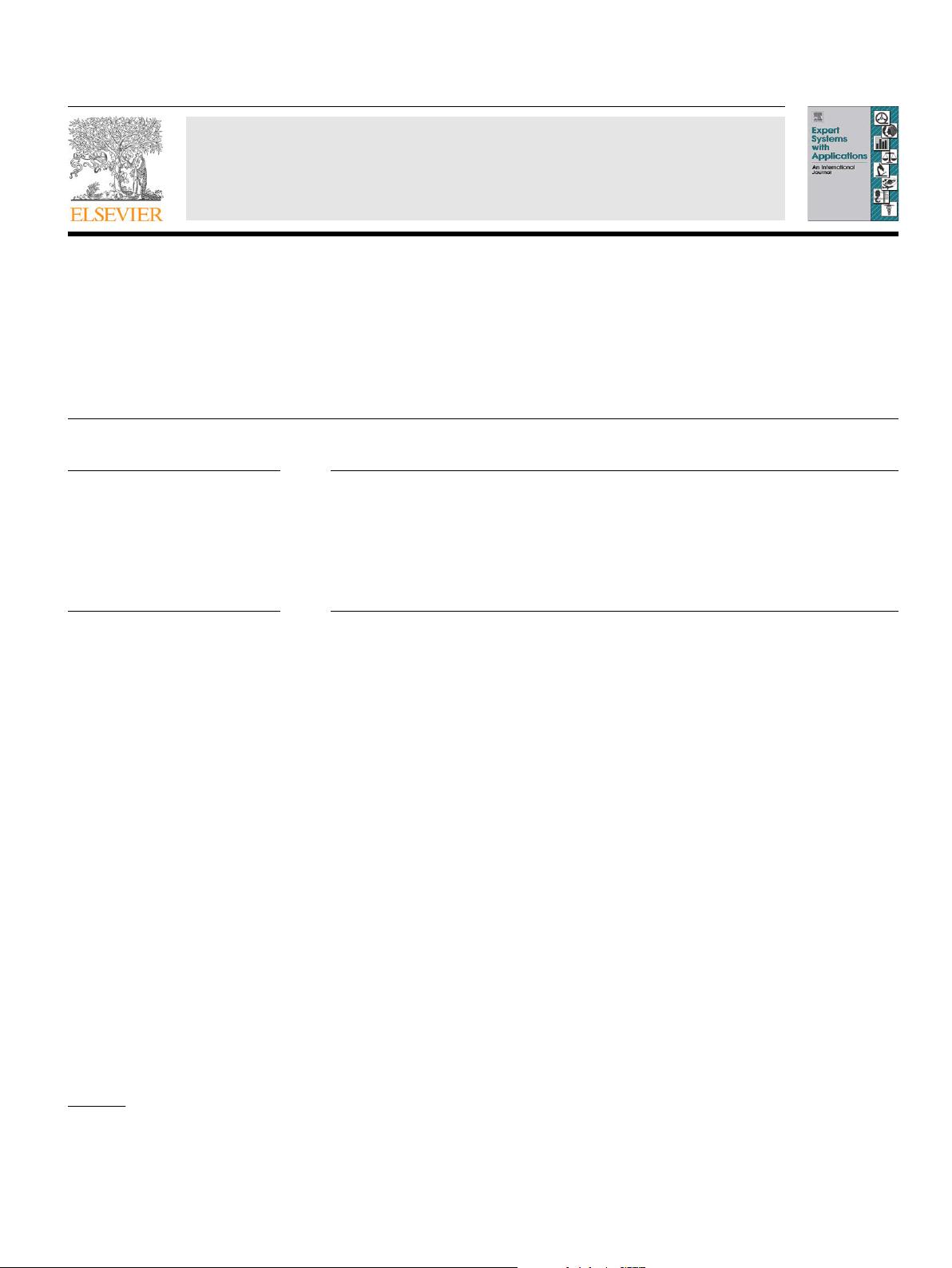
Improving forecasts of GARCH family models with the artificial neural networks:
An application to the daily returns in Istanbul Stock Exchange
Melike Bildirici
a,
*
, Özgür Ömer Ersin
b,1
a
Yıldız Technical University, Faculty of Economics and Business Administration, Department of Economics, Barbaros Bulvari, Besiktas,
_
Istanbul, Turkey
b
Yeditepe University, Faculty of Commerce, Department of International Trade and Business, Kadikoy, Kayisdagi,
_
Istanbul, Turkey
article info
Keywords:
Volatility
Stock returns
ARCH/GARCH
EGARCH
TGARCH
PGARCH
APGARCH
Artificial neural networks
abstract
In the study, we discussed the ARCH/GARCH family models and enhanced them with artificial neural net-
works to evaluate the volatility of daily returns for 23.10.1987–22.02.2008 period in Istanbul Stock
Exchange. We proposed ANN-APGARCH model to increase the forecasting performance of APGARCH
model. The ANN-extended versions of the obtained GARCH models improved forecast results. It is note-
worthy that daily returns in the ISE show strong volatility clustering, asymmetry and nonlinearity
characteristics.
Ó 2008 Elsevier Ltd. All rights reserved.
1. Introduction
The importance of volatility has led to the development and
application of many significant econometric models and found
important application areas in financial markets as a result of both
the need of modeling the uncertainty and the risk in financial asset
returns. The three most significant characteristics of returns in
financial assets could be stated as the following: volatility clustering
property, as a result of the volatility changes over time in magni-
tude and in cases where prices hardly change but volatility in-
creases in sizes of large clusters; asymmetric relation property of
volatility to past return shocks (Engle & Ng, 1993; Glosten, Jagan-
nathan, & Runkle, 1993; Nelson, 1991, 1992); and nonlinearity
property, the path of volatility reacts differently in different re-
gimes (Klaassen, 2002; Kramer, 2006).
Engle (1982) ARCH models and Bollerslev (1986) GARCH mod-
els found many important applications in the financial markets.
As a result, GARCH models respond to the need felt for a foresight
method that takes into account various properties of the probabil-
ity distribution of return series of financial variables and had been
used intensively in academic studies. Due to this effect, asymmet-
ric GARCH models have rapidly expanded (Nelson, 1991). While
Nelson (1991) developed Exponential GARCH (EGARCH) model,
Zakoian (1994) and Glosten et al., 1993 working independent of
one another developed the GJR-GARCH model. Zakoian (1994)
introduced the Threshold GARCH (TGARCH) model and Sentana
(1995) introduced the Quadratic GARCH (QGARCH) model.
GARCH models of Taylor (1986) and Schwert (1989) relate the
conditional standard deviation of a series and past standard devia-
tions of a different property compared to other models. The model
was generalized by Ding, Granger, and Engle (1993) as Power
GARCH. This study can be considered as the basis of the APGARCH
literature. Hentschel (1995) has applied his study, in which he pro-
poses a more general model of the Power ARCH model, to US stock
market data. Tse and Tsui (2002) determined the APGARCH model.
Brooks, Faff, McKenzie, and Mitchell (2000) show the leverage ef-
fect in the model and the usefulness of including a free power
term.
In this study, we aim to analyze the volatility of stock return
behavior of Istanbul Stock Exchange ISE 100 Index for the
23.10.1987–22.02.2008 period. The study will compare and com-
bine a general class of Autoregressive Conditional Heteroscedastic-
ity (G)ARCH family models (Bollerslev, 1986; Engle, 1982; Nelson,
1991) of GARCH, EGARCH, GJR-GARCH, TGARCH, NGARCH, SA-
GARCH, PGARCH, APGARCH, NPGARCH with Artificial Neural Net-
work models and discuss and compare them in accordance with
their forecast capabilities. The study is organized into the following
sections: Theory and Data Characteristics, Econometric Results and
lastly, Conclusion.
2. Theory
ANN models have found many important applications espe-
cially in the field of financial modeling and forecasting in the re-
cent literature. Among many, Abhyankar, Copeland, and Wang
(1997), Castiglione (2001), Freisleben (1992), Kim and Chun
0957-4174/$ - see front matter Ó 2008 Elsevier Ltd. All rights reserved.
doi:10.1016/j.eswa.2008.09.051
* Corresponding author.
E-mail addresses: bildiri@yildiz.edu.tr (M. Bildirici), oersin@yeditepe.edu.tr
(Ö.Ö. Ersin).
1
Tel.: +90 216 578 0956.
Expert Systems with Applications 36 (2009) 7355–7362
Contents lists available at ScienceDirect
Expert Systems with Applications
journal homepage: www.elsevier.com/locate/eswa