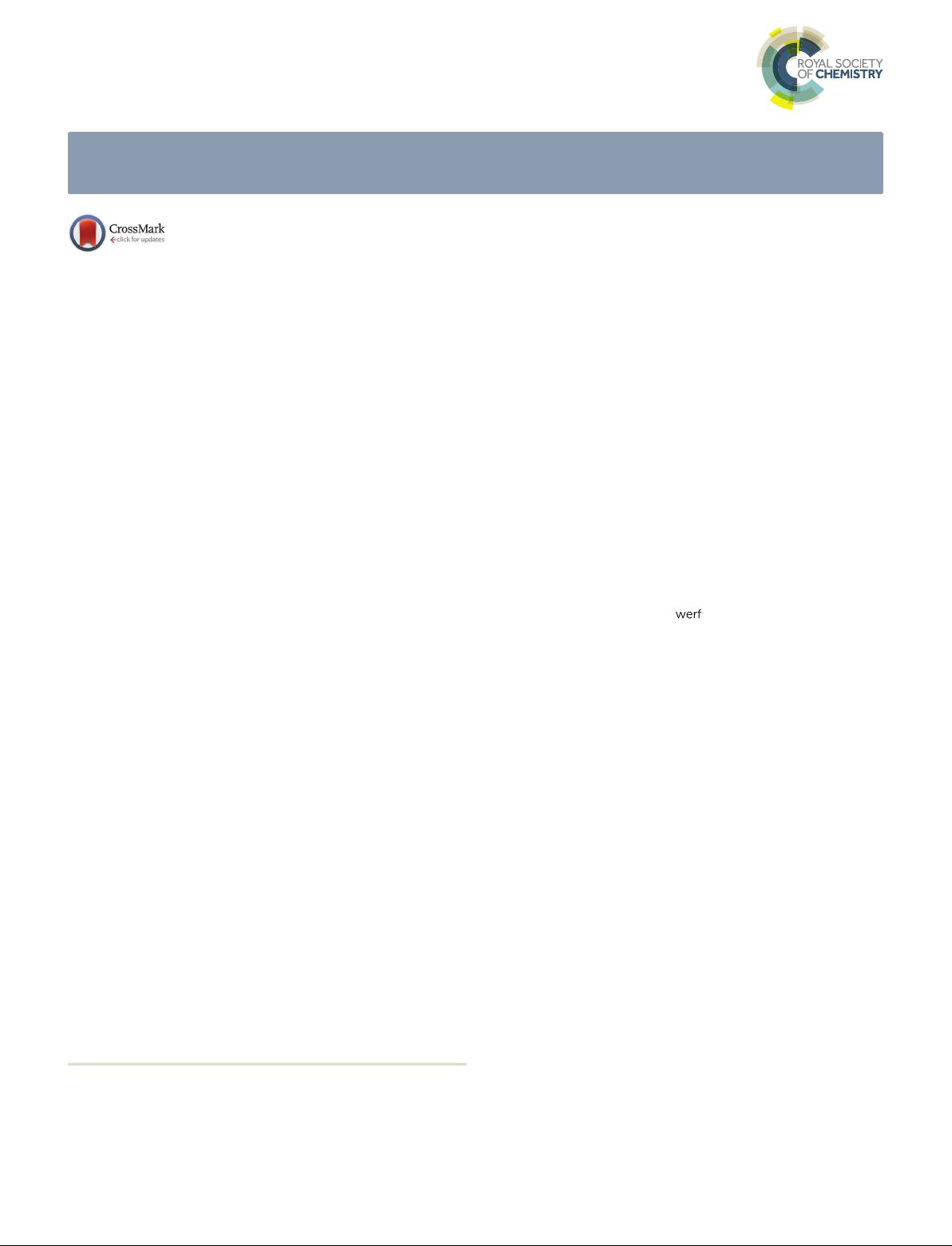
892 | Mol. BioSyst., 2015, 11,892--897 This journal is
©
The Royal Society of Chemistry 2015
Cite this: Mol. BioSyst., 2015,
11,892
lncRNA-MFDL: identification of human long
non-coding RNAs by fusing multiple features and
using deep learning†
Xiao-Nan Fan and Shao-Wu Zhang*
Long noncoding RNAs (lncRNAs) are emerging as a novel class of noncoding RNAs and potent gene
regulators, which play an important and varied role in cellular functions. lncRNAs are closely related with
the occurrence and development of some diseases. High-throughput RNA-sequencing techniques
combined with de novo assembly have identified a large number of novel transcripts. The discovery of
large and ‘hidden’ transcriptomes urgently requires the development of effective computational methods
that can rapidly distinguish between coding and long noncoding RNAs. In this study, we developed a
powerful predictor (named as lncRNA-MFDL) to identify lncRNAs by fusing multiple features of the open
reading frame, k-mer, the secondary structure and the most-like coding domain sequence and
using deep learning classification algorithms. Using the same human training dataset and a 10-fold
cross validation test, lncRNA-MFDL can achieve 97.1% prediction accuracy which is 5.7, 3.7, and 3.4%
higher than that of CPC, CNCI and lncRNA-FMFSVM predictors, respectively. Compared with CPC and
CNCI predictors in other species (e.g., anole lizard, zebrafish, chicken, gorilla, macaque, mouse, lamprey,
orangutan, xenopus and C. elegans) testing datasets, the new lncRNA-MFDL predictor is also much more
effective and robust. These results show that lncRNA-MFDL is a powerful tool for identifying lncRNAs.
The lncRNA-MFDL software package is freely available at http://compgenomics.utsa.edu/lncRNA_MDFL/
for academic users.
Introduction
A mass of evidence reveals that B98% of the genome can be
transcribed, of which only B2% encodes protein genes,
1,2
and
a majority of unexpected noncoding transcripts have also been
identified.
3
Therefore the vast majority of these unexpected
transcripts, sometimes referred to as ‘‘dark matter’’,
4,5
have
drawn a great deal of attention. In the mammalian noncoding
transcriptome, long noncoding transcripts (4200 nt) appear to
comprise the largest portion, and show critical roles in diverse
regulatory levels, such as transcriptional regulation and post-
transcriptional regulation.
6,7
With the development of high-throughput next-generation
sequencing techniques, more and more novel transcripts have
been generated. The desire to develop computational methods
for efficiently and effectively identifying noncoding RNA has led
to the development of theoretical and computational methods
in the recent few years. These approaches such as CONC (Coding
Or Non-Coding),
8
CPC (Coding Potential Calculator),
9
PORTRAIT,
10
PhyloCSF
11
and CPAT
12
typically identify noncoding genes that
have short open reading frames (ORFs) and are less homologous
with protein-coding genes.
13
However, they are not suitable for
identifying long noncoding RNAs (lncRNAs), because lncRNAs may
contain long putative ORFs or short protein-like sub-sequences.
14,15
Recently, several approaches and tools,
8,16–18
have been developed
to identify lncRNAs. CNCI
8
extracted five features (i.e. the length
and S-score of MLCDS, length-percentage, score-distance and
codon-bias) by profiling adjoining nucleotide triplets and used
the support vector machine (SVM) to distinguish protein-coding
and long noncoding RNA sequences, but it did not consider the
RNA structural information. Lv et al.
16
used the LASSO regular-
ized logistic regression to select the chromatin and genomic
features to identify lncRNAs in mouse brain development;
however, relatively comprehensive chromatin data were only
available for a handful of tissues/cells and species, and this
method was not suitable for large-scale prediction of lncRNAs.
iSeeRNA
17
used the SVM model to identify the long intergenic
noncoding RNAs (lincRNAs) by integrating multiple features
(e.g. conservation, ORF, seven di- and tri-nucleotide sequence
frequencies). Wang et al.
18
used the GA-SVM algorithm to
Key Laboratory of Information Fusion Technology of Ministry of Education, School
of Automation, Northwestern Polytechnical University, Xi’an, 710072, China.
E-mail: zhangsw@nwpu.edu.cn; Tel: +86-29-88431308
† Electronic supplementary information (ESI) available. See DOI: 10.1039/
c4mb00650j
Received 4th November 2014,
Accepted 6th January 2015
DOI: 10.1039/c4mb00650j
www.rsc.org/molecularbiosystems
Molecular
BioSystems
PAPER