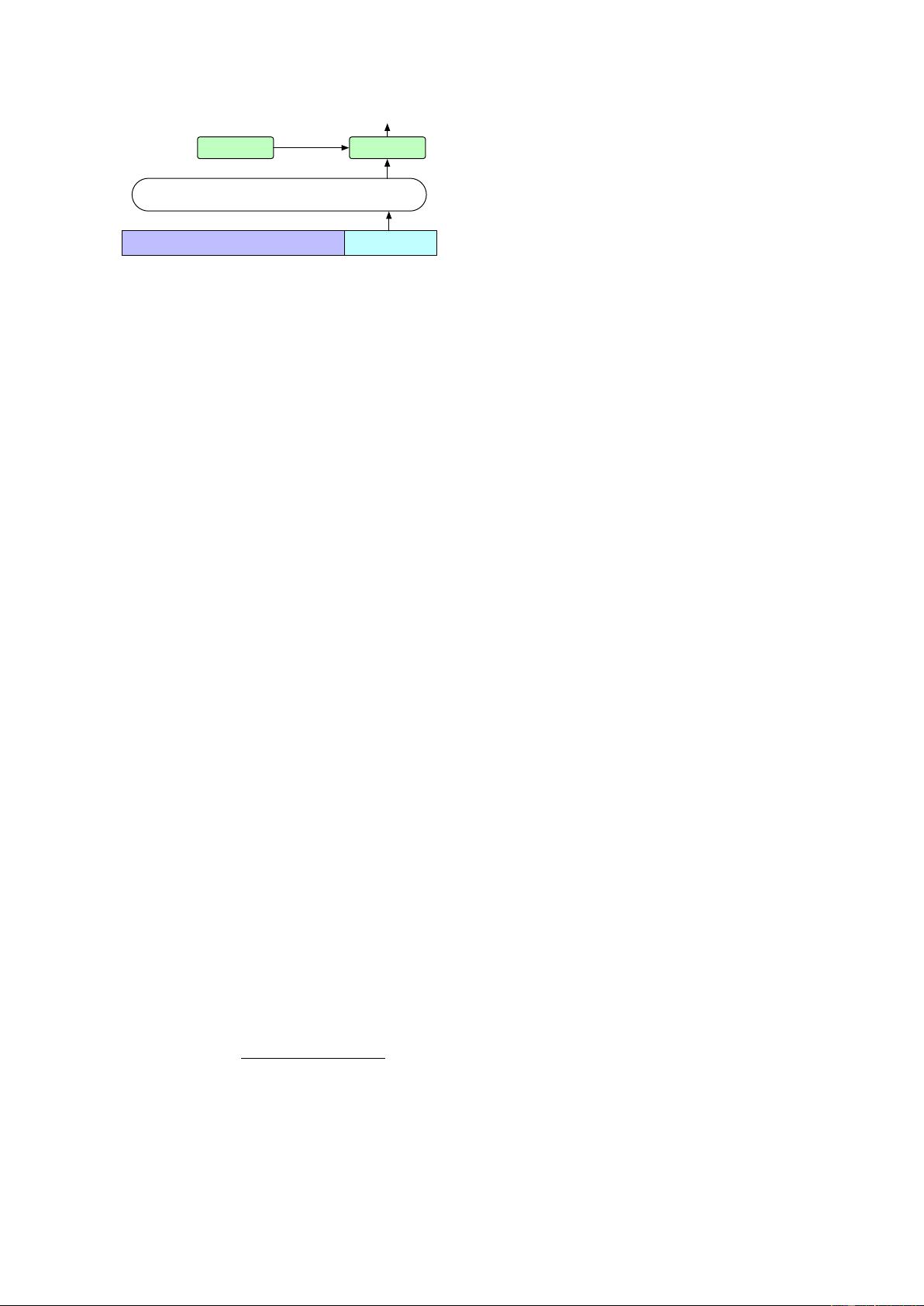
Coronet has the best lines of all day cruisers.
Positive
<latexit sha1_base64="cb5S93r+RGEy3gKCUaUf2i3SjJQ=">AAAB6HicbVDJSgNBEK2JW4xb1KMijUHwFGY8qMegF48JmAWSIfR0apI2PQvdPcIw5OjJiwdFvPoV+Q5vfoM/YWc5aPRBweO9KqrqebHgStv2p5VbWl5ZXcuvFzY2t7Z3irt7DRUlkmGdRSKSLY8qFDzEuuZaYCuWSANPYNMbXk/85j1KxaPwVqcxugHth9znjGoj1dJusWSX7SnIX+LMSalyOK59PRyNq93iR6cXsSTAUDNBlWo7dqzdjErNmcBRoZMojCkb0j62DQ1pgMrNpoeOyIlResSPpKlQk6n6cyKjgVJp4JnOgOqBWvQm4n9eO9H+pZvxME40hmy2yE8E0RGZfE16XCLTIjWEMsnNrYQNqKRMm2wKJgRn8eW/pHFWds7Lds2kcQUz5OEAjuEUHLiACtxAFerAAOERnuHFurOerFfrbdaas+Yz+/AL1vs31mKQqQ==</latexit>
<latexit sha1_base64="CZ8abL6hJorh4jxHBPAGp8NppAA=">AAAB63icbVBNSwMxEJ2tX7V+VT16CS1CRSi7HtRj0YvHCvYD2qVk07QNTbJLki0sS/+CFwVFvPqHvPXfmG170NYHA4/3ZpiZF0ScaeO6Mye3sbm1vZPfLeztHxweFY9PmjqMFaENEvJQtQOsKWeSNgwznLYjRbEIOG0F4/vMb02o0iyUTyaJqC/wULIBI9hk0qSSXPSKZbfqzoHWibck5Vqpe/k6qyX1XvG72w9JLKg0hGOtO54bGT/FyjDC6bTQjTWNMBnjIe1YKrGg2k/nt07RuVX6aBAqW9Kgufp7IsVC60QEtlNgM9KrXib+53ViM7j1Uyaj2FBJFosGMUcmRNnjqM8UJYYnlmCimL0VkRFWmBgbT8GG4K2+vE6aV1Xvuuo+2jTuYIE8nEEJKuDBDdTgAerQAAIjeIY3eHeE8+J8OJ+L1pyznDmFP3C+fgCio5Dy</latexit>
<latexit sha1_base64="cIlXHKTMHL8y94GI+KZXnlT1K7g=">AAAB7XicbVDLSgNBEOyNrxhfUY9ehgQhIoRdD+ox6MVjBPOAZAmzk9lkzOzMMjMrLjH/4EEPinj1f7zlb5w8DppY0FBUddPdFcScaeO6Yyezsrq2vpHdzG1t7+zu5fcP6lomitAakVyqZoA15UzQmmGG02asKI4CThvB4HriNx6o0kyKO5PG1I9wT7CQEWysVI9L6dPjSSdfdMvuFGiZeHNSrBTapy/jSlrt5L/bXUmSiApDONa65bmx8YdYGUY4HeXaiaYxJgPcoy1LBY6o9ofTa0fo2CpdFEplSxg0VX9PDHGkdRoFtjPCpq8XvYn4n9dKTHjpD5mIE0MFmS0KE46MRJPXUZcpSgxPLcFEMXsrIn2sMDE2oJwNwVt8eZnUz8reedm9tWlcwQxZOIIClMCDC6jADVShBgTu4Rne4N2Rzqvz4XzOWjPOfOYQ/sD5+gFYz5H0</latexit>
<latexit sha1_base64="XknPsXXFT3s+dKVLzg736M6sfhc=">AAAB9XicbVC7TsMwFL3hWcKrwMgSUSExVQkDsCAqWBiLRB9SGyrHcVqrjh3ZDlBF/Q8WBh5i5TPYWRB/g9N2gJYjWT465175+AQJo0q77rc1N7+wuLRcWLFX19Y3Notb23UlUolJDQsmZDNAijDKSU1TzUgzkQTFASONoH+R+41bIhUV/FoPEuLHqMtpRDHSRrppB4KFahCbK7sfdoolt+yO4MwSb0JKZx/2afLyZVc7xc92KHAaE64xQ0q1PDfRfoakppiRod1OFUkQ7qMuaRnKUUyUn41SD519o4ROJKQ5XDsj9fdGhmKVRzOTMdI9Ne3l4n9eK9XRiZ9RnqSacDx+KEqZo4WTV+CEVBKs2cAQhCU1WR3cQxJhbYqyTQne9JdnSf2w7B2V3Su3VDmHMQqwC3twAB4cQwUuoQo1wCDhAZ7g2bqzHq1X6208OmdNdnbgD6z3H7R5lks=</latexit>
Figure 3: Formulation of the sentiment classification
task as blank infilling with GLM.
reconstructing them. It is an important difference
as compared to other models. For example, XL-
Net (Yang et al., 2019) encodes the original posi-
tion so that it can perceive the number of missing
tokens, and SpanBERT (Joshi et al., 2020) replaces
the span with multiple [MASK] tokens and keeps
the length unchanged. Our design fits downstream
tasks as usually the length of the generated text is
unknown beforehand.
2.3 Finetuning GLM
Typically, for downstream NLU tasks, a linear clas-
sifier takes the representations of sequences or to-
kens produced by pretrained models as input and
predicts the correct labels. The practices are differ-
ent from the generative pretraining task, leading to
inconsistency between pretraining and finetuning.
Instead, we reformulate NLU classification tasks
as generation tasks of blank infilling, following
PET (Schick and Schütze, 2020a). Specifically,
given a labeled example
(x, y)
, we convert the in-
put text
x
to a cloze question
c(x)
via a pattern
containing a single mask token. The pattern is writ-
ten in natural language to represent the semantics
of the task. For example, a sentiment classification
task can be formulated as “{SENTENCE}. It’s
really
[MASK]
”. The candidate labels
y ∈ Y
are
also mapped to answers to the cloze, called ver-
balizer
v(y)
. In sentiment classification, the labels
“positive” and “negative” are mapped to the words
“good” and “bad”. The conditional probability of
predicting y given x is
p(y|x) =
p(v(y)|c(x))
P
y
0
∈Y
p(v(y
0
)|c(x))
(3)
where
Y
is the label set. Therefore the probability
of the sentence being positive or negative is propor-
tional to predicting “good” or “bad” in the blank.
Then we finetune GLM with a cross-entropy loss
(see Figure 3).
For text generation tasks, the given context con-
stitutes the Part A of the input, with a mask token
appended at the end. The model generates the text
of Part B autoregressively. We can directly apply
the pretrained GLM for unconditional generation,
or finetune it on downstream conditional generation
tasks.
2.4 Discussion and Analysis
In this section, we discuss the differences between
GLM and other pretraining models. We are mainly
concerned with how they can be adapted to down-
stream blank infilling tasks.
Comparison with BERT (Devlin et al., 2019).
As pointed out by (Yang et al., 2019), BERT fails
to capture the interdependencies of masked tokens
due to the independence assumption of MLM. An-
other disadvantage of BERT is that it cannot fill in
the blanks of multiple tokens properly. To infer the
probability of an answer of length
l
, BERT needs
to perform
l
consecutive predictions. If the length
l
is unknown, we may need to enumerate all possible
lengths, since BERT needs to change the number
of [MASK] tokens according to the length.
Comparison with XLNet (Yang et al., 2019).
Both GLM and XLNet are pretrained with autore-
gressive objectives, but there are two differences
between them. First, XLNet uses the original posi-
tion encodings before corruption. During inference,
we need to either know or enumerate the length of
the answer, the same problem as BERT. Second,
XLNet uses a two-stream self-attention mechanism,
instead of the right-shift, to avoid the information
leak within Transformer. It doubles the time cost
of pretraining.
Comparison with T5 (Raffel et al., 2020).
T5
proposes a similar blank infilling objective to pre-
train an encoder-decoder Transformer. T5 uses
independent positional encodings for the encoder
and decoder, and relies on multiple sentinel tokens
to differentiate the masked spans. In downstream
tasks, only one of the sentinel tokens is used, lead-
ing to a waste of model capacity and inconsistency
between pretraining and finetuning. Moreover, T5
always predicts spans in a fixed left-to-right order.
As a result, GLM can significantly outperform T5
on NLU and seq2seq tasks with fewer parameters
and data, as stated in Sections 3.2 and 3.3.
Comparison with UniLM (Dong et al., 2019).
UniLM combines different pretraining objectives
under the autoencoding framework by changing the