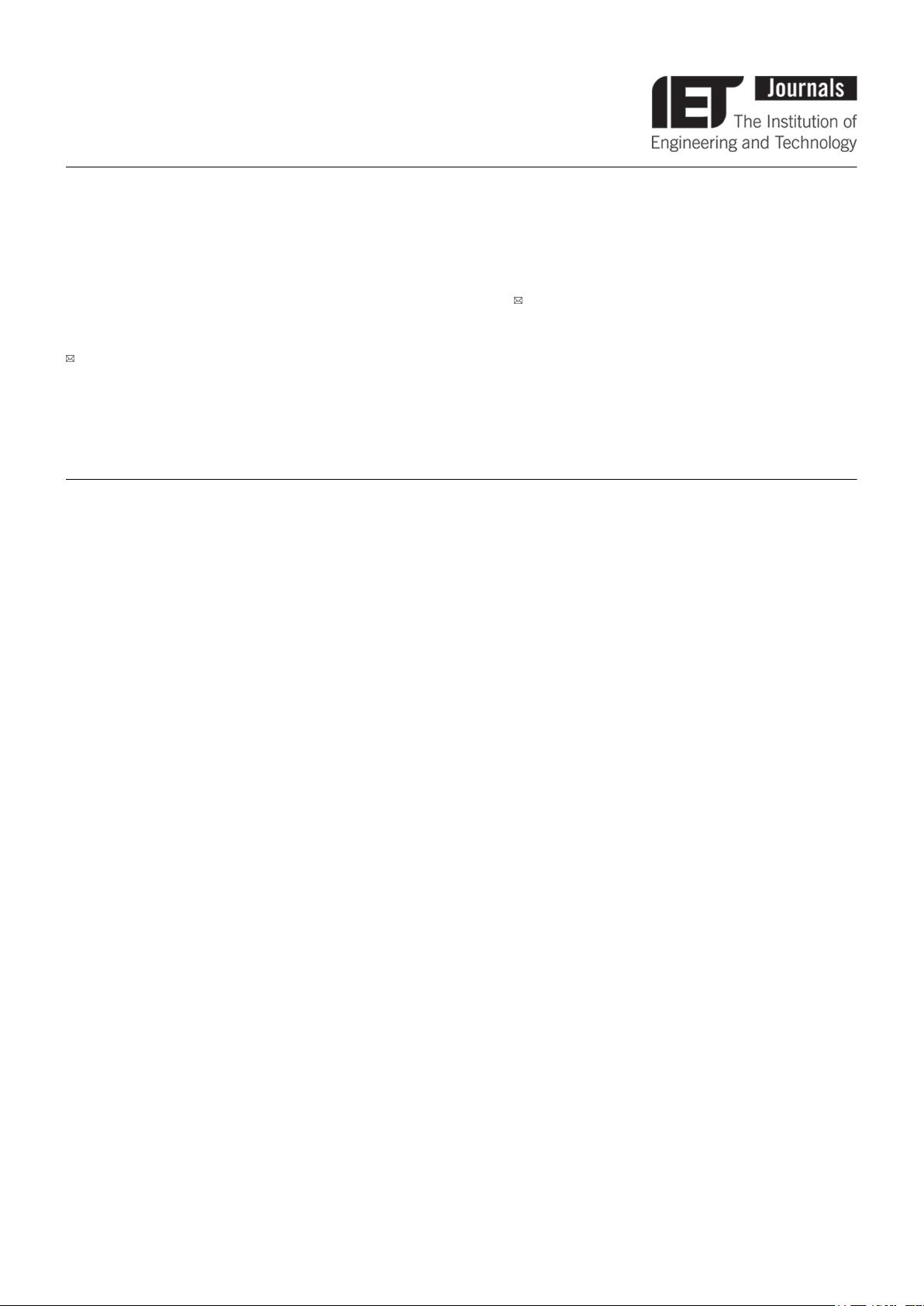
IET Radar, Sonar & Navigation
Research Article
Persymmetric Rao and Wald tests for adaptive
detection of distributed targets in compound-
Gaussian noise
ISSN 1751-8784
Received on 18th May 2016
Revised 5th October 2016
Accepted on 14th October 2016
E-First on 17th November 2016
doi: 10.1049/iet-rsn.2016.0251
www.ietdl.org
Xiaolu Guo
1,2
, Haihong Tao
1,2
, Hong-Yan Zhao
1,2
, Jun Liu
1,2
1
National Laboratory of Radar Signal Processing, Xidian University, Xi'an, People's Republic of China
2
Collaborative Innovation Center of Information Sensing and Understanding, Xidian University, Xi'an, People's Republic of China
E-mail: junliu@xidian.edu.cn
Abstract: The problem of detecting a distributed target in the presence of compound-Gaussian noise with unknown covariance
matrix is studied in this paper. Since no uniformly most powerful test exists for the problem at hand, two detectors based on the
Rao and Wald tests are devised. Remarkably, the persymmetric structure of the covariance matrix is exploited in the design of
the proposed detectors. Simulation results show that the proposed detectors outperform the traditional detectors, especially in
training-limited scenarios.
1 Introduction
The problem of adaptively detecting distributed targets has drawn
considerable attention recently [1–4]. A high-resolution radar
(HRR) can resolve a target into a group of scattering centres,
depending on the range extent of the target and the range resolution
capabilities of the radar. Moreover, it is well known that the point-
like target model may fail in many practical scenarios wherein a
low/medium resolution radar is employed [5]. When the target is
separated into a number of range cells, it is referred to as a
distributed or range-spread target.
Much work has been directed toward the detection of
distributed targets. For example, this detection problem has been
addressed in white Gaussian noise of known spectral level [6] or
known spectral structure [7]. In [8], Liu et al. examine the
detection of distributed targets in the presence of subspace
interference and Gaussian noise. Additionally, a model-based
adaptive detection approach for the range-spread targets has been
proposed in [9]. Most solutions to the distributed target detection
assume a homogeneous environment, i.e. the training samples
(secondary data) used for adaptation share the same covariance
matrix as the noise in the test cell (primary data).
Note that the homogeneity might not be met in realistic
scenarios [3, 10]. Therefore, the target detection in a non-
homogeneous background is actually a problem of primary
concern. In fact, the Gaussian assumption can be no longer met in
HRRs and/or at low grazing angles. More specifically, the noise is
usually described as a compound-Gaussian process [11]. This
process is mathematically expressed as the product of a texture and
a speckle. The texture is a temporally and spatially ‘slowly
varying’ component, accounting for the reflectivity of the
illuminated patch. While speckle is a ‘more rapidly’ varying
process [12, 13]. Note that the compound-Gaussian distribution
includes as special cases many distributions such as Weibull, log-
Weibull and K-distribution [14, 15].
Recently, many studies have been discussed on the distributed
target detection in the compound-Gaussian noise. On the basis of
the generalised likelihood ratio test (GLRT) principle, adaptive
detection schemes of range-spread targets in the compound-
Gaussian noise have been addressed partly in [16–18].
Furthermore, [19] provided the performance analysis of GLRT-
based adaptive detector for distributed targets in the compound-
Gaussian noise. The Rao and Wald tests for detecting distributed
targets in the compound-Gaussian noise has been discussed in [5],
where the texture is modelled as an unknown determinate
parameter. Some other related works can be seen in [20–22] and
the references therein. In these studies, sufficient training data are
usually assumed to be available. On the other hand, there is a
requirement of reducing the secondary data in adaptive detection.
In [23], the Bayesian Rao and Wald tests are proposed when only a
small number of training data is available. Both [24, 25] derived
Bayesian tests in non-homogeneous environments or
heterogeneous noise. In [26], a Bayesian space time adaptive
programming (STAP) algorithm is developed with knowledge-
aided in heterogeneous noise.
It is worth noting that the demanding requirement on the
number of secondary data maybe prohibitive in realistic scenarios.
A possible way to reduce the sample requirement is to exploit the
structural information of the noise covariance matrix. In radar
system, the noise covariance matrix has a persymmetric property if
a linear array is symmetrically spaced with respect to the phase
centre or the pulse train is uniformly spaced [27]. Several detection
schemes that take into account the persymmetric property are
developed [28–33]. A GLRT adaptive detector with persymmetric
property in homogeneous environments is proposed in [28]. In
partially homogeneous environments, the GLRT, Rao and Wald
tests have been proposed with exploiting persymmetry [29, 30].
For compound-Gaussian noise with unknown deterministic texture,
a persymmetric normalised matched filter detector is given in [32,
33]. A persymmetric GLRT detector in compound-Gaussian noise
with random texture has been proposed in [10]. Note that the
persymmetric detectors in [10, 28–33] are designed for the
detection of point-like targets. A persymmetric detector for
distributed targets in a partially homogeneous environment is
presented in [31]. An invariant approach which deals with
detection in Gaussian noise with a persymmetric covariance is
proposed in [34]. Persymmetric Rao and GLRT detectors are
devised for point-like target in Gaussian noise [35]. Nevertheless,
no persymmetric detector has been proposed for the distributed
target detection problem in compound-Gaussian noise.
In this paper, we propose two persymmetric detectors based on
the Rao and Wald tests for the problem of detecting a distributed
target in compound-Gaussian noise. The texture component in the
compound-Gaussian noise is assumed to follow an inverse-Gamma
distribution. This assumption has been verified in the real data
[36]. Note that the persymmetric structure has been employed in
our proposed detectors. Simulation results show that the proposed
persymmetric detectors outperform the counterparts, especially in
training-limited scenarios.
Note that the Rao and Wald tests are widely used, which are
asymptotically equivalent to the GLRT. Compared with the GLRT,
IET Radar Sonar Navig., 2017, Vol. 11 Iss. 3, pp. 453-458
© The Institution of Engineering and Technology 2016
453