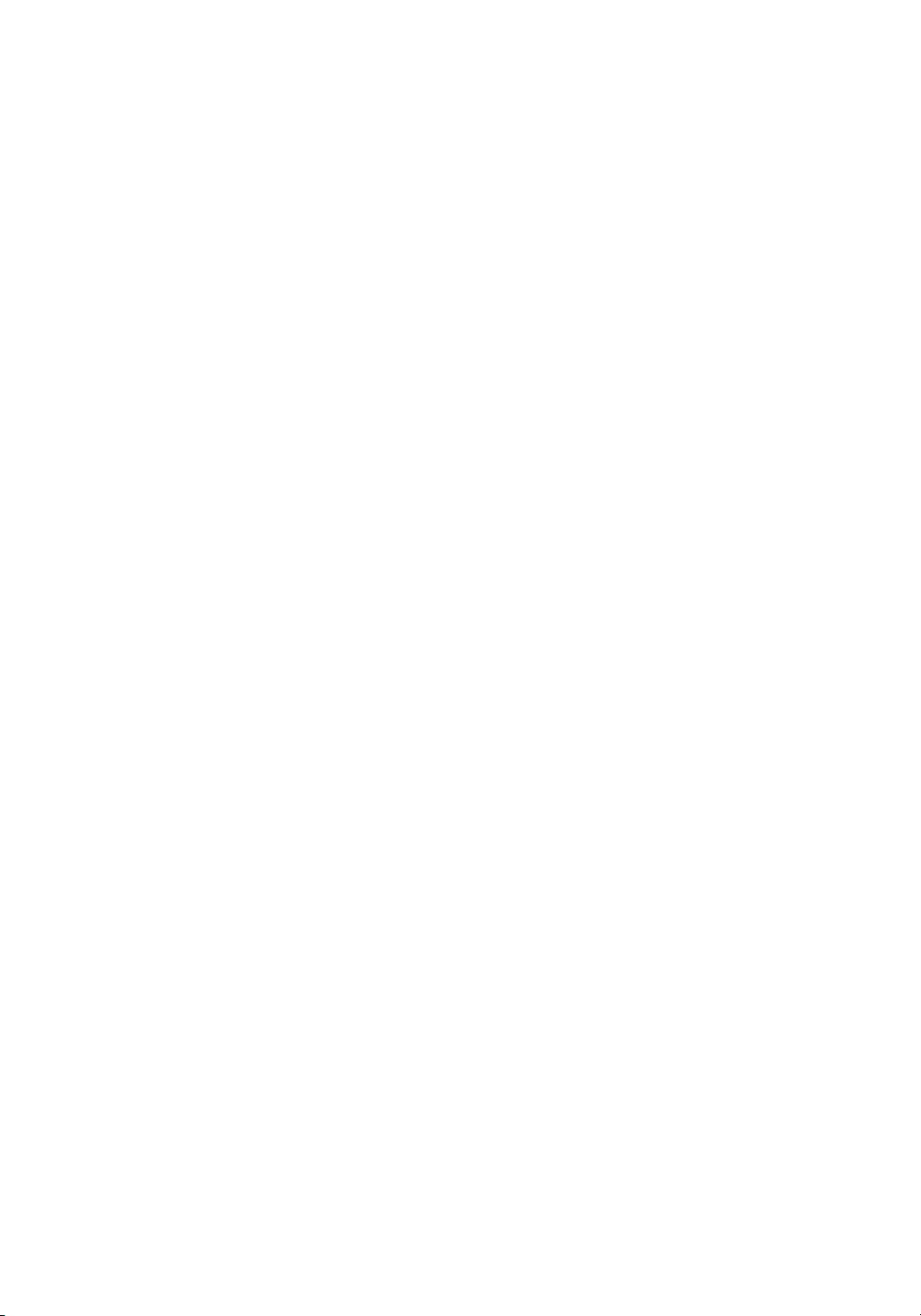
5
Generative Adversarial Networks 5
Once more, GANs comprise of two sections: generators and discriminators. The gen-
erator model produces engineered models (e.g., images) from arbitrary noise sampled
utilizing a distribution model, which alongside genuine models from a preparation infor-
mational collection are taken care of to the discriminator, which endeavors to recognize the
two. Both the generator and discriminator work on in their particular capacities until the
discriminator can’t perceive the genuine models from the integrated models with better
than the half precision expected of possibility.
GANs are algorithmic plans that utilize two brain afliations, setting one as opposed
to the accompanying to make new, created cases of information that can be pass with
genuine information. They are utilized regularly in picture age, video age and voice age.
Generative adversarial networks embraces up a game-hypothetical strategy, rather than
standard brain organizations. The organizations advance out a viable method for making
from a preparation appropriation through a two-player game. The two enemies are gen-
erator and discriminator. These two foes are in reliable ght all through the arranging cor-
respondence. Since an ill-disposed learning technique is taken on, we genuinely need not
care about approximating obstinate thickness capacities.
One brain organization, called the generator, delivers new data events, while the other,
the discriminator, evaluates them for validness; for instance, the discriminator closes
whether each instance of data that it reviews has a spot with the certiable planning
dataset or not.
As you can recognize from their names, a generator is used to make genuine looking
pictures and the discriminator’s liability is to perceive which one is phony. The
components/enemies are in a consistent battle as one (generator) endeavors to deceive the
other (discriminator), while various takes the necessary steps not to be deceived. To make
the best pictures, you will require a marvelous generator and a discriminator. This is since,
in such a case that your generator isn’t sufcient, it will not at any point have the choice to
deceive the discriminator and the model will not at any point join together. Accepting the
discriminator is terrible, then, picture which has no perceptible pattern will similarly be
named authentic and in this manner your model never plans and consequently you never
convey the best outcome. The information and unpredictable upheaval can be a Gaussian
appointment and characteristics can be inspected from this course and dealt with into the
generator association and a picture is created. This made the picture differentiated and a
genuine picture by the discriminator and it endeavors to perceive if the given picture is
phony or certied.
1.3.1 Image-to-Image Translation
GAN-based methods have been extensively used in picture to-picture interpretation and
conveyed drawing in results. Inpix2pix, contingent GAN (cGAN), was used to get to know
a preparation from an info picture to a result picture; cGAN gains a prohibitive generative
model using matched pictures from source and target regions. CycleGAN was utilized
for picture to-picture interpretation endeavors in the nonparticipation of joined models. It
takes in a preparation from a source region A to a goal space B by introducing two cycle-
consistency disasters. Likewise, DiscoGAN and DualGAN use an independent learning
approach for picture-to-picture interpretation reliant upon unaided data, yet with different
misfortune capacities. Consonant GAN utilizes for solo picture to-picture understanding
presents spatial smoothing to maintain unsurprising mappings during translation.
Image-to-image interpretation is to take in a preparation between pictures from a source
region and pictures from an objective region and has various applications including picture