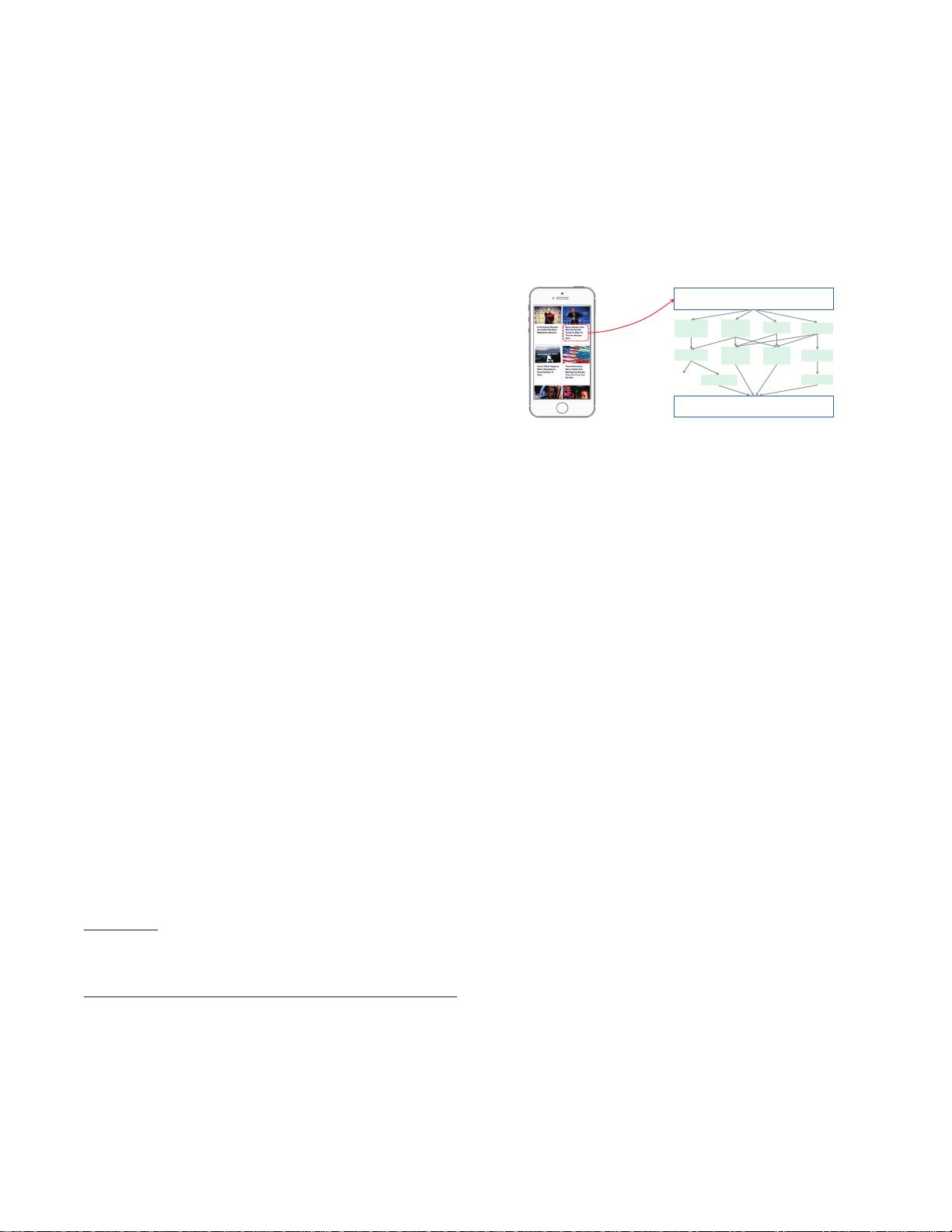
DKN: Deep Knowledge-Aware Network
for News Recommendation
Hongwei Wang
1,2
, Fuzheng Zhang
2
, Xing Xie
2
, Minyi Guo
1∗
1
Shanghai Jiao Tong University, Shanghai, China
2
Microsoft Research Asia, Beijing, China
wanghongwei55@gmail.com,{fuzzhang,xingx}@microsoft.com,guo-my@cs.sjtu.edu.cn
ABSTRACT
Online news recommender systems aim to address the informa-
tion explosion of news and make personalized recommendation for
users. In general, news language is highly condensed, full of knowl-
edge entities and common sense. However, existing methods are
unaware of such external knowledge and cannot fully discover la-
tent knowledge-level connections among news. The recommended
results for a user are consequently limited to simple patterns and
cannot be extended reasonably. To solve the above problem, in
this paper, we propose a deep knowledge-aware network (DKN) that
incorporates knowledge graph representation into news recom-
mendation. DKN is a content-based deep recommendation frame-
work for click-through rate prediction. The key component of DKN
is a multi-channel and word-entity-aligned knowledge-aware con-
volutional neural network (KCNN) that fuses semantic-level and
knowledge-level representations of news. KCNN treats words and
entities as multiple channels, and explicitly keeps their alignment re-
lationship during convolution. In addition, to address users’ diverse
interests, we also design an attention module in DKN to dynami-
cally aggregate a user’s history with respect to current candidate
news. Through extensive experiments on a real online news plat-
form, we demonstrate that DKN achieves substantial gains over
state-of-the-art deep recommendation models. We also validate the
ecacy of the usage of knowledge in DKN.
ACM Reference Format:
Hongwei Wang, Fuzheng Zhang, Xing Xie, and Minyi Guo. 2018. DKN: Deep
Knowledge-Aware Network for News Recommendation. In Proceedings of
The 2018 Web Conference (WWW 2018). ACM, New York, NY, USA, 10 pages.
https://doi.org/10.1145/3178876.3186175
1 INTRODUCTION
With the advance of the World Wide Web, people’s news reading
habits have gradually shifted from traditional media such as news-
papers and TV to the Internet. Online news websites, such as Google
News
1
and Bing News
2
, collect news from various sources and pro-
vide an aggregate view of news for readers. A notorious problem
∗
M. Guo is the corresponding author. This work was partially sponsored by the National
Basic Research 973 Program of China under Grant 2015CB352403.
1
https://news.google.com/
2
https://www.bing.com/news
This paper is published under the Creative Commons Attribution 4.0 International
(CC BY 4.0) license. Authors reserve their rights to disseminate the work on their
personal and corporate Web sites with the appropriate attribution.
WWW 2018, April 23–27, 2018, Lyon, France
©
2018 IW3C2 (International World Wide Web Conference Committee), published
under Creative Commons CC BY 4.0 License.
ACM ISBN 978-1-4503-5639-8/18/04.
https://doi.org/10.1145/3178876.3186175
Boris Johnson Has Warned Donald Trump
To St ick To The Iran Nuclear Deal
Boris
Johnson
Donald
Trump
Iran
Nuclear
Politician
Weapon
Congress
United
States
North
Korea
North Korean EMP
Attack Would Cause Mass
U.S. Starvation, Says Congressional Report
EMP
……
News the user
may also like
News the user
have read
Figure 1: Illustration of two pieces of news connected
through knowledge entities.
with online news platforms is that the volume of articles can be
overwhelming to users. To alleviate the impact of information over-
loading, it is critical to help users target their reading interests and
make personalized recommendations [2, 25, 27, 32, 34, 39].
Generally, news recommendation is quite dicult as it poses
three major challenges. First, unlike other items such as movies [
9
]
and restaurants [
12
], news articles are highly time-sensitive and
their relevance expires quickly within a short period (see Section
5.1). Out-of-date news are substituted by newer ones frequently,
which makes traditional ID-based methods such as collaborative
ltering (CF) [
41
] less eective. Second, people are topic-sensitive
in news reading as they are usually interested in multiple specic
news categories (see Section 5.5). How to dynamically measure
a user’s interest based on his diversied reading history for cur-
rent candidate news is key to news recommender systems. Third,
news language is usually highly condensed and comprised of a
large amount of knowledge entities and common sense. For ex-
ample, as shown in Figure 1, a user clicks a piece of news with
title “Boris Johnson Has Warned Donald Trump To Stick To The
Iran Nuclear Deal" that contains four knowledge entities: “Boris
Johnson”, “Donald Trump”, “Iran” and “Nuclear”. In fact, the user
may also be interested in another piece of news with title “North
Korean EMP Aack Would Cause Mass U.S. Starvation, Says Con-
gressional Report” with high probability, which shares a great deal
of contextual knowledge and is strongly connected with the previ-
ous one in terms of commonsense reasoning. However, traditional
semantic models [
30
] or topic models [
3
] can only nd their relat-
edness based on co-occurrence or clustering structure of words, but
are hardly able to discover their latent knowledge-level connection.
As a result, a user’s reading pattern will be narrowed down to a
limited circle and cannot be reasonably extended based on existing
recommendation methods.
To extract deep logical connections among news, it is necessary
to introduce additional knowledge graph information into news
recommendations. A knowledge graph is a type of directed het-
erogeneous graph in which nodes correspond to entities and edges
WWW 2018, April 23-27, 2018, Lyon, France