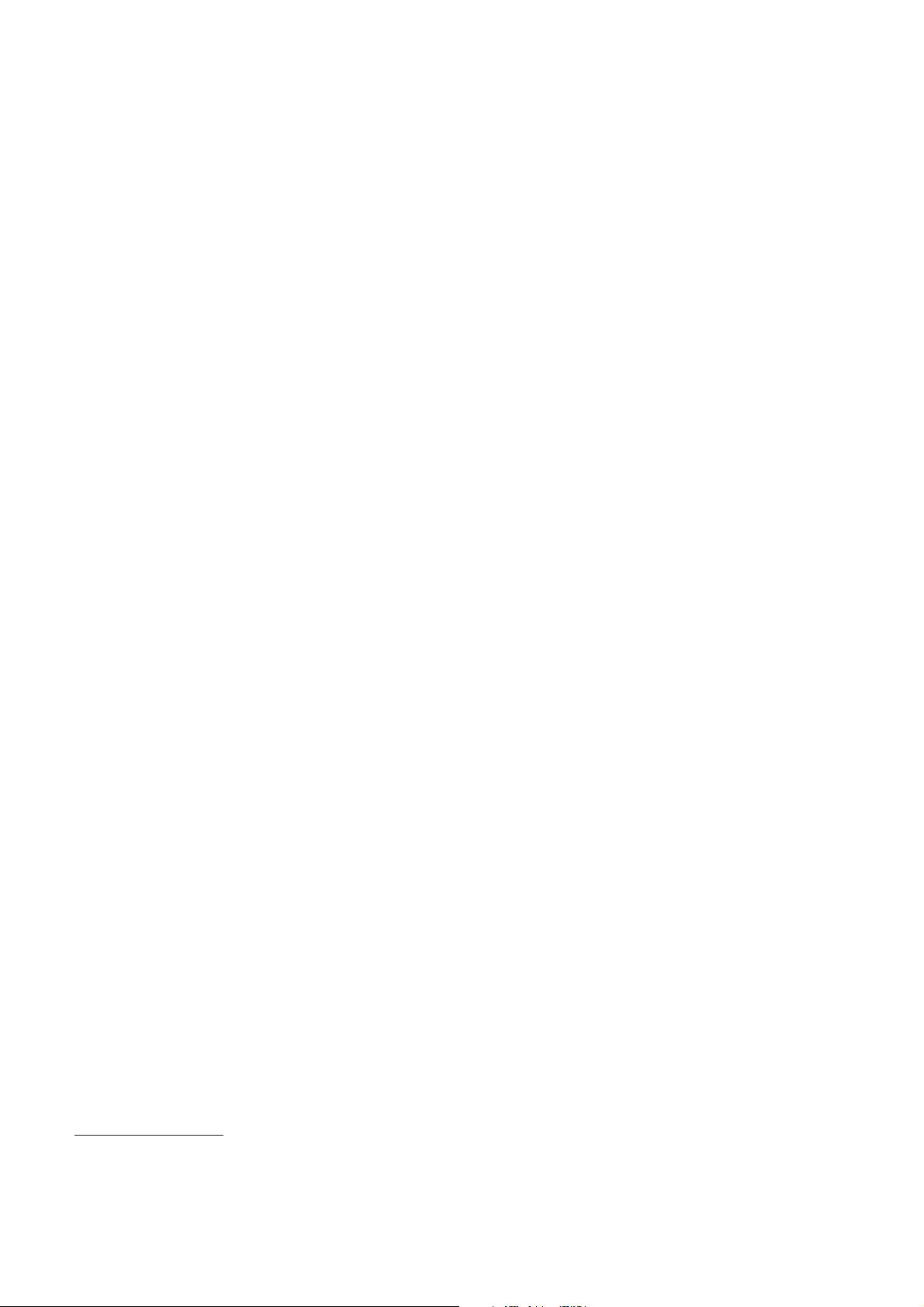
Consensus Analysis of A Long-Range Opinion Model with A
Small Confidence Bound
Jiangbo Zhang
1
, Yiguang Hong
2
1. Jiangbo Zhang is with the faculty of science, Southwest Petroleum University; Chengdu 610500, P. R. China
2. Key Lab of Systems and Control, Academy of Mathematics and Systems Science, Chinese Academy of Sciences, Beijing 100190,
P. R. China,
E-mail: jbzhang@amss.ac.cn; yghong@iss.ac.cn
Abstract: In this paper, we propose a bounded-confidence opinion model and focus on the study of the opinion consensus prob-
ability based on long-range opinion interactions. In this model, each agent updates its opinion using some agents’ opinion values
according to its confidence bound. We provide a lower bound of the opinion consensus probability when the confidence bound
is sufficiently small. Also, we give discussion and simulation examples for the opinion evolution along with some adjustable
parameters.
Key Words: opinion dynamics, consensus probability, confidence bound, long-range interaction
1 Introduction
In recent years, social problems have attracted more and
more attention for both the academy and public, and the
modeling and analysis of social opinion dynamics become
an important theoretical research direction to understand the
underlying social mechanism (referring to [4, 6, 11, 12]).
In light of multi-agent networks, different models for such
problems have been proposed [2, 8, 10, 13, 21], and vari-
ous mathematical methods, such as graph theory, stochastic
process, optimization, and control theory, have been used to
analyze them [18, 22].
As we know, many existing opinion dynamics models il-
lustratively showed how the opinions reach their agreement.
[1, 24]. Based on the zero-entries symmetry of the adjacency
matrices, [5] proved the stability of one class of agent-based
opinion dynamics models, including the discrete-time ho-
mogeneous Hegselmann-Krause (HK) model and the sym-
metric homogeneous Deffuant-Weisbuch (DW) model [19].
Then [6] developed simple methods to show the convergence
of one kind of bound confidence model, while [7] discussed
empirical opinion density using the system’s mean-field dy-
namics as the population size of the agents becomes suffi-
ciently large. From the viewpoint of infinite flow stability of
certain random matrices, [3] provided a new proof about the
convergence of the HK model and gave a convergence ter-
mination time bound for this model. Recently, [16] analyzed
the “Group-think” problem by studying the cooperation of
the appraise dynamics and influence dynamics. [14] stud-
ied some opinion models such as French-DeGroot model,
the linear Abelson model, Friedkin-Johnsen model and Tay-
lor model, with graph-based methods to show how opinion
states evolve in the networks.
Consensus and convergence of multi-agent systems have
been studied widely [9–11, 13, 15]. However, many tech-
niques for the analyze of multi-agent systems (with switch-
ing topologies described by a given random switching signal
This work was supported by the project of Systems science and enter-
prise development, Sichuan Province (Grant No. XQ15B10), the scientific
research starting project of SWPU (Grant No. 2014QHZ032), the NNSF
of China (Grant Nos. 61333001, 61573344), and Beijing Natural Science
Foundation (Grant No. 4152057).
function [12]) cannot apply to the bounded confidence mod-
el, because the switching topologies are state-dependent.
In this paper, we study the consensus probability of one
bounded-confidence opinion model- the Long-range mod-
el [23] with a small confidence bound. The motivation of
the research is to character the consensus probability for a
proposed bounded-confidence opinion model. The key fea-
tures of our model include its bounded confidence restric-
tions (the acceptable degree of the regular agent opinions)
and the multi-selection long-range learning [17]. Although
our model can be viewed as a Markov process, the meth-
ods for graph-based network analysis and stochastic ma-
trix analysis cannot be applied to this long-rang interac-
tion model, because this confidence-based model is state-
dependent and highly nonlinear resulting from the bounded
confidence assigned to each regular agent. Other methods
used in the study of random multi-agent coordination (refer-
ring to [13, 22]) are not effective in our problem, either. The
main contributions in this paper include that we introduce an
bounded confidence opinion model with multiple selection
methods, and provide the estimation for the lower bound for
the probability of opinion consensus in order to measure the
opinions’ aggregation degree, when the confidence bound is
sufficiently small.
The rest of this paper is organized as follows. Section 2 in-
troduces preliminary background and formulates the opinion
model. Section 3 provides the lower bound of agent opinion
limit states’ consensus probability related with some param-
eters and demonstrates the consensus probability when the
confidence bound is sufficiently small. The Section 4 shows
some numerical simulations. Finally, Section 5 gives some
concluding remarks.
Notations: N denotes the non-negative integer number set
{0, 1, 2,...}, while N
+
is the positive integer number set
{1, 2,...}; R is the real number set; R
m×n
means the m×n
matrix with entries taking values in R; 1
m×n
and 0
m×n
de-
note the m × n matrix with all entries being 1 and 0, respec-
tively.
Proceedings of the 36th Chinese Control Conference
Jul
26-28, 2017, Dalian, China
1533