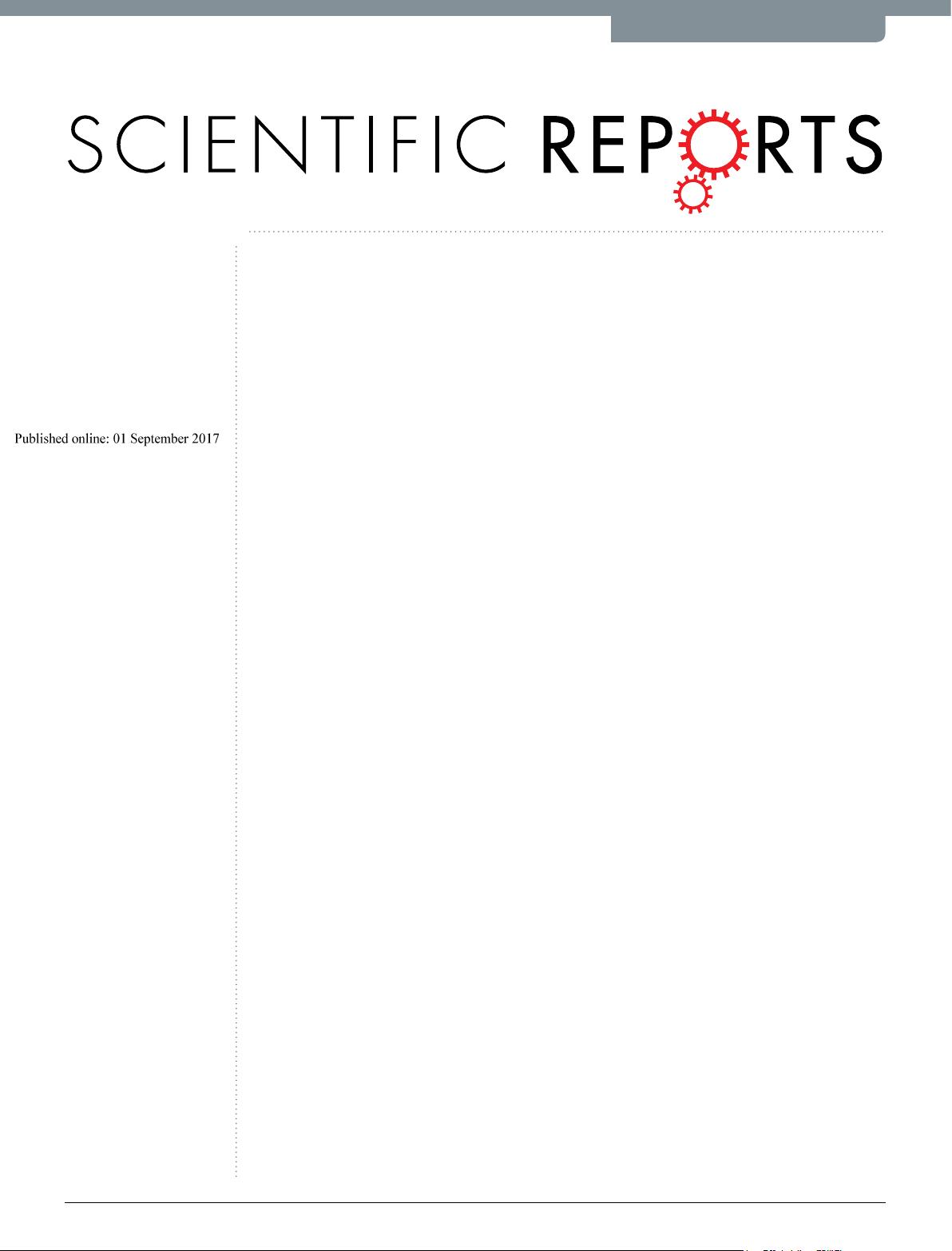
1
Scientific RepoRts | 7: 8533 | DOI:10.1038/s41598-017-08040-8
www.nature.com/scientificreports
Automatic Categorization and
Scoring of Solid, Part-Solid and
Non-Solid Pulmonary Nodules in CT
Images with Convolutional Neural
Network
Xiaoguang Tu
1
, Mei Xie
3
, Jingjing Gao
3
, Zheng Ma
1
, Daiqiang Chen
4
, Qingfeng Wang
5
, Samuel
G. Finlayson
6,7
, Yangming Ou
8
& Jie-Zhi Cheng
2
We present a computer-aided diagnosis system (CADx) for the automatic categorization of solid, part-
solid and non-solid nodules in pulmonary computerized tomography images using a Convolutional
Neural Network (CNN). Provided with only a two-dimensional region of interest (ROI) surrounding each
nodule, our CNN automatically reasons from image context to discover informative computational
features. As a result, no image segmentation processing is needed for further analysis of nodule
attenuation, allowing our system to avoid potential errors caused by inaccurate image processing.
We implemented two computerized texture analysis schemes, classication and regression, to
automatically categorize solid, part-solid and non-solid nodules in CT scans, with hierarchical features
in each case learned directly by the CNN model. To show the eectiveness of our CNN-based CADx,
an established method based on histogram analysis (HIST) was implemented for comparison. The
experimental results show signicant performance improvement by the CNN model over HIST in both
classication and regression tasks, yielding nodule classication and rating performance concordant
with those of practicing radiologists. Adoption of CNN-based CADx systems may reduce the inter-
observer variation among screening radiologists and provide a quantitative reference for further nodule
analysis.
Pulmonary nodules are small masses within the pulmonary interstitium that pose a dicult but extremely impor-
tant dierential diagnosis when detected by computerized tomography. In CT scans, pulmonary nodules appear
as relatively bright structures, and can appear in isolation within the lung parenchyma or as an attachment to the
lung chest wall, airway, pulmonary vessel, or ssure. Recent studies have suggested that the phenotypic features
of pulmonary nodules detected in CT images are predictive of malignancy
1
, and are thus extremely important for
determining further diagnosis, management, and treatment. In clinical practice, however, the dierential diagno-
sis of a pulmonary nodule in CT images is a complicated and challenging decision process
2, 3
. Despite continuous
modication and improvement in diagnostic guidelines over the years
3, 4
, physicians’ accuracy in diagnosing
pulmonary nodules remains varied
3, 5
and also highly dependent on experience
6
. ese clinical challenges moti-
vate the development of computer-aided diagnosis (CADx) systems that perform objective, quantitative, and
1
School of Communication and Information Engineering, University of Electronic Science and Technology of China,
Xiyuan Ave. 2006, West Hi-Tech Zone, Chengdu, Sichuan, 611731, China.
2
Department and Graduate Institute of
Electrical Engineering, Chang Gung University, 259 Wen-Hwa 1st Road, Kwei-Shan Tao-Yuan, 333, Taiwan.
3
School
of Electronic Engineering, University of Electronic Science and Technology of China, Xiyuan Ave. 2006, West Hi-Tech
Zone, Chengdu, Sichuan, 611731, China.
4
Third Military Medical University, Chongqing, 400038, China.
5
School of
Software Engineering, University of Science and Technology of China, 230026, Hefei, China.
6
Department of Systems
Biology, Harvard Medical School, 10 Shattuck St., Boston, MA, 02115, USA.
7
Harvard-MIT Division of Health Sciences
and Technology (HST), 77 Massachusetts Avenue, E25-518, Cambridge, MA, 02139, USA.
8
Department of Radiology,
Harvard Medical School, 1 Autumn St., Boston, MA, 02215, USA. Correspondence and requests for materials should
be addressed to M.X. (email: mxie@uestc.edu.cn) or J.-Z.C. (email: jzcheng@ntu.edu.tw)
Received: 30 August 2016
Accepted: 29 June 2017
Published: xx xx xxxx
OPEN