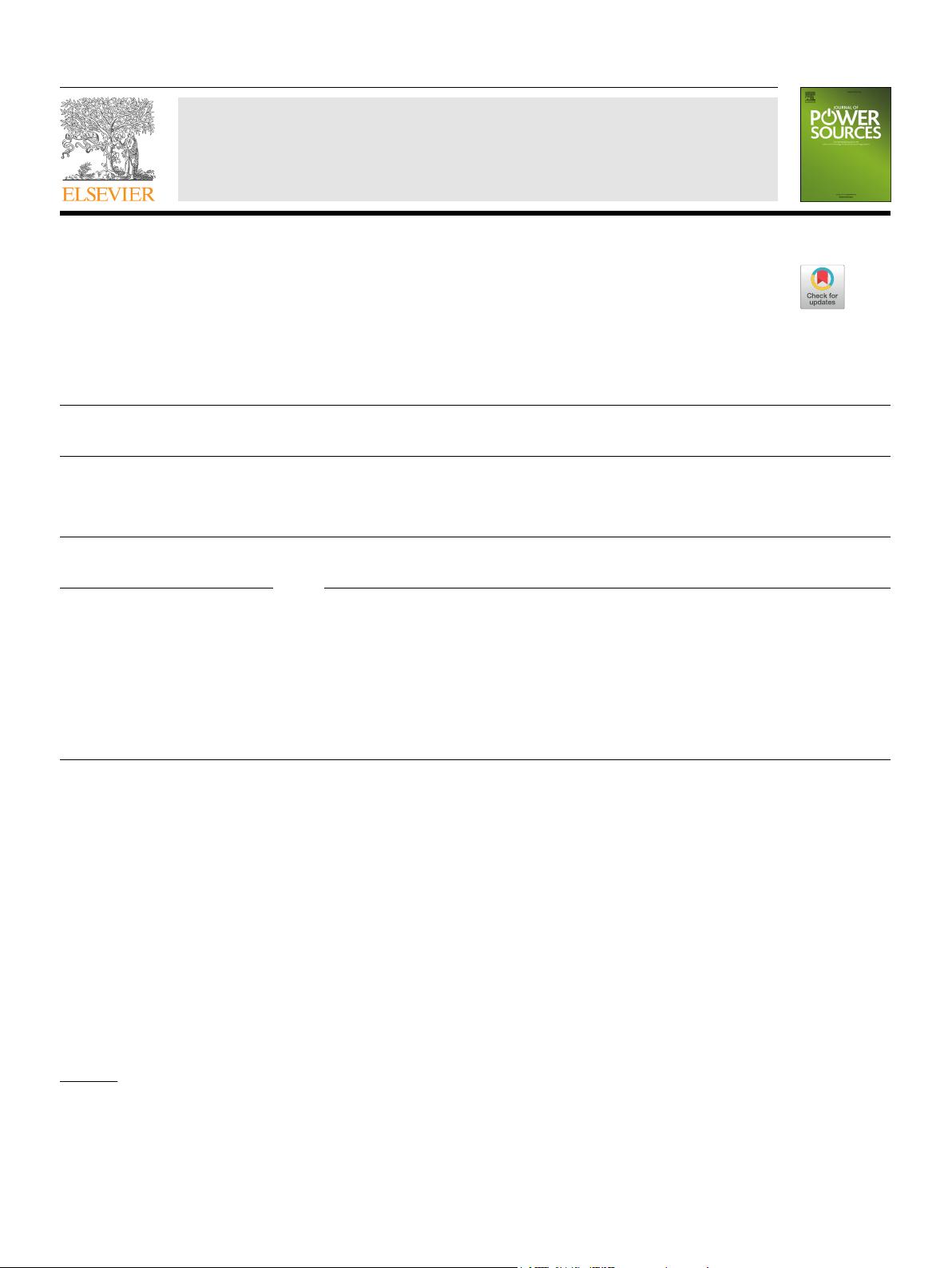
Contents lists available at ScienceDirect
Journal of Power Sources
journa l homepa ge: www.elsevier.com/locate/jpowsour
Short communication
A fast estimation algorithm for lithium-ion battery state of health
Xiaopeng Tang
a
, Changfu Zou
b,∗
, Ke Yao
a,c
, Guohua Chen
a
, Boyang Liu
a
, Zhenwei He
c
,
Furong Gao
a,c,∗∗
a
Department of Chemical and Biological Engineering, Hong Kong University of Science and Technology, Clear Water Bay, Kowloon, Hong Kong
b
Department of Electrical Engineering, Chalmers University of Technology, Gothenburg, 41296, Sweden
c
Guangzhou HKUST Fok Ying Tung Research Institute, Guangzhou, 511458, China
HIGHLIGHTS
•
A new SoH estimation algorithm has been proposed for lithium-ion batteries.
•
Concepts of regional capacity and voltage are introduced based on ICA.
•
SoH models are developed as linear functions of the regional capacity.
•
Experimental results show that the estimation errors are less than 2.5%.
ARTICLE INFO
Keywords:
Lithium-ion batteries
Battery management system
State of health estimation
Incremental capacity analysis
ABSTRACT
This paper proposes a novel and computationally efficient estimation algorithm for lithium-ion battery state of
health (SoH) under the hood of incremental capacity analysis. Concepts of regional capacity and regional voltage
are introduced to develop an SoH model against experimental cycling data from four types of batteries. In the
obtained models, SoH is a simple linear function of the regional capacity, and the R-square of linear fitting is up
to 0.948 for all the considered batteries with properly selected regional voltage. The proposed method without
using characteristic parameters directly from incremental capacity curves is insensitive to noise and filtering
algorithms, and is effective for common current rates, where rates of up to 1C have been demonstrated. Then, a
model-based SoH estimator is designed and shown to be capable of closely matching battery's aging data from
NASA, with the error less than 2.5%. Furthermore, such a small scale of error is achieved in the absent of state of
charge and impedance which are often used for SOH estimation in available methods.
1. Introduction
Lithium-ion (Li-ion) batteries are playing an important role in many
applications, such as electrified transportation and smart electric grids
[1,2]. These batteries undergo a persistent aging process once manu-
factured. If inappropriately utilized due to overcharging, over-dis-
charging, and/or overheating, the battery will be prematurely degraded
or even cause fire and explosion, leading to dramatically deteriorated
state of health (SoH) [3]. It is therefore imperative to monitor the
battery's SoH for safety and health-aware management.
Due to its unmeasurable property, various prediction/estimation
algorithms have been proposed for the SoH of Li-ion batteries. The first
type of algorithms relies on model-based estimation techniques. For
example, physical models have been used for battery health estimation
by quantifying the electrochemical states/parameters, such as the total
number of active lithium, resistance of the solid-electrolyte interface
(SEI) film, and diffusion coefficients [4,5]. In the paradigm of equiva-
lent circuit models, Wei et al. [6] and Hu et al. [7] obtained the SoH
through estimating the capacity and internal resistance. However, the
model-based algorithms are complex, and furthermore, the develop-
ment of a precise and general health model is still ongoing [8,9]. To
sidestep complex battery models, Hung et al. [ 10] explored and
exploited the impedance to reflect SoH. Whereas the impedance ap-
proach can be error-prone as many uncertain factors, including state of
charge (SoC) changes, load conditions, temperature, and measurement
noise, can influence the health estimation results significantly. Data-
driven approaches, such as support vector machine [11] and Gaussian
process regression [12], have also been used to estimate the battery's
health, but are subject to heavy online computations.
Incremental capacity analysis (ICA) has recently emerged to track
https://doi.org/10.1016/j.jpowsour.2018.06.036
Received 9 April 2018; Received in revised form 4 June 2018; Accepted 9 June 2018
∗
Corresponding author. Department of Electrical Engineering, Chalmers University of Technology, Gothenburg, 41296, Sweden.
∗∗
Corresponding author. Department of Chemical and Biological Engineering, Hong Kong University of Science and Technology, Clear Water Bay, Kowloon, Hong Kong.
E-mail addresses: changfu.zou@chalmers.se (C. Zou), kefgao@ust.hk (F. Gao).
Journal of Power Sources 396 (2018) 453–458
0378-7753/ © 2018 Elsevier B.V. All rights reserved.
T