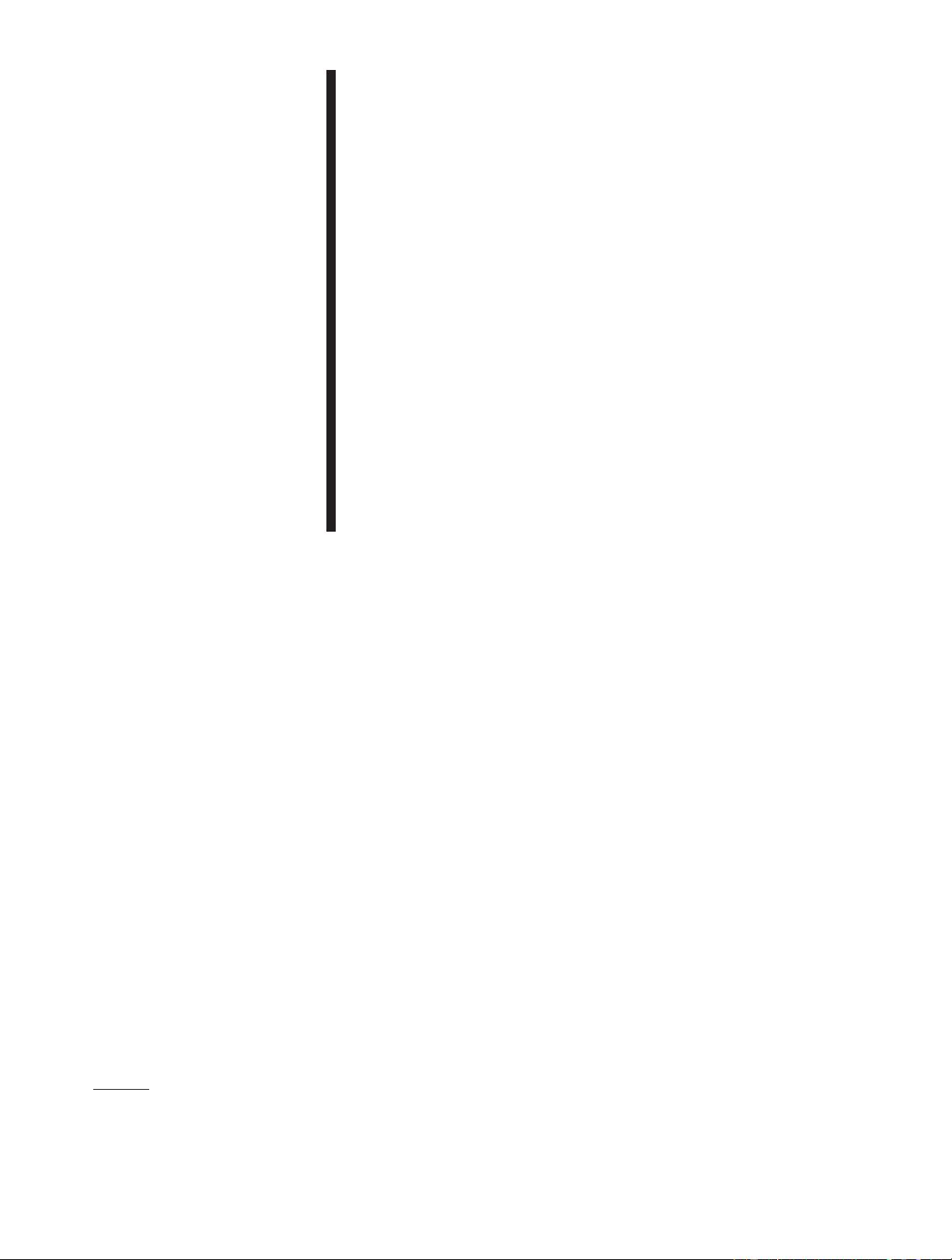
Jianhua Zhang
State Key Laboratory of Alternate Electrical Power
System With Renewable Energy Sources,
North China Electric Power University,
Beijing 102206, China
Jing Xiong
Zhuhai Power Supply Bureau,
Zhuhai 519000, China
Mifeng Ren
1
College of Information Engineering,
Taiyuan University of Technology,
Taiyuan 030024, China
e-mail: renmifeng@126.com
Yuntao Shi
Key Lab of Field Bus and
Automation of Beijing,
North China University of Technology,
Beijing 100144, China
Jinliang Xu
The Beijing Key Laboratory of New
and Renewable Energy,
North China Electric Power University,
Beijing 102206, China
Filter-Based Fault Diagnosis
of Wind Energy Conversion
Systems Subject to
Sensor Faults
The operational reliability of wind energy conversion systems (WECSs) has attracted a
lot of attention recently. This paper is concerned with sensor fault detection (FD) and iso-
lation problems for variable-speed WECSs by using a novel filtering method. A physical
model of WECS with typical sensor faults is first built. Due to the non-Gaussianity of
both wind speed and measurement noises in WECSs, an improved entropy optimization
criterion is then established to design the filter for WECSs. Different from previous
entropy-filtering results, the generalized density evolution equation (GDEE) is adopted to
reveal the relationship among the estimation error, non-Gaussian noises, and the filter
gain. The sensors FD and isolation algorithms are then obtained by evaluating the deci-
sion rule based on the residual signals generated by the filter. Finally, simulation results
show that the sensor faults in WECSs can be detected and isolated effectively by using
the proposed method. [DOI: 10.1115/1.4032827]
Keywords: wind energy conversion systems, fault diagnosis, minimum error entropy,
sensor faults
1 Introduction
Due to the rapid development and strong requirement of wind
turbine technology, fault diagnosis of the WECSs has received a
lot of attention in recent years [1]. The reliability of more than
6000 modern onshore wind turbines and their subassemblies have
been investigated [2].
Because of the direct interaction with environment, which
makes it susceptible to noise, aging, manufacturing defects, etc.,
sensors are vulnerable to faults. Therefore, sensor fault diagnosis
has attracted a lot of attention and some strategies have been pro-
posed [3–13]. Generally speaking, these sensor fault diagnosis
approaches can be mainly classified into two groups: (1) observer
or filter-based method [3] and (2) statistic approach based on the
Bayesian theorem, Monte Carlo approach, likelihood method, and
hypothesis test technique [4].
These observer or filter-based sensor fault diagnosis methodol-
ogies can diagnose sensor faults through the analysis and dis-
posal of the residual generated by observer or filter [5–7]. An
approach for detecting and isolating sensor fault was presented
and applied into aircraft by using an extended Kalman filter [5].
An adaptive sliding mode observer was developed for sensor
fault diagnosis in an industrial gas turbine with parameter uncer-
tainties [6]. The sensor fault of a microelectromechanical system
was diagnosed by an agile and efficient extended Kalman filter
[7]. The observer or filter-based fault diagnosis methods applied
in WECSs proved effective as well [8–13]. Liu et al. [8] and
Wei and Liu [9] utilized mixed H
=H
1
observer to diagnose
power sensor fault and blade root bending moment sensor fault
in various speed wind turbine systems, respectively. Both Odg-
aard and Stoustrup [10] and Chen et al. [11] employed the
unknown input observer for sensor and process FD in WECSs.
In Ref. [12], a robust fuzzy fault-tolerant control algorithm was
used to deal with sensor faults, wind disturbances, and paramet-
ric uncertainties of variable-speed WECSs based on a group of
observers, which supposed that only one sensor failed at each
instant. A bank of observers were applied to detect multiple sen-
sor faults occurred simultaneously in doubly fed induction gener-
ators [13].
The main limitation of the above method is that it needs the
exact system model, however, it is difficult to obtain exact
models due to nonlinearities, uncertainties, and multivariable
coupling existed in practical industrial processes. Hence, the
statistic approach might be an alternative fault diagnosis strat-
egy. If the statistical properties of the noise, together with the
way it affects the plant outputs, are known (or can be reason-
ably approximated or assumed), the FD and isolation problem
can then be formulated in the framework of statistical
decision-making. In Ref. [14], a sensor FD and identification
approach for steam generators in thermal power plants was
presented. Based on the identified model, a data-driven fault
detector was then formulated. Subspace identification techni-
ques have been adopted for sensor FD of wind turbine in Ref.
[15]. Luo et al. [16] extended the data-driven scheme to fault-
tolerant control of WECSs.
The above-mentioned approaches presented for WECSs hardly
obtained satisfied diagnosis results in practical WECSs subject to
non-Gaussian disturbances induced by both wind speed and mea-
surement noises. A few improved filter-based fault diagnosis
scheme has been proved to be an efficient method to solve FD and
isolation problems for nonlinear and non-Gaussian systems
recently. Guo and coworkers [17] and [18] presented filtering
algorithms for stochastic distribution systems based on minimum
1
Corresponding author.
Contributed by the Dynamic Systems Division of ASME for publication in the
J
OURNAL OF DYNAMIC SYSTEMS,MEASUREMENT, AND CONTROL. Manuscript received
April 28, 2015; final manuscript received December 21, 2015; published online April
1, 2016. Assoc. Editor: Ryozo Nagamune.
Journal of Dynamic Systems, Measurement, and Control JUNE 2016, Vol. 138 / 061008-1
Copyright
V
C
2016 by ASME
Downloaded From: http://dynamicsystems.asmedigitalcollection.asme.org/ on 12/24/2016 Terms of Use: http://www.asme.org/about-asme/terms-of-use