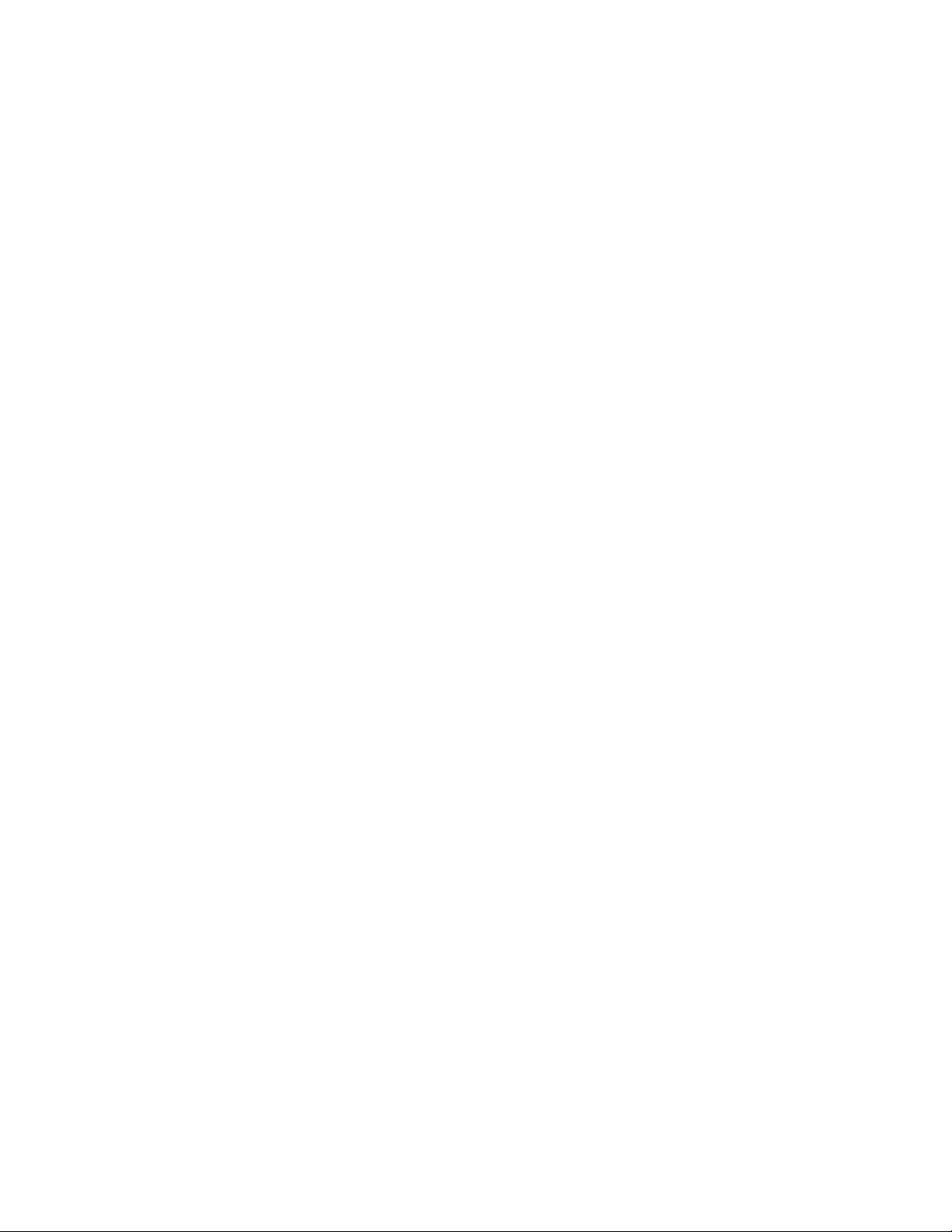
reconstruction of the sparse coefficients.
10
So far, this technique has been widely applied to SAR
image classification. Xing et al.
11
employed a representative feature vector to construct the
dictionary, instead of using the image pixel directly, in the SR classification model. Hou et al.
12
presented a hierarchical SR-based model for SAR image classification. This model utilizes the
features extracted from multisize patch around pixel to describe the complex terrains. Dong
et al.
13
developed an image classification method based on the joint sparse representation
(JSR) strategy, which was robust to noise corruption. In Ref. 14, the effective features were
first extracted and then used to construct an overcomplete dictionary. By measuring the residual
errors, this SR model could classify terrain targets for PolSAR images. Because the seriously
speckles and weak intensity differences in SAR images, however, many SR classifiers combin-
ing with weak discriminative features may not produce desired outputs.
10
As a means of achiev-
ing parsimonious representations in the context of regression and classification, sparse Bayesia n
learning (SBL) has been presented to solve this problem in the machine learning literature.
15–17
In Ref. 15, Wipf and Rao used an SBL framework to solve the signal processing problem of basis
selection. In Ref. 16, Zhang et al. applied SBL to recover spatiotemporal signals with satisfactory
recovery quality. Luo et al.
17
introduced two Bayesian correlated group regression methods to
measure the smaller reconstruction residuals. These approaches improve the classification results
significantly. Thus, SBL can be inherited in SAR image classification.
In addition, the methods using the fusion strategy can improve the interpretation results.
In Ref. 4, Tang and Jiao adopted a fusion similarity-based reranking method to enhance the
performance of SAR image retrieval. In Ref. 5, a region-based fuzzy matching measure was
developed to evaluate the similarities between SAR image patches, in which the different fuzzy
feature sets were fused to produce an estimate. Huan and Pan
18
used multiview decision fusion
strategies to classify the ground vehicles. Due to the existence of intravariation and interambi-
guity for different targets, however, the performance of these methods using similarity and deci-
sion fusion may decrease. Thus, it is difficult to accurately classify targets having large intraclass
variations. Recent studies have demonstrated that the methods that make use of the subcatego-
rization scheme can significantly improve the classification accuracy.
19–21
In Ref. 19, a subclass
scheme was utilized to classify the chairs in optical images. Song et al.
20
developed a sub-
categorization method based on distance decision rule to categorize different organs in medical
images. In Ref. 21, Ruan et al. employed a more precise measurement of similarity to generate
the weakly supervised clustering scheme for the animal objects in optical images. Based on the
selected data, these subcategory schemes achieve good classification results. However, these
methods cannot be applied to SAR image directly. The main reason is that weak intensity
differences and inhomogeneous regions in SAR images degrade the discrimination ability of
the features to the targets. Thus, the specific subcategorization scheme for SAR images should
be further considered.
In this paper, we present a Bayesian sparse representation (BSR) model for SAR image clas-
sification. It involves the subcategorization of the training samples, the design of the BSR model,
and the classification decision. First, the training samples of each target category are subcate-
gorized into multiple subclasses. The discriminative features are then extracted from each sample
within one subclass. These common features from all samples are gathered to construct a subdic-
tionary. This means that there are N
f
subdictionaries for N
f
different features. After collecting all
N
f
subdictionaries, the sparse reconstructions are performed for all features. A Bayesian frame-
work is employed for such purposes. Finally, a fusion strategy is applied to the residuals to predict
the class label of the test sample. The test results demonstrate the effectiveness of our method.
The main contribution of our work is to integrate the subcategorization scheme into the
Bayesian sparse learning model. We focus on the design of the subcategorization scheme, and
the formulation of the BSR model, and the implementation of the classification decision (i.e.,
residual fusion). By performing sparse reconstructions over multiple subdictionaries with sound
discrimination ability, the presented method can estimate the more accurate class labels for dif-
ferent targets via a fusion strategy. Moreover, by subcategorizing the samples into multiclusters,
our BSR model decreases the intraclass variations of the samples, and thus improve the repre-
sentation ability of the features to different targets. It achieves a higher classification accuracy
than some state-of-the-art methods using a single feature or a synthetic dictionary in the complex
scenes.
Dai et al.: Bayesian sparse representation model for SAR image classification
Journal of Applied Remote Sensing 046517-2 Oct–Dec 2019
•
Vol. 13(4)