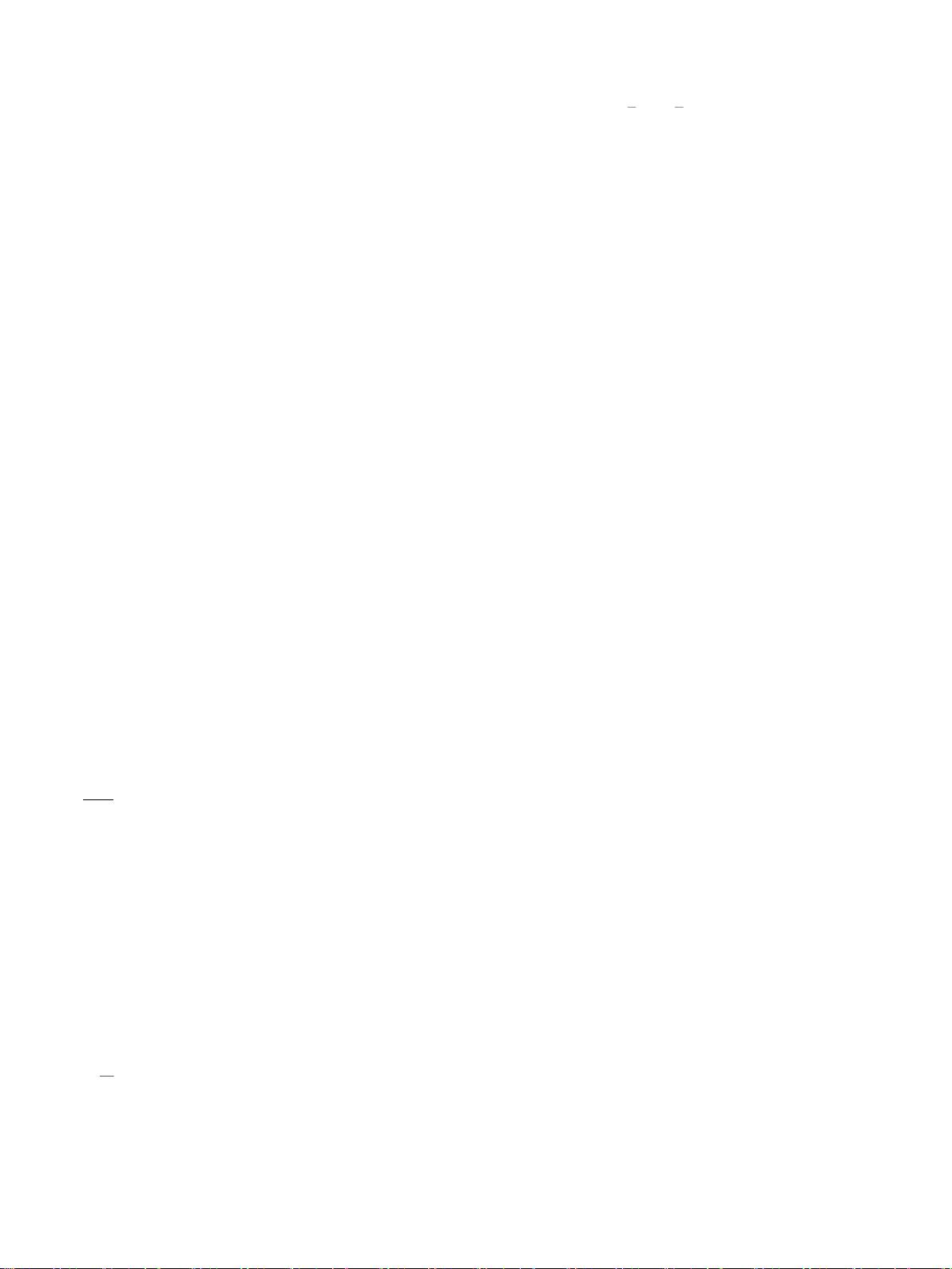
在海洋机械论中,责任与被忽视的数据价值有关,但不是责任人。
x
0
,
所以
Andx
ð
1i
denote the observed and missing elements ofx
i
,我尊
重和错过的元素。《机械论对平等的
其中
p
1
andp
0
¼ 1-p
1
分别
是
Y¼ 1 andY¼ 0
的采样比例
;
X
1
andX
0
分别是
X
的
given Y¼ 1 and Y¼ 0
,
and r
X
这是
X
标准
的退化。
This
的
测量范围从-1到+1。
选择的优势完全基于Pearson的相关性。Therefore,the
correlation values obtained for relationships相关价值观
不同的变量类型可以比较。
无论是
什么
,都有不同的
价值观
。
x
∈
1
i
;
x
ω
首页>外文书>艺术>音乐>
Σ
in
the
samplespace
of
X
1
您
的符合EQ标准。第二章错误在一个变量X
j
上
的概率依赖于其他值估计
值(或变量),但不是X
j
本身的值
最后,
NAR
机械的使命
2019
年
12
月
19
日,出版社出版了《
The
distri-bution ofm
i
depends on the Missing elements ofx
i
,
i.e.
Eq
》。
2Does
不求回报,
只求
回报
,
求
回报
。这是唯一的机制,它允许在一个变量X
j
和
X
j
本身的价值上的 概率
-责任之间的关联。此外,责任可以取决于被忽略的数据价
值作为长期的它甚至报告到责任人。
3.2.
Correlation
相关
Correlation
是衡量两个变量之间关联的标准一个关于如何改变一
个 变 量大小 的 指标是与另一个变量大小的变化相关的(
Schober
等
人)。
2018
)。但我们必须清楚一个特定变量的价值观是一个更好
的预测一个相关变量的价值,相关性不需要确定因果关系。
相关性是衡量变量之间相关性退化的统计方法存在严重的共同
性,适用于变量的特定类型和具有显着的低估假设。
Below
,这篇论
文中使用的有效性将被呈现。
PearsonCom- monly denoted byr
,
this coefficient measures
the strength of a lin- ear relationship between two numeric
,
random variablesX and Y
,
calculated by the following
equation
:(这是一个林
-ear
关系的强度之间的两个数字,随机变量
X
和
Y
,通过后续方程来计算
4.
基于相关性的归因技术
Novel Correlation-based Injection
Techniques
本 文 目 的 是 三 个 新 技 术 which level color- relation when
performing missing value implination。 这 些 技 术- Niques Aim to
tackle some of the drawback found in the methods from the
literature , such as the need for a complete sub- set and the
evaluation on a single mismisingness Mechanism.(从文献中吸取教
训 , 作 为 一 个 完 整 的 子 集 和 一 个 单 一 的 错 误 机 制 的 评 价 ) Fur-
thermore,他们利用相关性的概念,但建议不同意采用药物研究方法。
Rather than just resorting to the correlation between values,these
meth-ods investigate the potential benefits of considering the
correlation between values and missingness patterns.第一,我们只
是重新研究价值观和错误模式之间的相关性。如果此关联是强的,则不
完全变量的缺失元素将被限制在其他变量值的分布中的特定部分。对于
那些被忽略的元素,Such association可能会有所帮助。
对于这个目的,它是关键,以建立程序,由哪一个矩阵表示值和
缺失之间的相关性
-
路径被接受。
3.
了解任何数据集,让
g
拒绝所有功
能的 总 数 ,
6g
是
具有 错 误 值的功能 的 数 量。
g
j
represents thej th
feature
,
where
,等等。
j
2
f1
;
2
?
;g
,
and g
miss
;
i
represents the i th incomplete feature
,
where i
2
f1; 2
; . G
小 姐
G
小 姐
Furthermore
认 为
C
vm
是
a
{
g
miss
*
g
matrix
。
For
every
pair
f
i
;
j
g
,
with
g
mis
;
i
- g j ; C v m. i ; j ] stores the
correlation between the values of g j and the mismisingness pattern of g miss ; i
,
i
,
i. e.
,
a. n.
在每一个
g
的值和
g
小姐的错误路径之间的相关性
; i
,
i
,
i
,
e
,
i
,
e
,它的二进制缺失指标。
Additionally, letC
vv
加 入
别 名: Denote the Standard g g
Cor r ela t i on 相关
Matrix 的 。 This matrix is computed through a pairwise deletion
strat- egy这个矩阵是通过一个悬停性删除strat- egy计算出来的,即,
相关性是在分析基础上的可评估价值之间计算的,而分析基础上的数据
主体的可用价值是相互关联的。
4.1.
Correlation weighted K-nearest neighbours industration
最近的邻居
其中,
XY
是
X
和
Y
之间的协方差值,而
X
和
R
Y
分别是
X
和
Y
变量
的标准变
化值。
Pearson's Coefficient is a normalised covariance,scaled so that
it ranges from 1 to +1, indicating a perfect negative and positive
lin- ear correlation,分别地,从1到1的范围缩小。Pearson的相关性
仅 适 用于随 机数字 变 量, 遵 循一 个 bivariate normal distri- bution
(Schober等,。Akoglu,2018)。
The Phi Coefficient
,
denoted by
/
,
is the equivalent of
Pearson
[1] cefficient
,计算两个二进制变量
X
和
Y
之间的
线性相关。它可以通
过
Pearson
的
chi-square goodness-of-fit
统计数据来计算
r
2
根 据 《 The Correlation Weighted K-Nearest Neighbours
Imputation(CWKNNI)》,该杂志提出了由Liu et al.(2019)提出
的由CohiKNN发起的归因技术方法。However,instead of uniquely
considering the correlation between values of different attributes,
the weights in CWKNNI are obtained through a weighted average
of the correlation between values and the extra- lation between
values and missivingness patterns. However , instead of unially
considering the correlation between values of different attributes,
CWKNNI're obtained through a weighted average of the correlation
between values and the crising patterns. However,不同属性之间的
价 值 和 错 误 路 径 之 间 的 相 互 关 系 的 相 互 作 用 。 添 加 , CWKNNI
overcomes the limitation of CohiKNN in terms of its dependency on
a complete data subset to impute the missing val- ues , as it
computes the correlation matrix through a pairwise of him- tion
approach instead of performing listwise deletion.在一个完整的数据
子项下超越了CohiKNN的限制,因为它计算了一个匹配矩阵,通过它
的一个徘徊-完成列表删除的方法。
一个简单的流媒体的CWKNNI是拍在图1a和4- lowing step-by-step
explanation provides the outline for this method:
其中v
2
是Chi-square的统计数据,而N是观测值的个数。Phi Coefficient
的排名从-1到+1。
点
biseric
相关系数,由
r
pbi
表示,测量在一个
binary
标称变量
Y
和