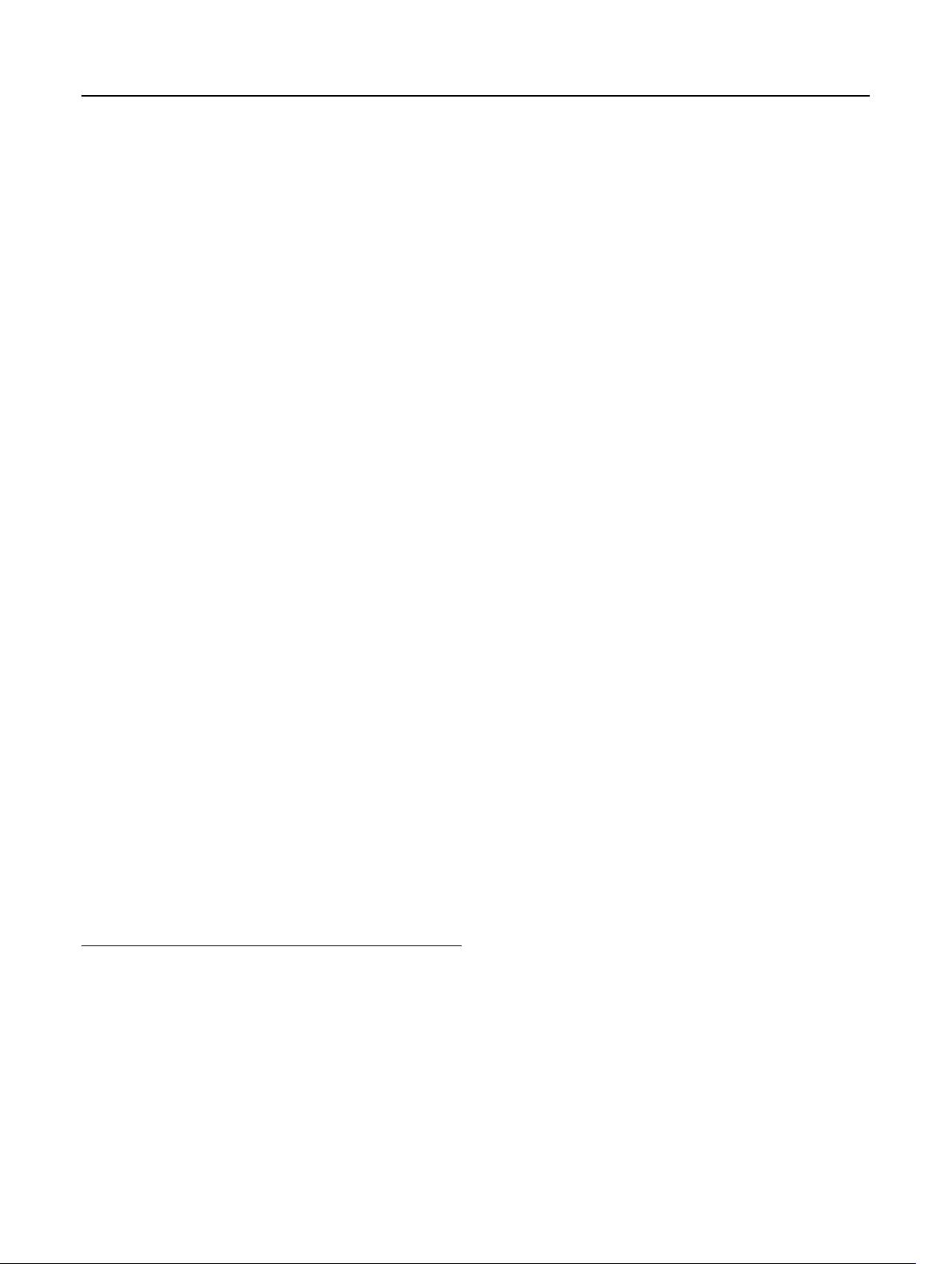
Automatic Removal of Artifacts from Attention Deficit
Hyperactivity Disorder Electroencephalograms Based
on Independent Component Analysis
Ling Zou
•
Soukun Xu
•
Zhenghua Ma
•
Jieru Lu
•
Wenjing Su
Received: 30 April 2012 / Accepted: 28 November 2012 / Published online: 5 January 2013
Springer Science+Business Media New York 2013
Abstract In this paper, we present an automatic artifact
removal method by combining independent component
analysis (ICA) and wavelet denoising to remove artifacts
from attention deficit hyperactivity disorder (ADHD)
electroencephalograms (EEGs). First, the ICA algorithm is
used to analyze EEG signals to get the independent com-
ponents (ICs); then, the wavelet method is applied to the
demixed ICs as an intermediate step; finally, the EEG data
are reconstructed from the new ICs (that are obtained in the
intermediate step) by using the inverse ICA. The experi-
mental results show that the automatic artifact removal
method can not only remove eye artifacts, muscle artifacts
and some unknown physiological artifacts sources, but also
efficiently keep the weak neural activities from strong
background artifacts. The results can establish a basis for
ADHD clinical diagnose study.
Keywords Attention deficit hyperactivity disorder
(ADHD) Electroencephalograms (EEGs) Automatic
artifacts removal method (AARM) Independent
component analysis (ICA) Wavelet denoising (WD)
Introduction
Attention deficit hyperactivity disorder (ADHD) is one of
the most common neurological diseases in children today,
affecting 3–5 % of all school-aged children [1]. The
symptoms include hyperactivity, impulsiveness and inat-
tention. ADHD interferes with many areas of normal
functioning and can severely affect normal development [2,
3]. For this reason, the disorder has been paid much
attention in recent years. A task that is often used to assess
different executive processes impaired in ADHD is the so-
called cued-Go/Nogo task or continuous performance test
(CPT) task, in which subjects have to respond to a Go
stimulus provided that it is preceded by a predefined cue
stimulus, and inhibit responses on Nogo trials. Event-
related potentials (ERPs) are widely used to study atten-
tion, memory and affective mechanisms of the nervous
system. By measuring ERPs additional to behavioral
measures in the CPT task, it is possible to further specify
which subprocesses are involved in deficient performance
in such tasks [4, 5].
The recorded electroencephalogram (EEG) signals rep-
resent a mixture of brain and non-brain activities, such as
cortical brain sources, physiological artifacts (including
eye movements, eye blinks and muscle activities), external
artifacts and spatially irregular components of unknown
origins. Impurities or contamination of any EEG activity
can lead to serious misreading, misinterpretation and
incorrect analysis, consequently, loss of data. Thus, a
critical point in EEG is to pay careful attention to reducing
any contamination.
The most common method to reduce artifact contami-
nation is linear regression in the time or frequency domain.
This method estimates and removes the influence of eye
blinks or eye movements on the signals recorded by scalp
L. Zou
State Key Laboratory of Robotics and System, Harbin Institute
of Technology, Harbin 150001, Heilongjiang, China
L. Zou S. Xu Z. Ma (&) J. Lu
School of Information Science and Engineering, Changzhou
University, Changzhou 213164, Jiangsu, China
e-mail: jsjma@126.com
W. Su
School of Information and Electronics, Beijing Institute
of Technology, Beijing 100081, China
123
Cogn Comput (2013) 5:225–233
DOI 10.1007/s12559-012-9199-3