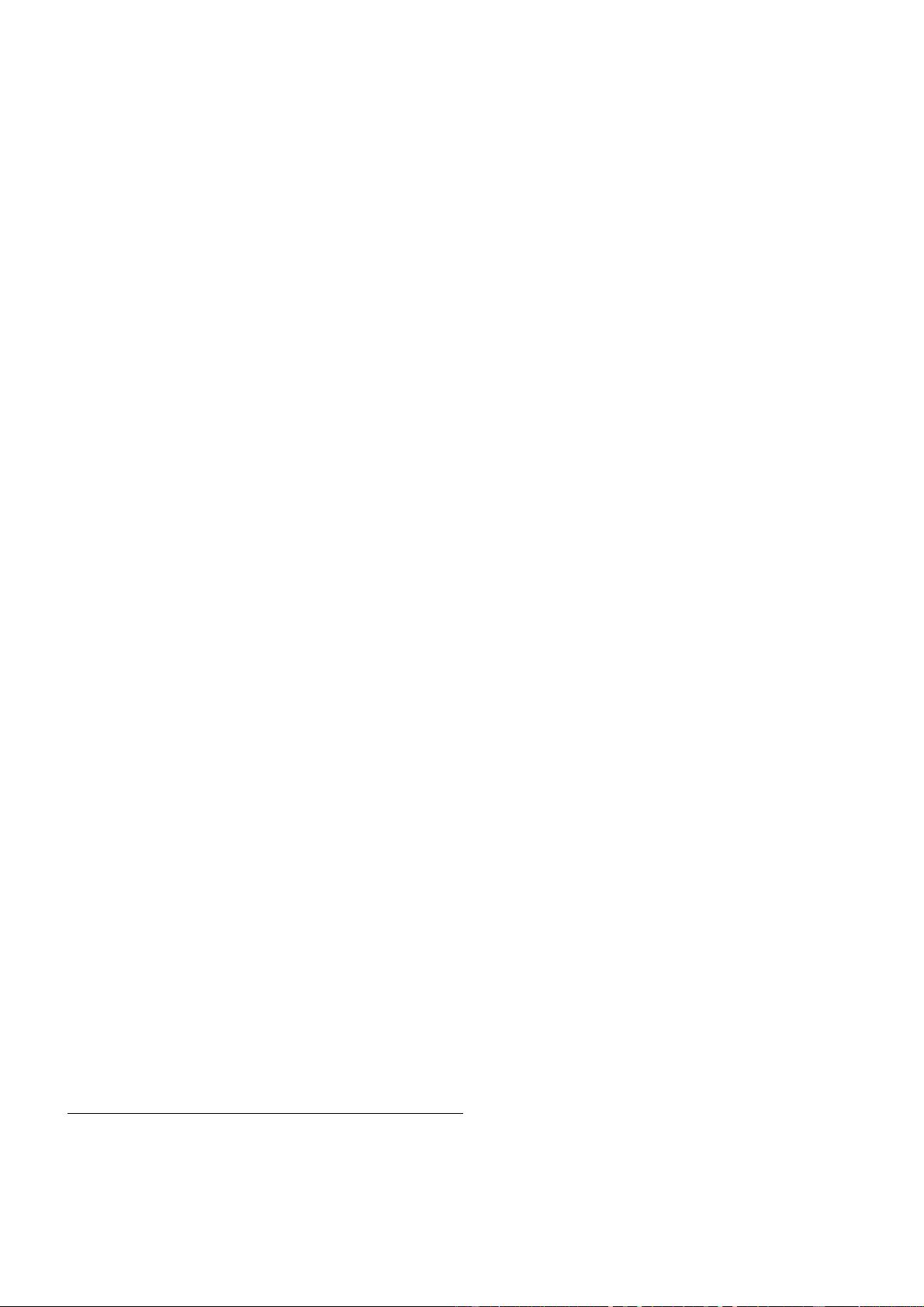
Method of Tikhonov Regularization for Weighted
Frequency-Difference Electrical Impedance
Tomography
Bing Han, Yanbin Xu, Feng Dong
Tianjin Key Laboratory of Process Measurement and Control
School of Electrical Engineering and Automation, Tianjin University
Tianjin, China
E-mail: xuyanbin@tju.edu.cn
Abstract—Frequency-difference electrical impedance
tomography (fdEIT) has attracted considerable attention, which
can visualize anomalies without requiring any time-reference
data obtained in the absence of anomalies and alleviate
undesirable effects of modeling errors. Simple frequency-
difference (SFD) method and weighted frequency-difference
(WFD) method are two existing algorithms. Besides, WFD
perform better due to its high resolution in the condition where
the conductivity of background changes much. However,
truncated singular value decomposition (TSVD) regularization
adopted in WFD have several problems such as difficulties to
choose the accurate truncation parameter, unsatisfactory image
quality even in low noise condition and overwhelming
computational tasks for large-scale problems. In this paper, an
improved WFD algorithm using Tikhonov regularization
(WFDTR) method as well as the selection method of
regularization parameter is proposed. This method can figure out
the appropriate regularization parameter more easily through L
curve method and acquire reconstructed distribution images of
good quality. What’s more, the second corner of L curve is
investigated. Finally, the proposed method has been compared
with the conventional WFD method through numerical
simulations. The proposed method works better than WFD in
relatively low noise cases according to localization error, shape
error as well as image noise indexes. In relatively high noise
condition, they perform approximately equally.
Keywords—electrical impedance tomography; weighted
frequency-difference; Tikhonov regularization; L curve
I. INTRODUCTION
Electrical impedance tomography (EIT) is a developing
measurement technique aiming to reconstruct complex
conductivity distribution of the measured object and detect the
anomalies. It recovers the internal electrical property
distribution usually by injecting current into the object and
acquiring the resulting voltage data on its boundary. EIT has
several advantages such as non-invasion, rapid response and
functional imaging, which means a promising possibility for
clinical diagnosis and long-term monitoring in hospital [1].
However, the spatial resolution is not so satisfying due to its
ill-posed nature.
Frequency-difference EIT (fdEIT) method was proposed to
reconstruct a distribution image of frequency-dependent
changes. According to the conductivity’s frequency-dependent
property of biological tissues, it employs two driving
frequencies with no need for demarcate knowledge [2-6].
Traditional fdEIT adopts simple frequency-difference (SFD)
method utilizing a simple measurement difference at two
frequencies to reconstruct distribution of conductivity
difference. However, it fails to work when it is assumed that
the conductivity of the background changes with frequency [9-
10].
Weighted frequency-difference (WFD) method was
introduced by Woo in 2008 [7-11] as an improved method for
conventional fdEIT. It employs electric currents at two
frequencies and measures the resulting weighted voltage
difference to reconstruct distribution of weighted conductivity
difference, from which anomalies can be detected. Compared
to SFD, WFD can significantly improve the quality of
reconstructed image in cases when the conductivity of the
background varies.
In WFD method, truncated singular value decomposition
(TSVD) is utilized to solve the inverse problem. Generalized
cross-validation (GCV) and Picard plot methods are normal
approaches to determine the truncation parameter [12].
However, those methods do not perform well in certain
situations of fdEIT [7]. What’s more, the truncation parameter
of TSVD is an integer, which means its minimum resolution is
“1” and the calculation precision cannot reach the decimal
scale. Besides, TSVD explicitly requires the whole
computation of SVD. This computational task is too
overwhelming for large-scale problems [13], which is not
suited for future development of fdEIT with more pixels and
more measured data.
To address the issues of WFD, in this paper, an improved
WFD algorithm based on Tikhonov regularization (WFDTR)
was proposed. L curve method has been utilized to choose an
appropriate regularization parameter, whose calculation
precision can reach the decimal scale. Besides, a new corner of
the L curve has been explored. Properties of both the original
global corner and the new corner were investigated. A more
The authors appreciate the support from the National Natural Science
Foundation of China (No. 61671322 & No. 61571321) and the Natural
Science Foundation of Tianjin (No. 16JCYBJC18600).