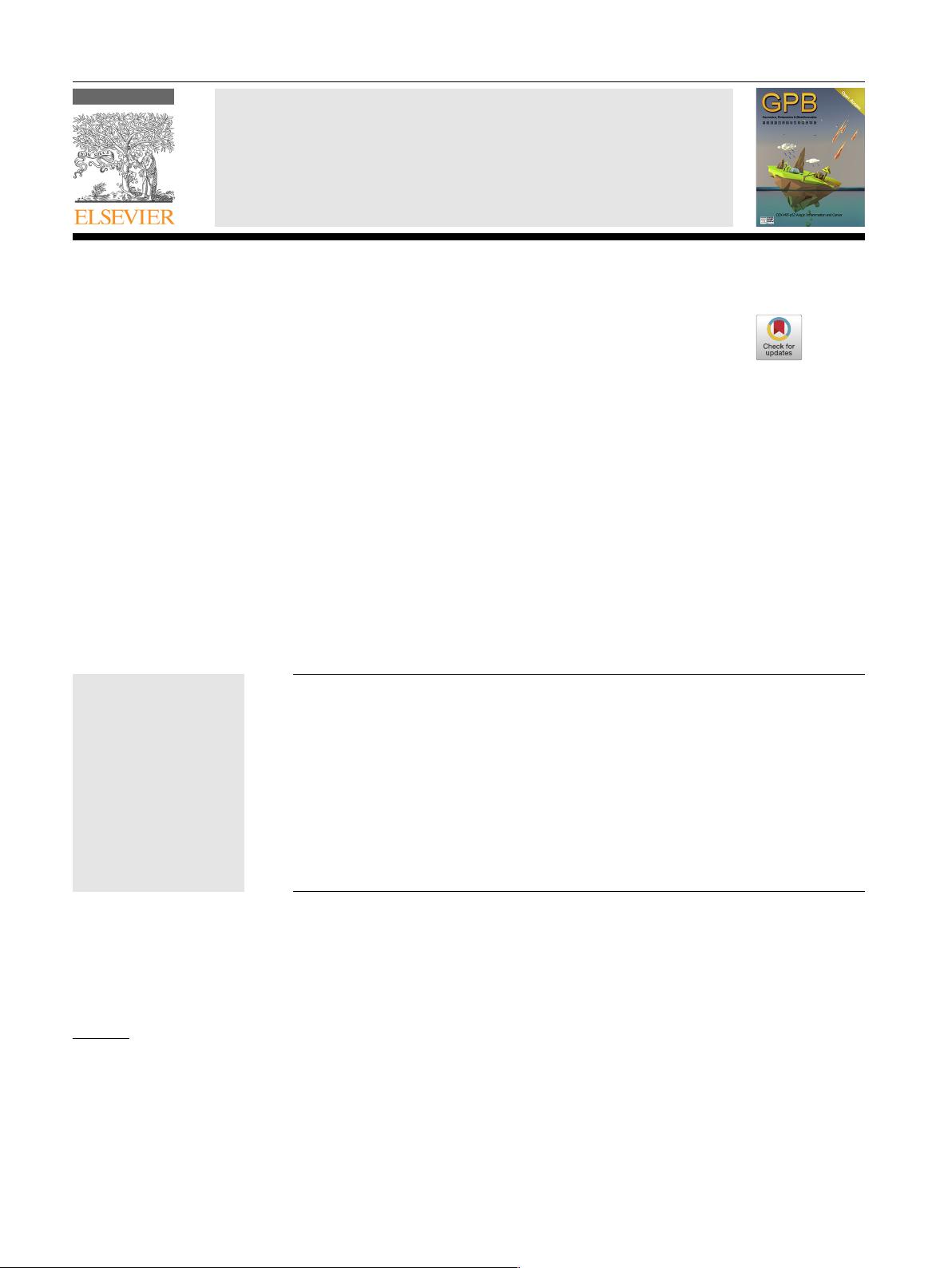
METHOD
Hybrid Method Based on Information Gain
and Support Vector Machine for Gene Selection
in Cancer Classification
Lingyun Gao
a
, Mingquan Ye
*
,b
, Xiaojie Lu
c
, Daobin Huang
d
School of Medical Information, Wannan Medical College, Wuhu 241002, China
Received 12 January 2017; revised 25 July 2017; accepted 8 August 2017
Available online 12 December 2017
Handled by Edwin Wang
KEYWORDS
Gene selection;
Cancer classification;
Information gain;
Support vector machine;
Small sample size with high
dimension
Abstract It remains a great challenge to achieve sufficient cancer classification accuracy with the
entire set of genes, due to the high dimensions, small sample size, and big noise of gene expression
data. We thus proposed a hybrid gene selection method, Information Gain-Support Vector Machine
(IG-SVM) in this study. IG was initially employed to filter irrelevant and redundant genes. Then,
further removal of redundant genes was performed using SVM to eliminate the noise in the datasets
more effectively. Finally, the informative genes selected by IG-SVM served as the input for the
LIBSVM classifier. Compared to other related algorithms, IG-SVM showed the highest classifica-
tion accuracy and superior performance as evaluated using five cancer gene expression datasets
based on a few selected genes. As an example, IG-SVM achieved a classification accuracy of
90.32% for colon cancer, which is difficult to be accurately classified, only based on three genes
including CSRP1 , MYL9, and GUCA2B.
Introduction
The incidence and mortality of cancer have been increasing in
recent years, posing a serious threat to human health. Uncon-
trolled proliferation and metastasis of cancer cells pose chal-
lenges in identification of cancer types. Moreover, most
patients are diagnosed with cancer only when it is at an
advanced stage [1], further increasing the difficulty in cancer
treatment. DNA microarray technology is able to simultane-
ously evaluate the expression levels of numerous genes [2],
enabling the identification of cancer types at the molecular
level. However, the massive amount of data generated and
unavoidable errors occurring during experimental processes
pose great challenges to the analysis of gene expression data.
Gene expression data are featured with high dimensions,
small sample size, and big noise, whereas only a few genes
among the genes examined could play an important role in
cancer prediction [3]. Therefore, various methods had been
developed to select as few informative genes as possible, while
*
Corresponding author.
E-mail: ymq@wnmc.edu.cn (Ye M).
a
ORCID: 0000-0003-2509-9505.
b
ORCID: 0000-0002-0237-4159.
c
ORCID: 0000-0001-5394-1742.
d
ORCID: 0000-0002-5165-7796.
Peer review under responsibility of Beijing Institute of Genomics,
Chinese Academy of Sciences and Genetics Society of China.
Genomics Proteomics Bioinformatics 15 (2017) 389–395
HOSTED BY
Genomics Proteomics Bioinformatics
www.elsevier.com/locate/gpb
www.sciencedirect.com
https://doi.org/10.1016/j.gpb.2017.08.002
1672-0229 Ó 2017 The Authors. Production and hosting by Elsevier B.V. on behalf of Beijing Institute of Genomics, Chinese Academy of Sciences and
Genetics Society of China.
This is an open access article under the CC BY license (http://creativecommons.org/licenses/by/4.0/).