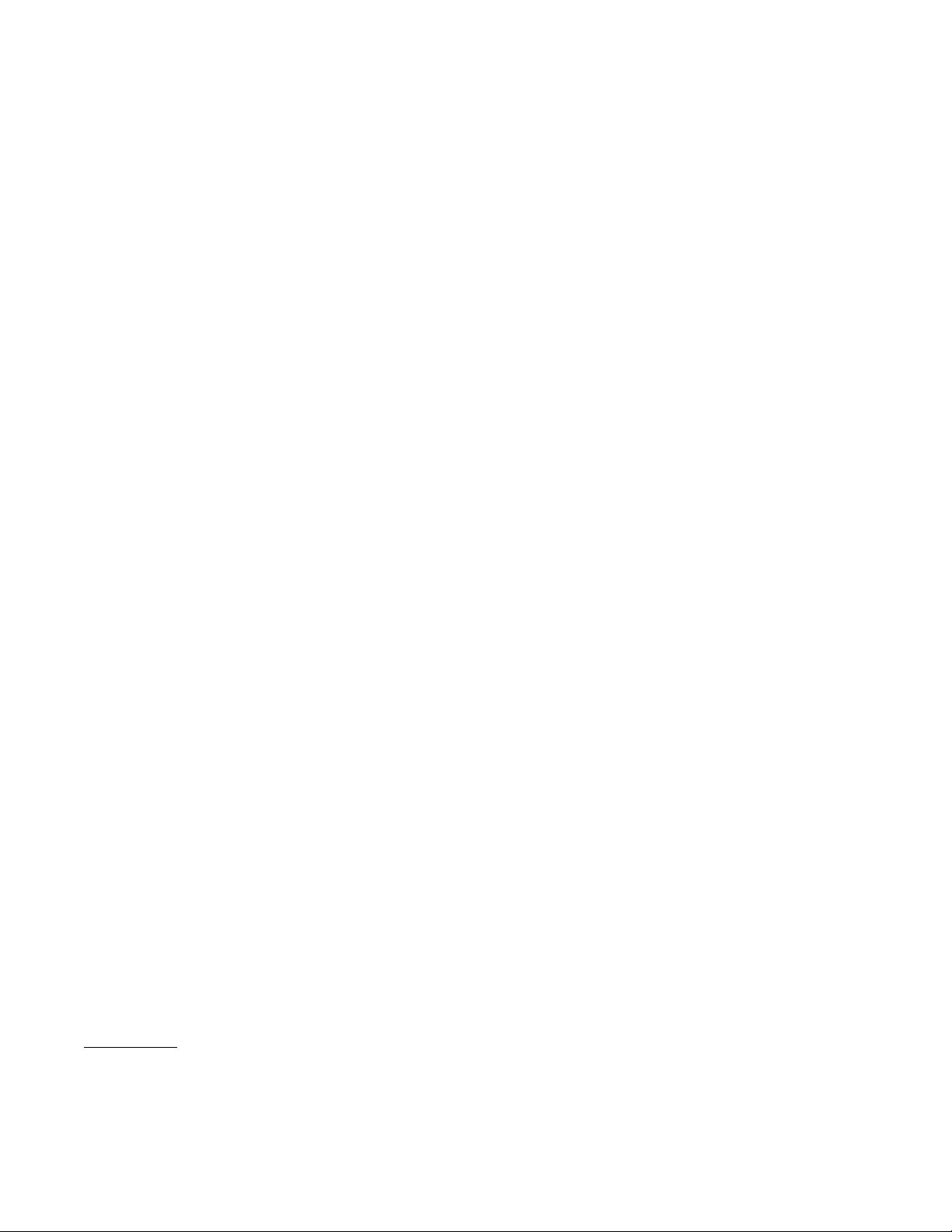
CMCGAN: A Uniform Framework for Cross-Modal Visual-Audio Mutual
Generation
Wangli Hao
1,4
, Zhaoxiang Zhang
1,2,3,4, ∗
, He Guan
1,4
1
Research Center for Brain-inspired Intelligence, CASIA
2
National Laboratory of Pattern Recognition, CASIA
3
CAS Center for Excellence in Brain Science and Intelligence Technology, CAS
4
University of Chinese Academy of Sciences
{haowangli2015,zhaoxiang.zhang,guanhe2015}@ia.ac.cn
Abstract
Visual and audio modalities are two symbiotic modalities un-
derlying videos, which contain both common and comple-
mentary information. If they can be mined and fused suffi-
ciently, performances of related video tasks can be signifi-
cantly enhanced. However, due to the environmental inter-
ference or sensor fault, sometimes, only one modality exists
while the other is abandoned or missing. By recovering the
missing modality from the existing one based on the com-
mon information shared between them and the prior informa-
tion of the specific modality, great bonus will be gained for
various vision tasks. In this paper, we propose a Cross-Modal
Cycle Generative Adversarial Network (CMCGAN) to han-
dle cross-modal visual-audio mutual generation. Specifical-
ly, CMCGAN is composed of four kinds of subnetworks:
audio-to-visual, visual-to-audio, audio-to-audio and visual-
to-visual subnetworks respectively, which are organized in
a cycle architecture. CMCGAN has several remarkable ad-
vantages. Firstly, CMCGAN unifies visual-audio mutual gen-
eration into a common framework by a joint corresponding
adversarial loss. Secondly, through introducing a latent vec-
tor with Gaussian distribution, CMCGAN can handle dimen-
sion and structure asymmetry over visual and audio modali-
ties effectively. Thirdly, CMCGAN can be trained end-to-end
to achieve better convenience. Benefiting from CMCGAN,
we develop a dynamic multimodal classification network to
handle the modality missing problem. Abundant experiments
have been conducted and validate that CMCGAN obtains
the state-of-the-art cross-modal visual-audio generation re-
sults. Furthermore, it is shown that the generated modality
achieves comparable effects with those of original modality,
which demonstrates the effectiveness and advantages of our
proposed method.
Video mainly contains two symbiotic modalities, the visu-
al and the audio ones. Information embedded in these two
modalities owns both common and complementary informa-
tion respectively. Common information can make the trans-
lation over visual and audio modalities be possible. Mean-
while, complementary information can be adopted as the pri-
or of one modality to facilitate the associative tasks. Thus,
sufficient utilization of these common and complementary
information will further boost the performances of related
∗
Corresponding author. (zhaoxiang.zhang@ia.ac.cn)
Copyright
c
2018, Association for the Advancement of Artificial
Intelligence (www.aaai.org). All rights reserved.
video tasks. However, due to the environmental disturbance
and sensor fault, one of the modality may be missing or dam-
aged, which will bring significant inconveniences such as
silent film and screen blurred. If we can restore the missing
modality from the remaining modality based on the cross-
modal prior, great bonus will be gained for various multime-
dia tasks and many traditional single-modal databases can
be reused in conjunction to gain better performance.
Generative Adversarial Networks (GANs) have gained
extraordinary popularity because of their ability in generat-
ing high-quality realistic samples, which is superior to oth-
er generative models. Compared to numerous work focus-
ing on static information translation, such as image-to-image
(Isola et al. 2016; Zhu et al. 2017) and text-to-image (Reed
et al. 2016), few of methods concern dynamic visual-audio
modality conversion and generation. Chen et al. firstly de-
sign Conditional GANs for cross-modal visual-audio gen-
eration. Drawbacks of their work are that the mutual gen-
eration process relies on different models and it cannot be
trained end-to-end.
Inspired by (Isola et al. 2016; Zhu et al. 2017), we propose
Cross-Modal Cycle Generative Adversarial Network (CM-
CGAN) to achieve cross-modal visual-audio mutual gener-
ation. Compared to CycleGAN, CMCGAN introduces a la-
tent vector to handle dimension and structure asymmetry a-
mong different modalities. Moreover, another two genera-
tion paths are coupled with CycleGAN to facilitate cross-
modal visual-audio translation and generation. Finally, a
joint corresponding adversarial loss is designed to unify the
visual-audio mutual generation in a common framework. In
addition, CMCGAN can be trained end-to-end to obtain bet-
ter convenience.
Benefiting from CMCGAN, a dynamic multimodal clas-
sification network is developed for double modalities. Once
only single modal as input, we will supplement the absent
one in the aid of CMCGAN and then perform the subsequent
classification task. In summary, we make the following con-
tributions:
• We propose a Cross-Modal Cycle Generative Adversari-
al Network(CMCGAN) to simultaneously handle cross-
modal visual-audio mutual generation in the same model.
• We develop a joint adversarial loss to unify visual-audio
mutual generation, which makes it possible not only to