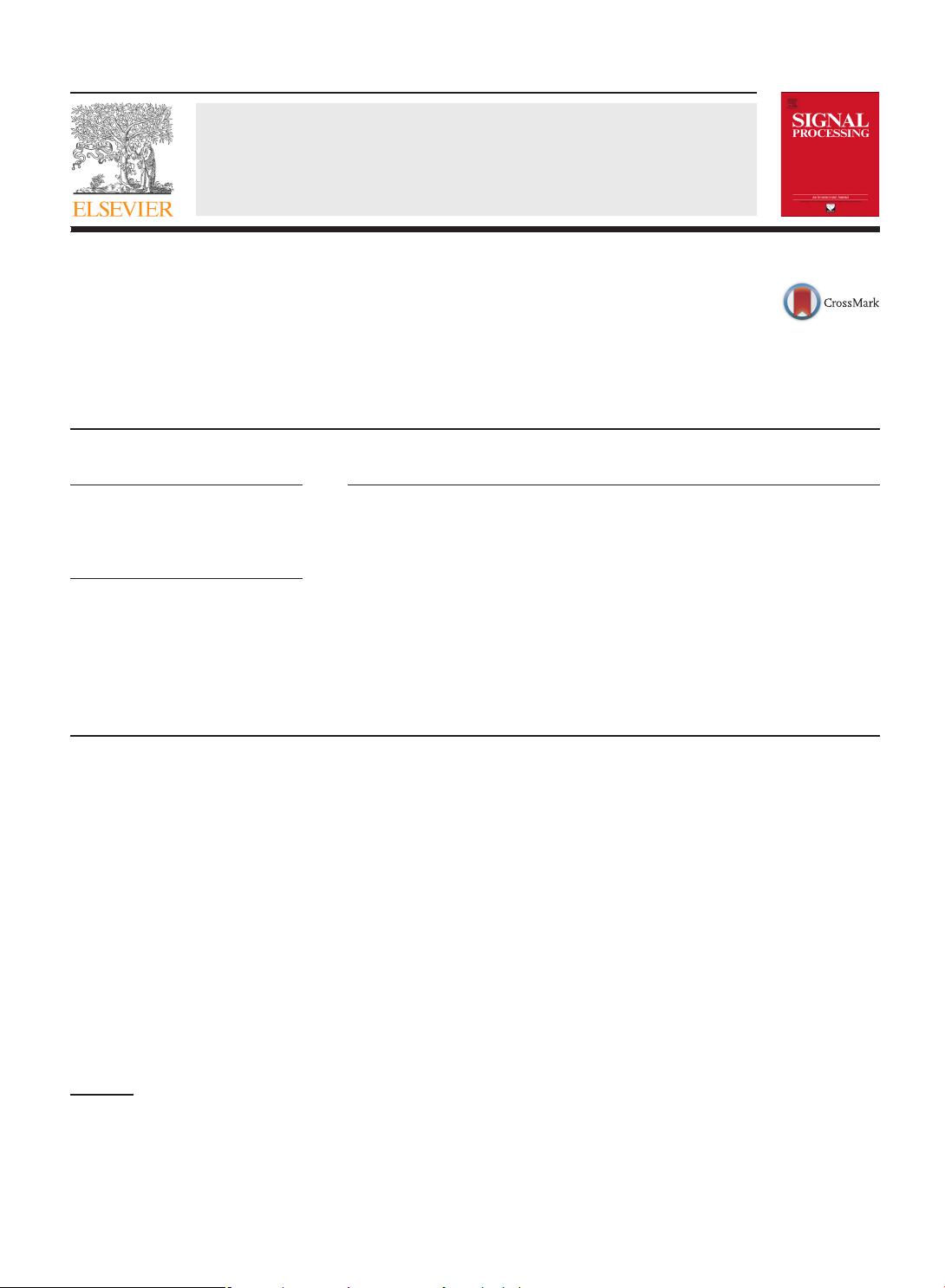
Fractional zero-phase filtering based on the
Riemann–Liouville integral
Jianhong Wang
a,b
, Yongqiang Ye
a,
n
, Xiang Pan
a
, Xudong Gao
a
, Chao Zhuang
a
a
College of Automation Engineering, Nanjing University of Aeronautics and Astronautics, Nanjing, China
b
School of Science, Nantong University, Nantong, China
article info
Article history:
Received 25 January 2013
Received in revised form
11 November 2013
Accepted 22 November 2013
Available online 1 December 2013
Keywords:
Fractional filtering
Zero-phase filtering
Forward–backward
Backward–forward
Riemann–Liouville integral
abstract
In this paper, two novel and computationally efficient, zero-phase filtering techniques are
proposed based on the Riemann–Liouville integral. Thanks to the reverse phase charac-
teristics of backward filtering, an overall zero-phase effect can be achieved by cascading a
fractional forward filtering with a fractional backward filtering, and vice versa. The
fractional zero-phase filtering can not only effectively suppress the phase distortion in the
filtering process but also better enhance the compromise capability between signal
denoising and signal information retention than the conventional filtering methods do.
The proposed methods are evaluated on Electrocardiogram (ECG) signal, by adding
disturbance, random, and white Gaussian noises to visually clean ECG record, and
studying SNR and MSE of the filter outputs. The results of the study demonstrate superior
performances compared with conventional signal denoising methods, such as Riemann–
Liouville integral filtering, Grünwald–Letnikov integral filtering, zero-phase Butterworth
filtering, and zero-phase average window filtering.
& 2013 Elsevier B.V. All rights reserved.
1. Introduction
Modern signal analysis and processing is essentially
researching non-linear, non-causal, non-minimum phase
system, non-Gaussian, non-stationary, non-integer dimen-
sion (or fractal) signal, and non-white additive noise [1].
Researches show that some fractional operation-based
methods such as fractional Fourier transform [2], fractional
wavelet packet transform [3], fractional wavelet spline
transform [4], fractional processes and fractional order
signal processing [5], and fractional Brownian motion [6],
are the powerful tools for analyzing and processing the
said problems. These researches indicate that fractional
calculus [7] can also be used for analyzing and processing
the above problems.
Signal denoising is an important part of signal proces-
sing. Scholars have put forward many denoising algo-
rithms including mean filtering, order statistics filtering,
low-pass filtering, Wiener filtering, etc. [1]. The above
various algorithms can remove noise in varying degrees.
However, these algorithms could lose signal detail infor-
mation in the filtering process and cause blur. The reason
for the phenomenon of signal blurring is that all the above
denoising algorithms make use of integer-order integral to
build their corresponding denoising model directly or
indirectly, which seriously reduce the high frequency
information in original signal [8]. Thus, it could result in
the lost of a lot of detail information in the process of
signal denoising.
The significant advantage of fractional order systems
over their counterparts is that they are characterized by
infinite memory, whereas integer order systems are char-
acterized by finite memory. As a result, modeling real-
world phenomena using fractional calculus has received
considerable attention in the last few decades [7]. Many
Contents lists available at ScienceDirect
journal homepage: www.elsevi er.com/locate/sigpro
Signal Processing
0165-1684/$ - see front matter & 2013 Elsevier B.V. All rights reserved.
http://dx.doi.org/10.1016/j.sigpro.2013.11.024
n
Corresponding author. Tel.: þ86 25 84892301.
E-mail addresses: wang.jhong@ntu.edu.cn (J. Wang),
melvinye@nuaa.edu.cn (Y. Ye), panzaochen@126.com (X. Pan),
happyday-gg@163.com (X. Gao), chaoauto@126.com (C. Zhuang).
Signal Processing 98 (2014) 150–157