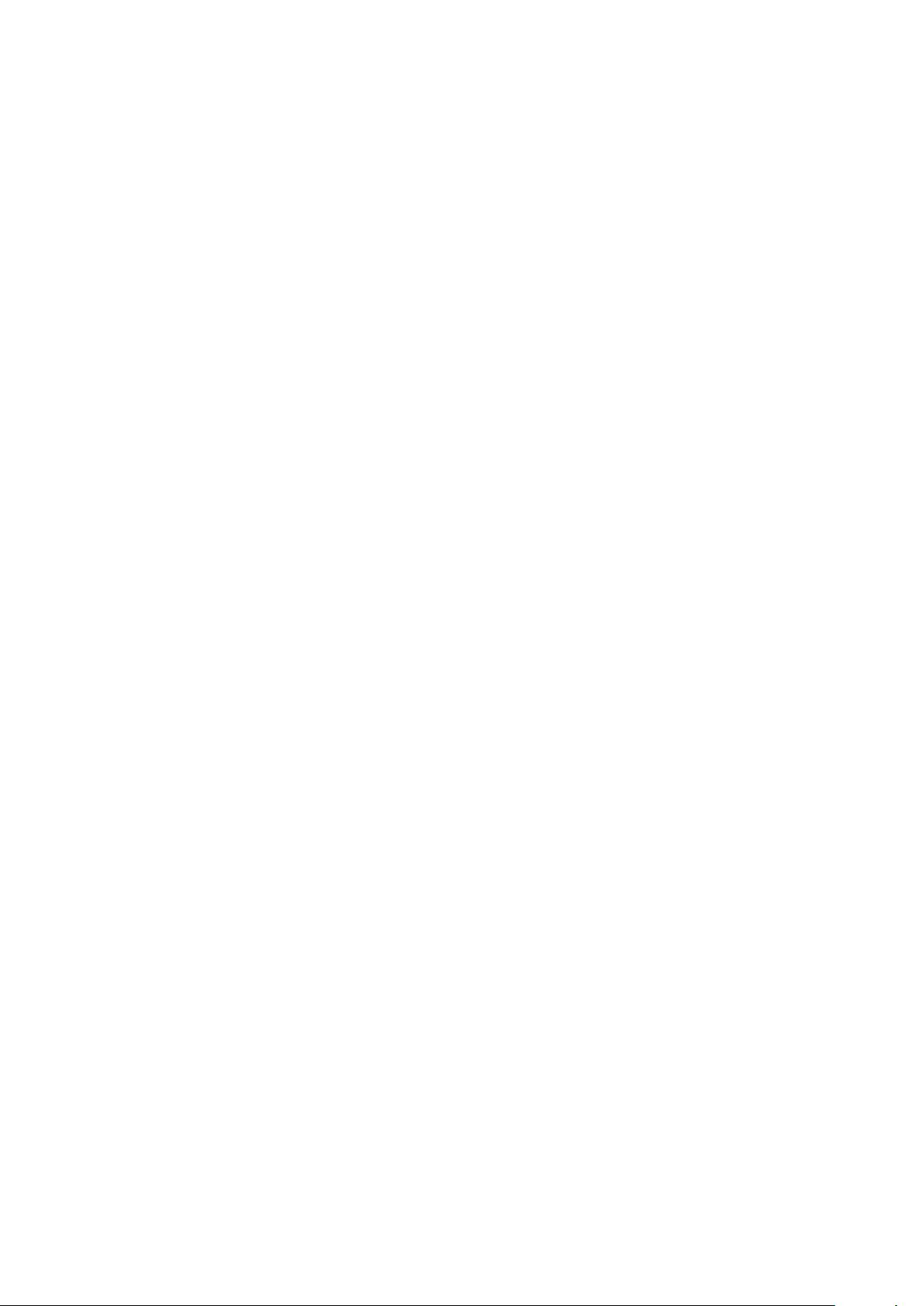
Convolutional Neural Networks for Multivariate Time Series Classification using
both Inter- & Intra- Channel Parallel Convolutions
G. Devineau
1
W. Xi
2
F. Moutarde
1
J. Yang
2
1
MINES ParisTech, PSL Research University, Center for Robotics, Paris, France
2
Shanghai Jiao Tong University, School of Electronic Information and Electrical Engineering, China
{guillaume.devineau, wang.xi, fabien.moutarde}@mines-paristech.fr
Abstract
In this paper, we study a convolutional neural network we
recently introduced in [9], intended to recognize 3D hand
gestures via multivariate time series classification.
The Convolutional Neural Network (CNN) we propo-
sed processes sequences of hand-skeletal joints’ positions
using parallel convolutions. We justify the model’s ar-
chitecture and investigate its performance on hand ges-
ture sequence classification tasks. Our model only uses
hand-skeletal data and no depth image. Experimental re-
sults show that our approach achieves a state-of-the-art
performance on a challenging dataset (DHG dataset from
the SHREC 2017 3D Shape Retrieval Contest).Our model
achieves a 91.28% classification accuracy for the 14 ges-
ture classes case and an 84.35% classification accuracy for
the 28 gesture classes case.
1 Introduction
Gesture is a natural way for a user to interact with one’s
environment. One preferred way to infer the intent of a
gesture is to use a taxonomy of gestures and to classify
the unknown gesture into one of the existing categories ba-
sed on the gesture data, e.g. using a neural network to per-
form the classification. In this paper we present and study a
convolutional neural network architecture relying on intra-
and inter- parallel processing of sequences of hand-skeletal
joints’ positions to classify complete hand gestures. Where
most existing deep learning approaches to gesture recog-
nition use RGB-D image sequences to classify gestures
[41], our neural network only uses hand (3D) skeletal data
sequences which are quicker to process than image se-
quences. The rest of this paper is structured as follows. We
first review common recognition methods in Section II. We
then present the DHG dataset we used to evaluate our net-
work in Section III. We detail our approach in Section IV in
terms of motivations, architecture and results. Finally, we
conclude in Section VI and discuss how our model can be
improved and integrated into a realtime interactive system.
Note that the contents of this paper are highly similar to
that of [9], especially sections 1, 2 and 3, as well as the fi-
gure illustrating the network, however in this article we fo-
cus more on practical tips and on justifying the network ar-
chitecture whereas the original paper focus was more cen-
tered on gesture-related aspects. Readers familiar with [9]
can directly skip to the subsection Architecture Tuning of
section IV, in which the network architecture is justified
more thoroughly.
2 Definition & Related Work
We define a 3D skeletal data sequence s as a vector s =
(p
1
· · · p
n
)
T
whose components p
i
are multivariate time se-
quences. Each component p
i
= (p
i
(t))
t∈N
represents a mul-
tivariate sequence with three (univariate sequences) com-
ponents p
i
= (x
(i)
,y
(i)
,z
(i)
) that alltogether represent a time
sequence of the positions p
i
(t) of the i-th skeletal joint j
i
.
Every skeletal joint j
i
represents a distinct and precise arti-
culation or part of one’s hand in the physical world.
In the following subsections, we present a short review
of some approaches to gesture recognition. Typical ap-
proaches to hand gesture recognition begin with the ex-
traction of spatial and temporal features from raw data.
The features are later classified by a Machine Learning
algorithm. The feature extraction step can either be ex-
plicit, using hand-crafted features known to be useful for
classification, or implicit, using (machine) learned features
that describe the data without requiring human labor or ex-
pert knowledge. Deep Learning algorithms leverage such
learned features to obtain hierarchical representations (fea-
tures) that often describe the data better than hand-crafted
features. As we work on skeletal data only, with a deep-
learning perspective, this review pays limited attention to
non deep-learning based approaches and to depth-based
approaches ; a survey on the former approaches can be
found in [19] while several recent surveys on the latter ap-
proaches are listed in Neverova’s thesis [21].
2.1 Non-deep-learning methods using hand-
crafted features
Various hand-crafted representations of skeletal data can
be used for classification. These representations often des-
cribe physical attributes and constraints, or easily interpre-
table properties and correlations of the data, with an em-