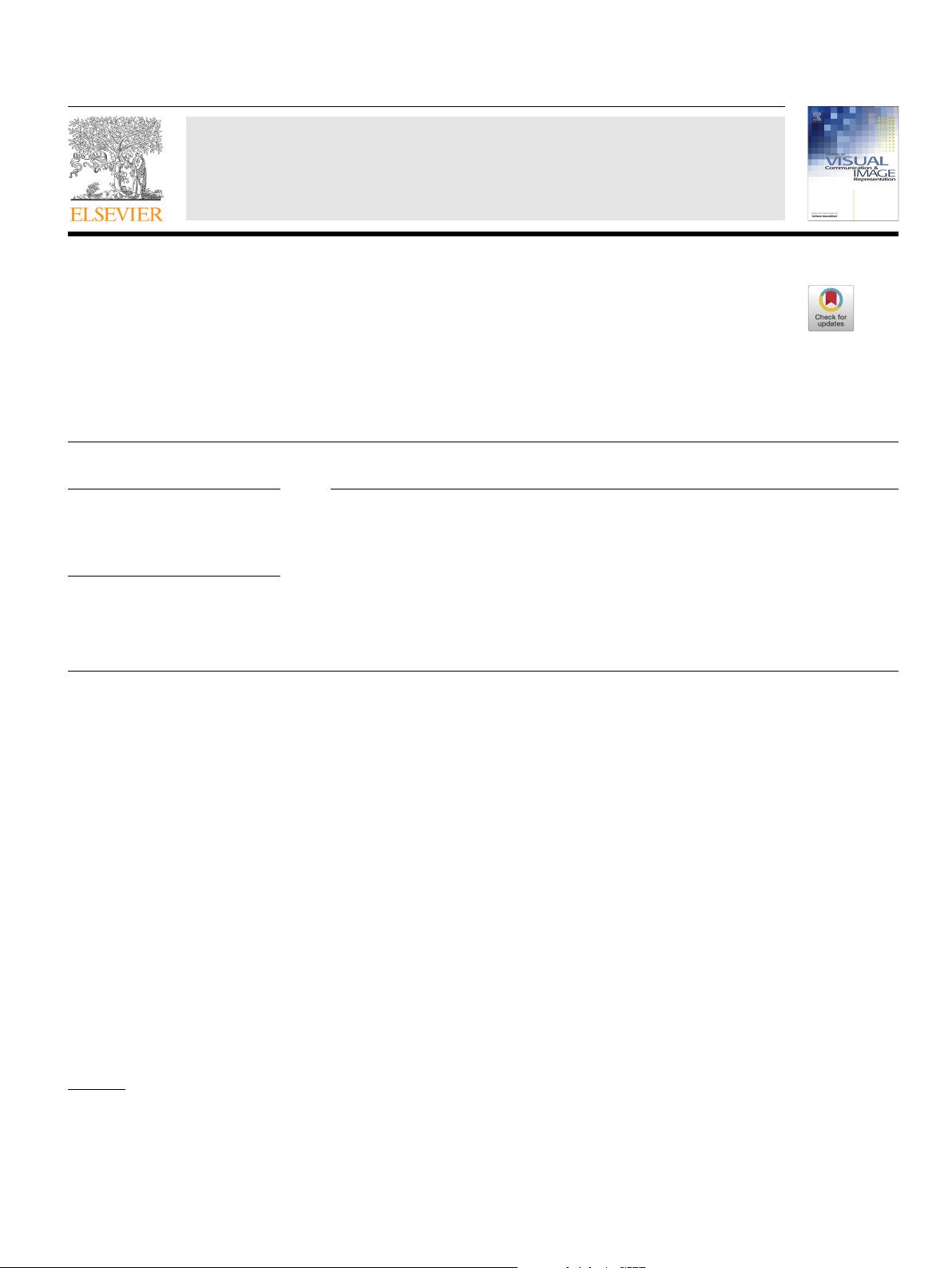
Weighted locality collaborative representation based on sparse
subspace
q
Xiao Dong
a
, Huaxiang Zhang
a,b,
⇑
, Lei Zhu
a,b
, Wenbo Wan
a,b
, Zhenhua Wang
a,b
,
Qiang Wang
a,b
, Peilian Guo
a,b
, Hui Ji
a
, Jiande Sun
a,b
a
School of Information Science and Engineering, Shandong Normal University, Jinan 250014, Shandong Province, China
b
Institute of Data Science and Technology, Shandong Normal University, Jinan 250014, Shandong Province, China
article info
Article history:
Received 2 February 2017
Revised 1 March 2018
Accepted 18 November 2018
Available online 23 November 2018
Keywords:
Collaborative representation
Sparse subspace
Linear representation
Face recognition
abstract
This paper takes into account both unlabeled data and their local neighbors to learn their sparse repre-
sentations, and proposes a face recognition approach named Weighted Locality Collaborative
Representation Classifier based on sparse subspace (WLCRC). WLCRC firstly learns a subset of the original
training data to build a much correlated dictionary, and then combines linear regression techniques
together with weighted collaborative representation techniques to optimize the linear reconstruction
of unlabeled data. It uses the newly built dictionary to learn the reconstruction coefficients for each unla-
beled datum while considering the influence of its local neighbors. Classifications are performed accord-
ing to the reconstruction residuals, and experimen tal results on benchmark datasets demonstrate that
WLCRC is effective.
Ó 2018 Elsevier Inc. All rights reserved.
1. Introduction
Benefiting from the power of compressive sensing [1], sparse
representation (SR) techniques are widely used to find the poten-
tial linear relationships among data, and demonstrates impressive
performance on face recognition problems [2,3]. Although SR [4]
succeeds in face recognition problems, is its performance deter-
mined by the sparsity property of the coefficients? Zhang et al.
[5] proposed a new method named collaborative representation
(CR) which is faster than SR for obtaining the optimal solutions,
while its performance is comparable with SR. Since SR and CR
demonstrate similar performance, successfully making full use of
the merits of both linear representation methods may be helpful
to improve the recognition performance. Zhang et al. [6] proposed
a method named RBCM by integrating sparse representation clas-
sifier (SRC) with the residuals of collaborative representation clas-
sifier (CRC) for performance improvement, and Li et al. [7]
simultaneously used SR and CR for target detections. Zeng et al.
[8] computed the weighted average of the representation coeffi-
cients obtained by SR and CR and proposed a weighted average
integration of sparse representation and collaborative representa-
tion approach for face recognition. Besides, Jiang et al. [9] used a
new label consistency constraint to enforce discriminability in
sparse codes for better performance. Jin et al. [10] proposed a
new method by combining R-CRC [11] and LLC [12] to improve
the recognition rate. Yang et al. [13] used regression analysis to
solve the occlusion and illumination problem for the better perfor-
mance. Although deep learning techniques, such as convolutional
neural network (CNN) [14–17], have been widely applied to com-
puter vision, they need a large number of training data. Manifold
learning (ML) [18–22] and nonnegative matrix factorization
(NMF) techniques [23–26] have also been widely used for face
recognition, but to some extent, they lose some discriminative
information of the original data when performing dimensionality
reduction. The sparse coefficients of SR and CR show that large
ones indicate strong data correlations, and it is assumed that data
belonging to the same class exist in the same subspace [27], so the
sparse model and the subspace model can be combined for perfor-
mance improvement.
Local properties of data are decisive for classification, and
should be considered in classification models. Linear regression
classification (LRC) [28] performs well on face recognition, and
can be combined with weighted collaborative representation clas-
sifier (WCRC) [29] to obtain much discriminative representation
coefficients. In this paper, based on the previous research [30],
we use LRC to obtain the sparse neighborhood for the unlabeled
samples, and use WCRC to reconstruct the unlabeled samples. In
https://doi.org/10.1016/j.jvcir.2018.11.030
1047-3203/Ó 2018 Elsevier Inc. All rights reserved.
q
This paper has been recommended for acceptance by Zicheng Liu.
⇑
Corresponding author at: School of Information Science and Engineering,
Shandong Normal University, Jinan 250014, Shandong Province, China.
E-mail addresses: huaxzhang@163.com, huaxzhang@hotmail.com (H. Zhang).
J. Vis. Commun. Image R. 58 (2019) 187–194
Contents lists available at ScienceDirect
J. Vis. Commun. Image R.
journal homepage: www.elsevier.com/locate/jvci