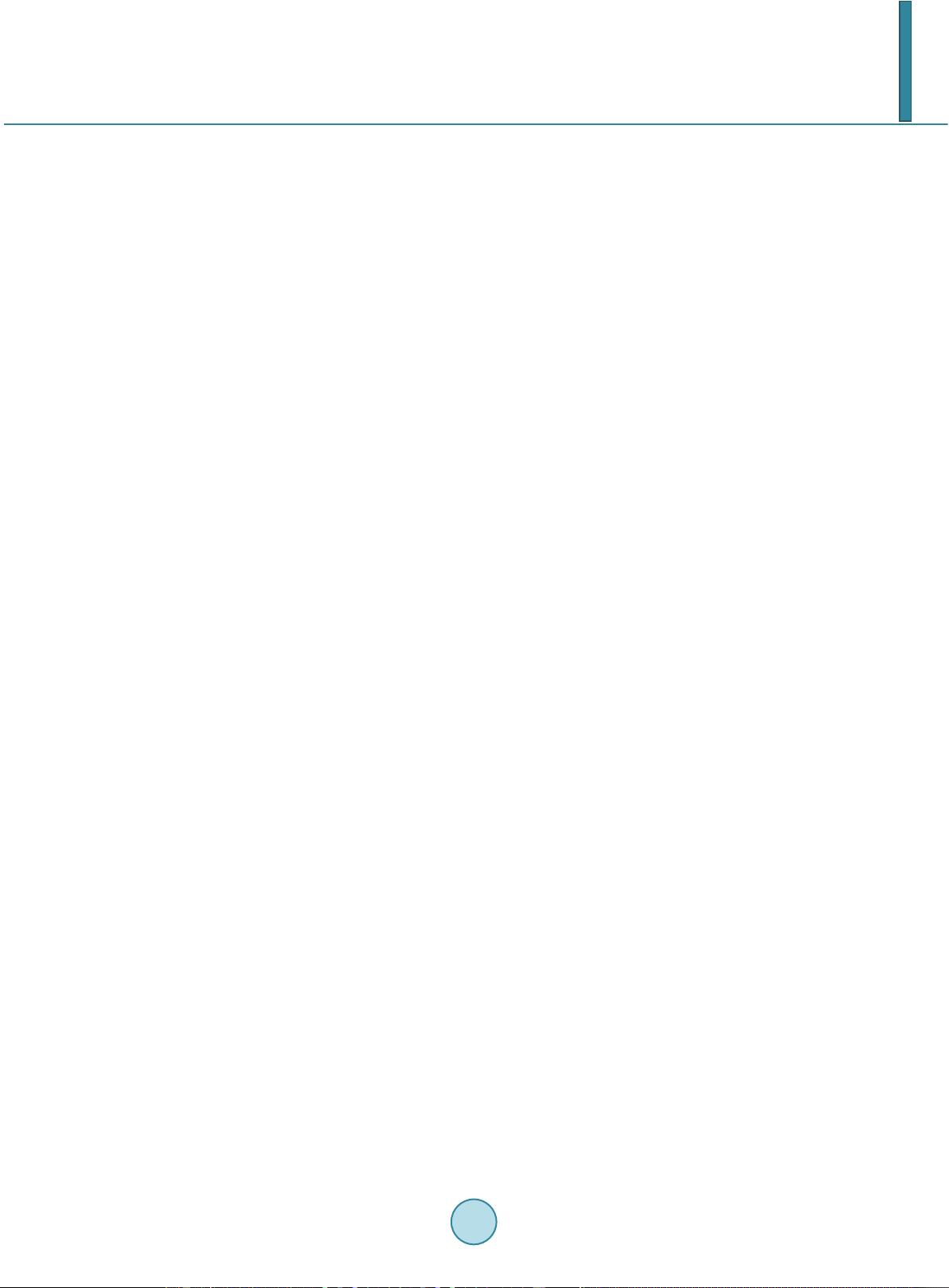
Wen-Ran Zhang & Karl E. Peace
soning in Intelligent Systems [30] is among the single most influential works in shaping the theory and practice
of knowledge-based systems in artificial intelligence, Rubin’s works have been leading in total number of cita-
tions in statistical analysis. While Pearl’s book Causality: Models, Reasoning, and Inference [31] went beyond
computer science and engineering and has made a major impact on the definability of causality, Rubin’s causal
model pioneered statistical assessment of cause and effect based on the conceptual framework of all potential
outcomes and is widely used in the medical and social sciences.
Here we are focused on comparing logical and statistical approaches to causal modeling but not on the two
competing statistical causal models. We randomly choose Pearl’s book as an example for our comparison with
logical approaches. Contrary to Zadeh’s assertion that “Causality is undefinable”, Judea Pearl’s book Causality:
Models, Reasoning, and Inference offers a complete axiomatic characterization – a structural causal model [31,
Ch7]. As Pearl described [32]: “Full Pearl calls for framing problems the way an investigator perceives nature
to work, regardless of whether one can estimate the parameters involved, then, once you set your model, you
mark down what you know and what you do not know, what you are sure about and what you are not sure
about, and do the analysis in this space of ‘Nature models’. If it so happens that you are only sure about the
structure of the graph and nothing else, then and only then, you do ‘graphs, colliders, and do operators.’”
It is evident from the above description that Pearl’s structural causal model is fundamentally a probabilistic
approach to reasoning and inference. In order to effectively use the model, a user needs to mark down what
he/she knows and what he/she does not know, what he/she is sure about and what he/she is not sure about. This
treatment clearly follows the empirical and analytical spirit in line with David Hume and Bertrand Russell,
where cause-effect relations are formed and improved through knowledge, experience and analysis but not on
the basis of new logical development and philosophical system building. Furthermore, since any probabilistic
certainty factor p can be deemed partial truth, the probabilistic approach is essentially truth-based. Therefore,
probabilistic or statistical definability of causality, albeit very useful in causal inference, doesn’t go beyond
truth-based reasoning and doesn’t attempt to answer the question: Is there a philosophically different funda-
mental causal logic system at the quantum level to be discovered?
2.2.6. Niels Bohr on Causality
Niels Bohr - a father figure of quantum mechanics - was the first to bring YinYang into quantum theory for his
particle-wave complementarity principle. When Bohr was awarded the Order of the Elephant by the Danish
government, he designed his own coat of arms which featured in the center a YinYang logo (or Taiji symbol)
and the Latin motto “contraria sunt complementa” or “opposites are complementary” (Fig. 1).
While Bohr’s quantum mechanics recognized particle-wave complementarity, it stopped short of identifying
the essence of YinYang bipolar coexistence. It can be argued that without bipolarity any complementarity is less
fundamental due to the missing direct “opposites” (Fig. 2) [9, 33]. If bipolar equilibrium is the most fundamental
form of equilibrium, any multidimensional model of spacetime such as string theory and superstring theory
cannot be most fundamental.
In brief, action-reaction, particle-antiparticle, negative-positive energies, input and output, or the Yin and
Yang of nature in general could be the most fundamental unitary opposites of all beings. Man and woman, space
and time, particle and wave, truth and falsity are dualities or dichotomies but not exactly unitary bipolar oppo-
sites. This could be the reason why Bohr found causal description of a quantum process unattainable and we
have to content ourselves with particle-wave complementary descriptions [5]. It could also be the fundamental
reason why quantum entanglement is still widely deemed a logical mystery until today and quantum computing
so far cannot lend itself as an analytical paradigm for quantum intelligence even though it has shown great po-
tential for solving some problems with high computation complexity. (Note: Newtonian action and reaction are
equal opposites that are extended to include non-equal opposites to account for modern discoveries such as CP
(Charge-Parity) violation.)