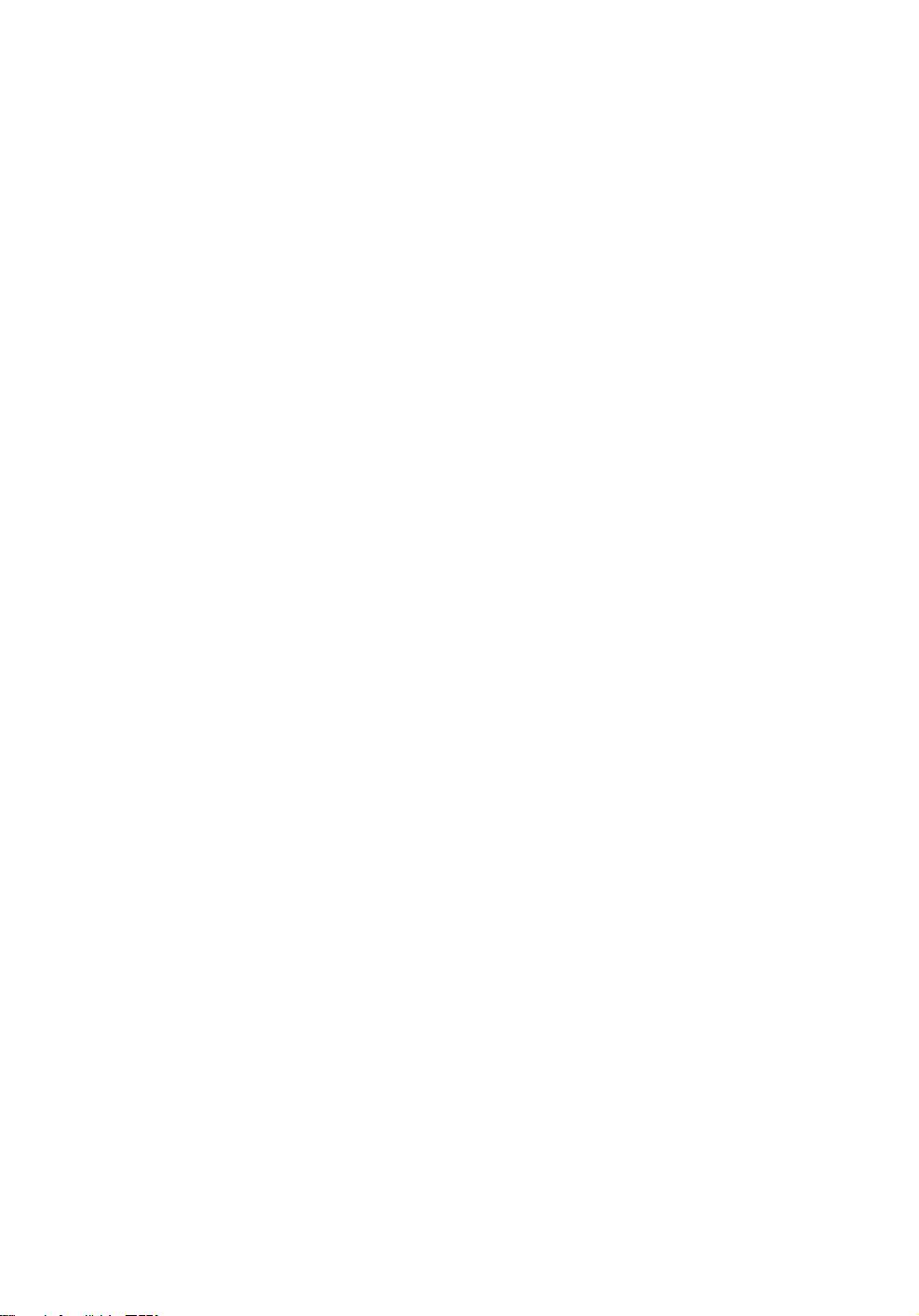
With the advent of cheap storage and fast computers, the amount of engineering
data generated during design and development accumulates beyond the ability of
human beings to shift the data into knowledge or information. Yet, accessing and
distilling the valuable knowledge hidden in this vast amount of data is crucial
(Romanowski and Nagi 2004). Data mining-based approaches in engineering design
have emerged with no end in sight, because they offer many attractive solutions
and methods for difficult information-related engineering problems (Romanowski
and Nagi 2004). Kusiak (2001a) used a classical rough set-based data mining
technique to extract rules for making predictions in the semiconductor industry.
Other approaches to decomposing data sets are discussed in Kusiak (2000, 2001b).
The decomposition is used to enhance the quality of knowledge extracted for large
databases by simplifying the data mining task. Kusiak (2001b) discussed the feature
transformation methods called feature bundling.
Huang (2004) pointed out that the tacit knowledge needs to be externalized as
explicit knowledge and proposed an approach to externalizing the implicit
knowledge to be used as design rule resulted from association rules mining. The
intention is to use such association rules to aid modular selecting in decision-making
of design. Agard and Kusiak (2004b) utilized clustering to identify similar customers
that share the same or highly similar behaviours. Since customers from the same
cluster share similar requirements, it is judicious to propose a specific product family
design for each customer group. However, the clustering is based on the customer
description such as age, income and gender, rather than the specific needs such as
price, security and performance/cost. Romanowski and Nagi (2006) presented a
novel data mining-based methodology for semi-automated formation of a GBOM
(Generic Bill of Materials) from the legacy BOM.
Traditional configuration design cannot differentiate the configuration
objects clearly and effectively, because the complex product features and
performance parameters confuse the customers too much. The relationships between
customer needs (CNs) and functional requirements (FRs) are often not readily
available at the early stage of design. Fortunately, the relationships turn out to be
more specific with the increasing number of purchased products and the FRs are
expressed by the product specifications in the downstream of design. Some kind of
configuration information is usually available by engineering database techniques.
For example, the requirement configuration information is stored within
Customer Relationship Management (CRM), while the engineering configuration
information is often stored within Product Data Management (PDM) or
Configuration Knowledge Base (CKB). But the configuration knowledge is usually
defined beforehand and is too rigid to satisfy the dynamic and changing customer
needs in a timely manner.
Sabin and Weigel (1998) realized that the major challenge in traditional
knowledge-based configuration system was the acquisition of rules or constraints.
Although product configuration is of critical importance, past research only focused
on the establishment of configuration rules between customer requirements and final
configuration alternatives as well as the mapping relation between CNs and FRs in
conceptual design. In fact, a mass of purchased products possess abundant customer
needs information, product specification information and eventual configuration
alternative information. Meanwhile, most new products are an evolution from the
products purchased. Product specifications consist of the product physical structure
Integrating data mining 2791
Downloaded by [Universitat Politècnica de València] at 02:48 28 October 2014