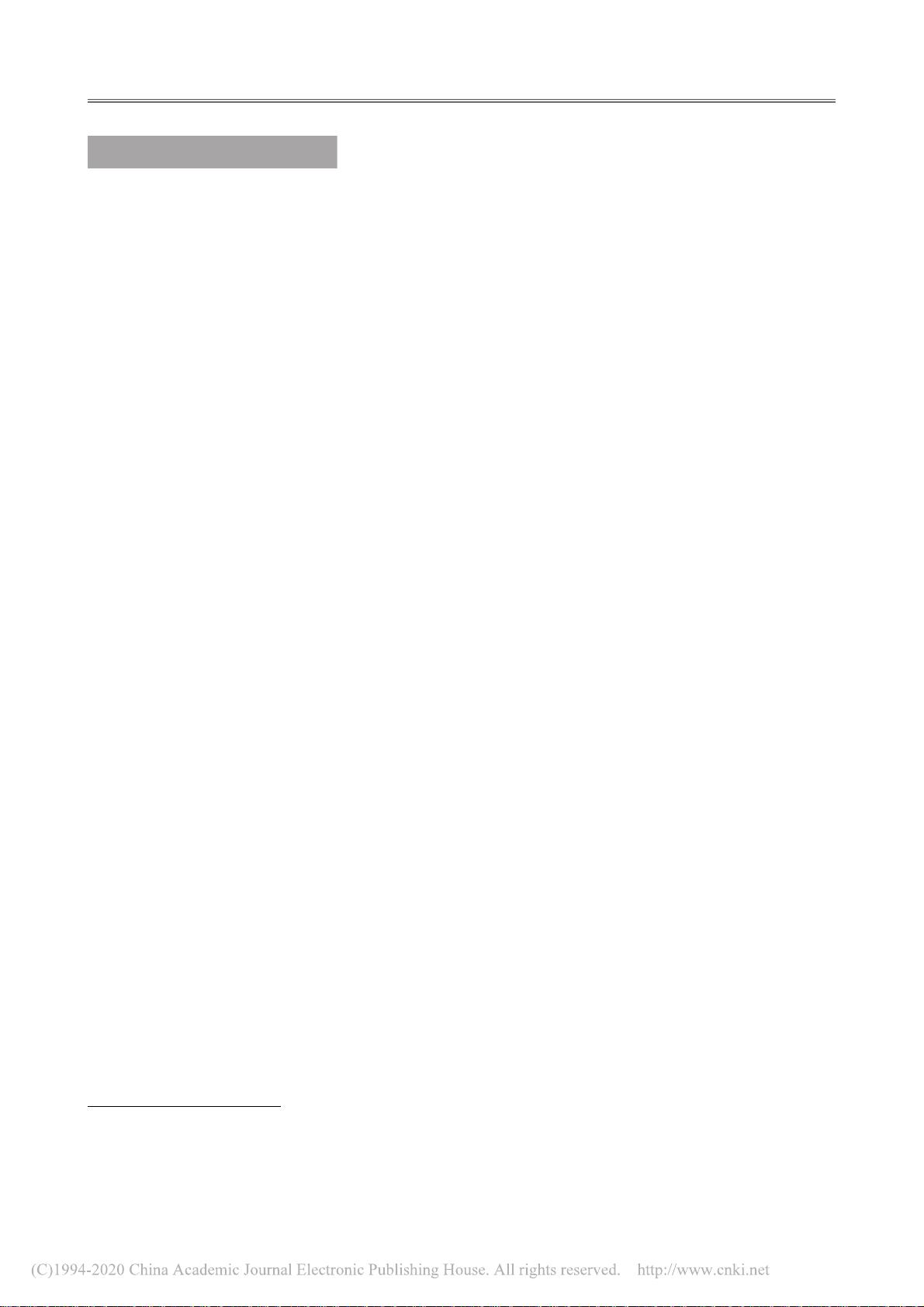
第
29
卷第
4
期
2020
年
8
月
实用心电学杂志
Journal of Practical Electrocardiology
Vol. 29 No. 4
Aug. 2020
基金项目
:
十堰市太和医院院级项目
( 2019JJXM039)
作者单位
: 442000
湖北 十堰
,
十堰市太和医院全科医学科
(
王官军
,
唐祖胜
) ,
循证医学中心
(
汪龙
) ;
湖北医药学院第一临床学院
(
吴婷
)
作者简介
:
王官军
,
主治医师
,
主要从事大数据在医学领域的应用研究
。
通信作者
:
唐祖胜
,E-mail: tzs116@ 126. com
心电大数据与人工智能 专栏主持
:
卢喜烈
【
开栏寄语
】
心电大数据是医学大数据不可分割的重要组成部分
。
大数据具有海量存储
、
数据类型多样
、
处理速度快
、
价值密度低等特点
。
深挖大数据中的有用信息
,
可为临床医学和人类健康服务
。
心电的根本
出路在于实现心电信息化
、
自动化和智能化
。
四十多年以来
,
国外在心电自动化诊断方面已经做出了可喜
的成就
;
近十年来
,
我国在心电智能方面的研究也取得了长足进展
,
但心电人工智能化水平还处于初级阶
段
。
本刊本期新推出
《
心电大数据与人工智能
》
专栏
,
为相关领域研究成果提供发表平台
,
欢迎投稿
!
基于机器学习的心电图诊断研究
王官军 吴婷 汪龙 唐祖胜
[
摘要
]
心电图在心血管疾病诊断中具有不可替代的作用
,
而机器学习在心电诊断自动化方
面显现出独特优势
。
本文利用
PTB-XL
公共心电数据库的
21 837
条心电图记录
,
对心电数据
进行缺失值删除
、
裁剪
、
去基线等预处理
,
利用主成分分析降维算法提取特征
,
再用
K-
近邻算
法
、
随机森林
、Logistic
回归
、
支持向量机算法进行分类研究
,
并根据研究结果对
4
种算法的性
能进行比较分析
。
结果表明
:
综合预测准确率
、
召回率
、
精准率
,
以及
ROC
曲线模型评价指标
来看
,
支持向量机在模型预测中的表现优于其他
3
种算法
。
[
关键词
]
机器学习
;
人工智能
;
智能诊断
;
支持向量机
; K-
近邻算法
;
随机森林
; Logistic
回归
[
中图分类号
] R540. 4;TP181 [
文献标志码
] A [
文章编号
] 2095 -9354( 2020) 04 -0262 -07
DOI: 10. 13308 / j. issn. 2095 - 9354. 2020. 04. 007
[
引用格式
]
王官军
,
吴婷
,
汪龙
,
等
.
基于机器学习的心电图诊断研究
[J].
实用心电学杂
志
,2020,29( 4) : 262 - 268,297.
Research of ECG diagnosis based on machine learning Wang Guan-jun
1
,Wu Ting
2
,Wang Long
3
,Tang Zu-
sheng
1
( 1. Department of General Medicine,Taihe Hospital of Shiyan City ,Shiyan Hubei 442000; 2. the First
Clinical College,Hubei University of Medicine,Shiyan Hubei 442000; 3. Evidence-based Medicine Center,Taihe
Hospital of Shiyan City,Shiyan Hubei 442000,China)
[Abstract] Electrocardiogram( ECG) plays an irreplaceable role in the diagnosis of cardiovascular diseases,and
machine learning shows a unique advantage in the automation of ECG diagnosis. A total of 21 837 ECG records in the
public ECG database PTB-XL were used. The ECG data were preprocessed by deleting missing values,clipping and
removing baseline before extracting their relevant features by principal component analysis. Classification research
was carried out using K-nearest neighbor,random forest,Logistic regression and support vector machine( SVM) algo-
rithms,and the performances of the four algorithms were compared and analyzed according to the research results.
The results show that the SVM algorithm is obviously superior to the other three classification algorithms in aspects of
model evaluation indicators including accuracy rate,recall rate,precision rate,and area under curve( AUC) .
[Key words] machine learning; artificial intelligence; intelligent diagnosis; support vector machine; KNN algo-