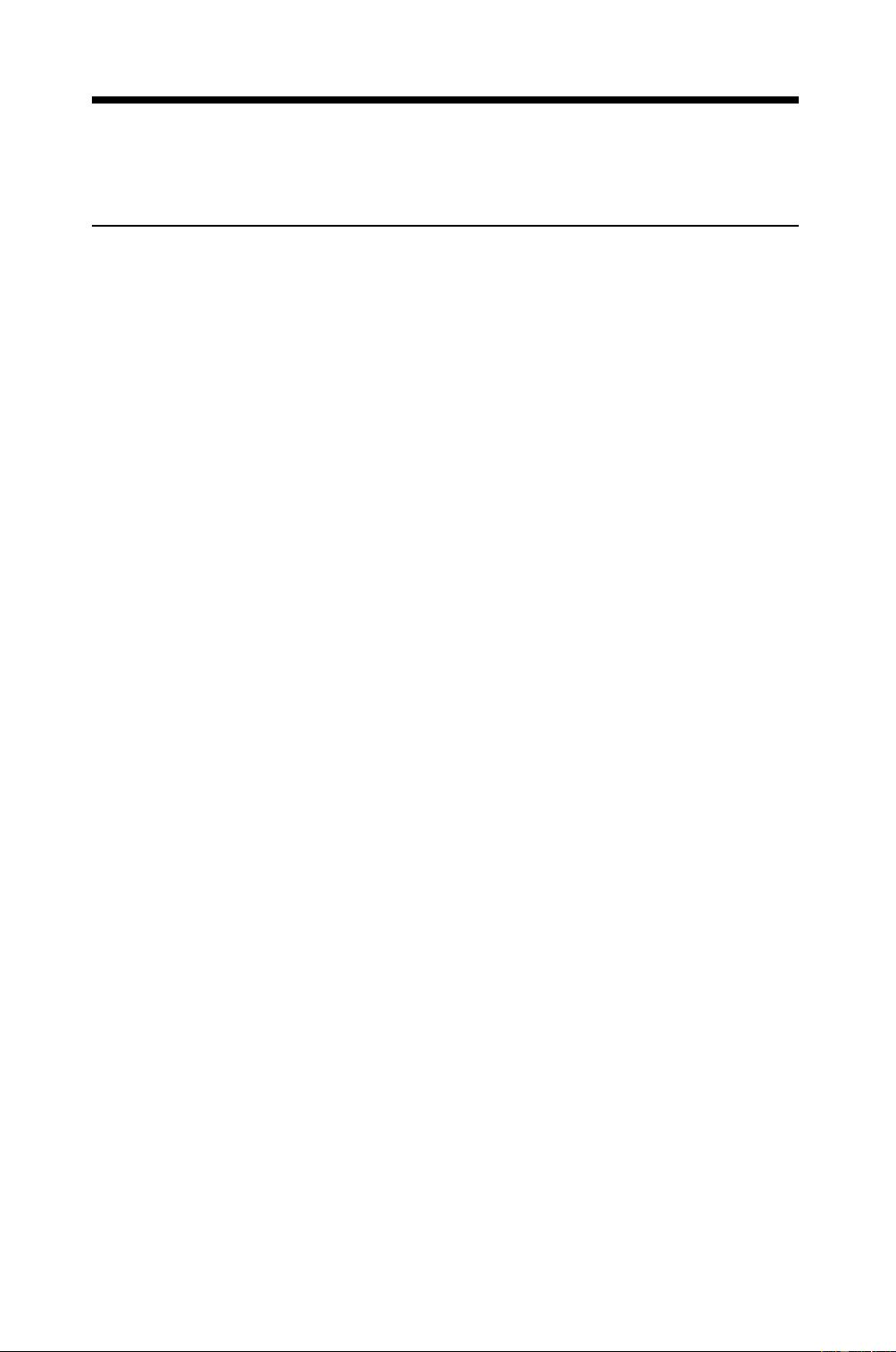
Fast Image Deconvolution
using Hyper-Laplacian Priors
Dilip Krishnan,
Dept. of Computer Science,
Courant Institute,
New York University
dilip@cs.nyu.edu
Rob Fergus,
Dept. of Computer Science,
Courant Institute,
New York University
fergus@cs.nyu.edu
Abstract
The heavy-tailed distribution of gradients in natural scenes have proven effective
priors for a range of problems such as denoising, deblurring and super-resolution.
These distributions are well modeled by a hyper-Laplacian
p(x) ∝ e
−k|x|
α
, typ-
ically with 0.5 ≤ α ≤ 0.8. However, the use of sparse distributions makes the
problem non-convex and impractically slow to solve for multi-megapixel images.
In this paper we describe a deconvolution approach that is several orders of mag-
nitude faster than existing techniques that use hyper-Laplacian priors. We adopt
an alternating minimization scheme where one of the two phases is a non-convex
problem that is separable over pixels. This per-pixel sub-problem may be solved
with a lookup table (LUT). Alternatively, for two specific values of α, 1/2 and 2/3
an analytic solution can be found, by finding the roots of a cubic and quartic poly-
nomial, respectively. Our approach (using either LUTs or analytic formulae) is
able to deconvolve a 1 megapixel image in less than ∼3 seconds, achieving com-
parable quality to existing methods such as iteratively reweighted least squares
(IRLS) that take ∼20 minutes. Furthermore, our method is quite general and can
easily be extended to related image processing problems, beyond the deconvolu-
tion application demonstrated.
1 Introduction
Natural image statistics are a powerful tool in image processing, computer vision and computational
photography. Denoising [14], deblurring [3], transparency separation [11] and super-resolution [20],
are all tasks that are inherently ill-posed. Priors based on natural image statistics can regularize these
problems to yield high-quality results. However, digital cameras now have sensors that record im-
ages with tens of megapixels (MP), e.g. the latest Canon DSLRs have over 20MP. Solving the above
tasks for such images in a reasonable time frame (i.e. a few minutes or less), poses a severe challenge
to existing algorithms. In this paper we focus on one particular problem: non-blind deconvolution,
and propose an algorithm that is practical for very large images while still yielding high quality
results.
Numerous deconvolution approaches exist, varying greatly in their speed and sophistication. Simple
filtering operations are very fast but typically yield poor results. Most of the best-performing ap-
proaches solve globally for the corrected image, encouraging the marginal statistics of a set of filter
outputs to match those of uncorrupted images, which act as a prior to regularize the problem. For
these methods, a trade-off exists between accurately modeling the image statistics and being able to
solve the ensuing optimization problem efficiently. If the marginal distributions are assumed to be
Gaussian, a closed-form solution exists in the frequency domain and FFTs can be used to recover the
image very quickly. However, real-world images typically have marginals that are non-Gaussian, as
shown in Fig. 1, and thus the output is often of mediocre quality. A common approach is to assume
the marginals have a Laplacian distribution. This allows a number of fast ℓ
1
and related TV-norm
methods [17, 22] to be deployed, which give good results in a reasonable time. However, studies
1